What are AI Hallucinations? When AI goes wrong
Liar liar, pants on fire
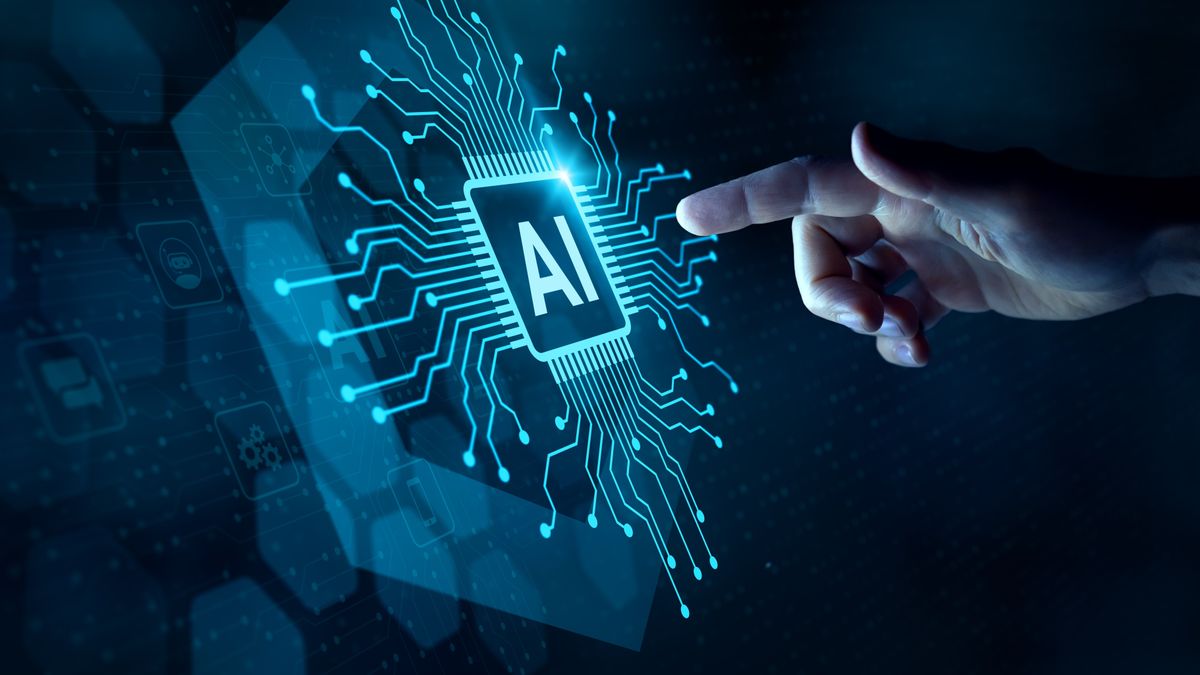
AI Hallucinations are instances when a generative AI tool responds to a query with statements that are factually incorrect, irrelevant, or even entirely fabricated.
For instance, Google’s Bard falsely claimed that the James Webb Space Telescope had captured the very first pictures of a planet outside our solar system. AI Hallucinations proved costly for two New York lawyers who were sanctioned by a judge for citing six fictitious cases in their submissions prepared with the assistance of ChatGPT.
“Even top models still hallucinate around 2.5% of the time,” says Duncan Curtis, SVP of GenAI and AI Product at Sama. “It’s such an issue that Anthropic’s major selling point for a recent Claude update was that its models were now twice as likely to answer questions correctly.”
Curtis explains that 2.5% seems like a relatively small risk, but the numbers quickly add up for popular AI tools like ChatGPT, which by some accounts receives up to 10 million queries per day. If ChatGPT hallucinates at that 2.5% rate, that would be 250,000 hallucinations per day or 1.75 million per week.
And this is not necessarily a steady rate, warns Curtis: “If models’ hallucinations are reinforced as “correct,” then they will perpetuate those mistakes and become less accurate over time.”
Why does AI hallucinate?
In very simple terms, generative AI works by predicting the next most likely word or phrase from what it has seen. But if it doesn’t understand the data it’s being fed, it’ll produce something that might sound reasonable but isn’t factually correct.
Simona Vasytė, CEO at Perfection42 works with visual AI models, and says to generate visuals, AI looks at the surroundings and "guesses" which right pixel to put in place. Sometimes they guess incorrectly, resulting in a hallucination.
Are you a pro? Subscribe to our newsletter
Sign up to the TechRadar Pro newsletter to get all the top news, opinion, features and guidance your business needs to succeed!
“If a large language model (LLM) is trained on vast information found all over the Internet, it can find any kind of information - some factual, some not,” says Vasytė. “Conflicting knowledge might cause variance in the answers it gives, increasing the change of AI hallucinations.”
Curtis says LLMs are not good at generalizing unseen information or self-supervising. He explains the top cause of hallucinations is a lack of sufficient training data and an inadequate model evaluation process. “Flaws in the data, such as mislabeled or underrepresented data, are a major reason why models make false assumptions,” explains Curtis.
For instance, if a model doesn’t have enough information, such as what qualifications someone must meet for a mortgage, it can make a false assumption and approve the wrong person, or not approve a qualified person.
“Without a strong model evaluation process to proactively catch these errors and fine-tune the model with additional training data, hallucinations will happen more frequently in production,” asserts Curtis.
Why is it important to eliminate hallucinations?
As the two New York lawyers found out, AI hallucinations aren’t just an annoyance. When an AI spews wrong information, especially in information-critical areas like law and finance, it can lead to costly mistakes. This is why experts believe it’s imperative to eliminate hallucinations in order to maintain confidence in AI systems and ensure they deliver reliable results.
“As long as AI hallucinations exist, we can't fully trust LLM-generated information. At the moment, it's important to limit AI hallucinations to a minimum, because a lot of people do not fact-check the content they stumble upon,” says Vasytė.
Olga Beregovaya, VP of AI and Machine Translation at Smartling says hallucinations will only create as many liability issues as the content that the model generates or translates.
Explaining the concept of "responsible AI,” she says when selecting what content type a generative AI application is used for, an organization or an individual needs to understand the legal implications of factual inaccuracies or generated text irrelevant to the purpose.
“The general rule of thumb is to use AI for any "informational content" where false fluency and inaccurate information will not make a human make a potentially detrimental decision,” says Beregovaya. She suggests legal contracts, litigation case conclusions, or medical advice should go through a human validation step.
Air Canada is one of the companies that’s already been bitten by hallucinations. Its chatbot gave someone the wrong refund policy, the customer believed the chatbot, and then Air Canada refused to honor it until the courts ruled in the customer’s favor.
Curtis believes the Air Canada lawsuit sets a serious precedent: if companies now have to honor hallucinated policies, that poses a major financial and regulatory risk. “It would not be a huge surprise if a new industry pops up to insure AI models and protect companies from these consequences,” says Curtis.
Hallucination-free AI
Experts say that although eliminating AI hallucinations is a tall order, reducing them is certainly doable. And it all begins with the datasets the models are trained on.
Vasytė asserts high-quality, factual datasets will result in fewer hallucinations. She says companies that are willing to invest in their own AI models will result in solutions with the least AI hallucinations. “Thus, our suggestion would be to train LLMs exclusively on your data, resulting in high-precision, safe, secure, and trustworthy models,” suggests Vasytė.
Curtis says although many of the root causes of hallucinations seem like they can be solved by just having a big enough dataset, it’s impractical to have a dataset that big. Instead, he suggests companies should use a representative dataset that’s been carefully annotated and labeled.
“When paired with reinforcement, guardrails, and ongoing evaluations of model performance, representative data can help mitigate the risk of hallucination,” says Curtis.
Experts also point to retrieval augmented generation (RAG) for addressing the hallucination problem.
Instead of using everything it was trained on, RAG gives generative AI tools a mechanism to filter down to only relevant data to generate a response. It is believed outputs from RAG-based generative AI tools are a lot more accurate and trustworthy. Here again, though companies must ensure the underlying data is properly sourced and vetted.
Beregovaya says the human-in-the-loop fact-checking approach is the safest way to ensure that hallucinations are caught and corrected. This, however, she says, happens after the model has already responded.
Tossing the ball to the other side of the fence, she says “The best, albeit not entirely bullet-proof, way of preventing or reducing hallucinations is to be as specific as possible in your prompt, guiding the model towards providing a very pointed response and limiting the corridor of possible interpretations.”
- We've rounded up the best Large Language Models (LLMs).
With almost two decades of writing and reporting on Linux, Mayank Sharma would like everyone to think he’s TechRadar Pro’s expert on the topic. Of course, he’s just as interested in other computing topics, particularly cybersecurity, cloud, containers, and coding.
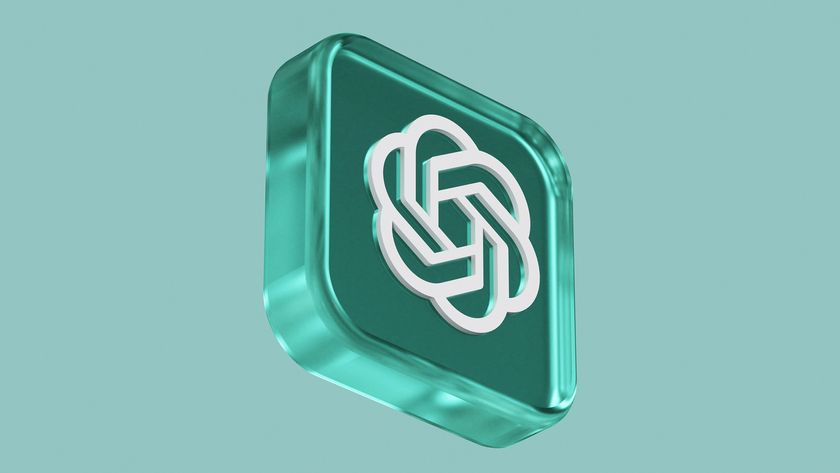
ChatGPT 4o’s image generation is now free for everyone – 3 ways to use the new AI tool without following the Studio Ghibli herd
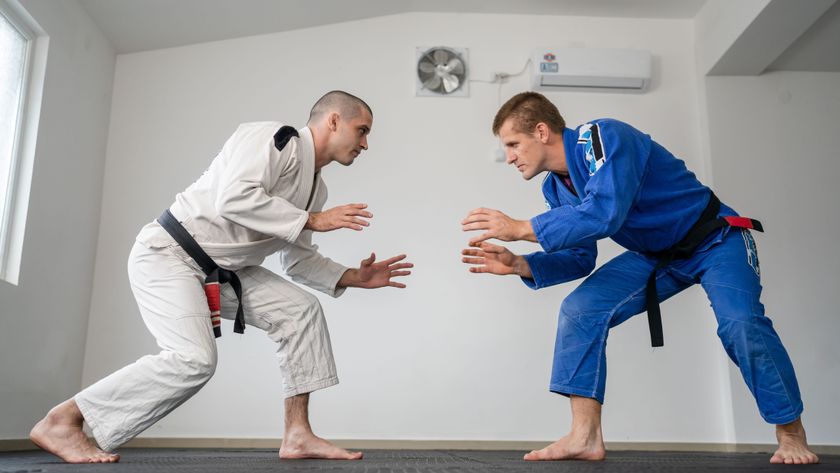
I’ve taught jiu-jitsu for over 5 years, and I’ve only just started using AI to come up with lesson plans – I don’t know why I didn’t think of this sooner!