Daedalean: the AI copilot looking for permission to fly
AI is becoming the norm on the ground, but what about the skies?
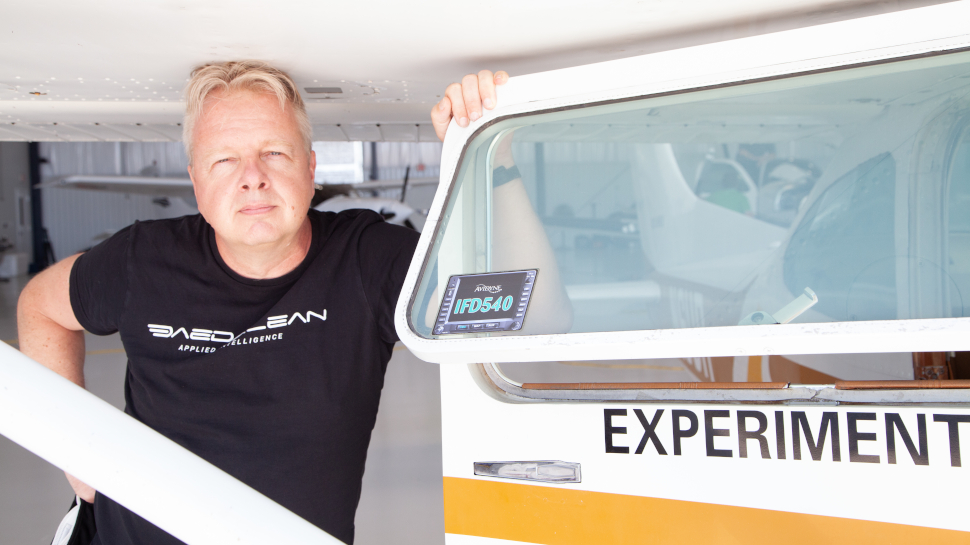
AI is starting to seep into virtually every aspect of our lives, automating all the tasks we don’t want to do. When it comes to transport, driverless cars have been around for a while now and continue to make progress; but what about going one step further - helping us to fly? One company - Daedalean - is on a mission to do just that.
In February, Daedalean, in partnership with Intel, published one in a series of upcoming white papers detailing its certifiable AI system, and how the technology could progress to lead to fully autonomous flight across the Aerospace and Defense (A&D) industry in future.
But focusing on its current technology, TechRadar Pro spoke to the company’s founder, CEO & CTO Dr. Luuk van Dijk, to find out more about its all-seeing AI and its goal towards becoming the first certified for a flight control application in civil aviation.
Planespotting
Daedalean’s inaugural AI product is called PilotEye, and is what Dr. van Dijk calls a “visual situational awareness suite”, making use of hardware manufactured by avionics firm Avidyne.
It is currently able to identify airborne traffic, but the full version, once released - currently known as the VXS (“visual everything suite”) will be able to detect all kinds of airborne hazards - “including birds and drones” - and provide “GNSS-independent navigation and positioning, and landing guidance for helicopters, fixed-wing aircraft, and eVTOL.” As Dr. van Dijk describes it, “VXS is an AI copilot working as a pilot assistant.”
The VXS comes equipped with several multidirectional cameras and a unit that computes the information using Daedalean’s algorithms:
“The software is based on computer vision and machine learning (“AI”) and works on board aircraft with no connection to any ground infrastructure. The algorithms explore the video feed from each camera, [and] recognize and interpret what they are “seeing”.”
Sign up to the TechRadar Pro newsletter to get all the top news, opinion, features and guidance your business needs to succeed!
This includes: “the landscape below to compare it with maps and provide pilot coordinates and altitude… ; the traffic around – what intruders are flying where and what is the distance to them; the runways or landing spots below, and the directions for the safe landing.”
Currently, the VXS can only operate effectively under optimal visual conditions, with Dr. van Dijk conceding that “while it is capable of working in moderately bad weather or reduced daylight, it is still not the omnipotent product.”
However, Dr. van Dijk claims that future developments will “make the suite work in any conditions, including the total absence of visibility.” This will be achieved by “adding night vision sensors, radars, and other sources of information.”
By parsing the input from the VXS cameras and other forthcoming sensors, the AI creates a “situational map of the environment”. This is what sets it apart from other avionic systems. The neural network analyzes these inputs and “gives answers to a question it is specified for: for example, is there an intruder on the image, like an aircraft or drone; or is there a runway below suitable for landing?”
Its job is to “process complicated information of high uncertainty, like an image with a landscape, objects on it, sky, clouds, and objects in the sky – and define what is there, which objects, where, on which distances, etc.”
But what exactly is the advantage of having an AI copilot over a human? Dr. van Dijk explains:
“Adding it to the cockpit will substantially add to flight safety: its tested performance results show that it sees much better and further than human vision.” Unlike a human, it is able to scan the entire sky at once, and is able to “recognize a Cessna at a distance of 3 nautical miles when it’s just a dot in the sky.”
Dr. van Dijk also makes the point that is usually made in favor of automated systems in general: “unlike a human, it is never tired or distracted. Its purpose is just to let a pilot concentrate on their mission.”
Training
As with all AI systems, the algorithm had to undergo training. Dr. van Dijk explains the process by which the complex statistical model employed by the AI was developed:
“It was created by… analyzing millions of similar images annotated by humans. Humans have a seemingly magical ability to know the answer to the question “is there an aircraft in this image” by looking at it – but a potent statistical model can extract statistical patterns from their decisions, finding millions of parameters distinguishing images for which a human answered “yes” from the ones with “no”. This process is called machine learning.”
Dr. van Dijk detailed the many layers involved in this training process, to ensure the AI is adequately equipped to identify traffic with a high degree of correctness.
The first step as mentioned above - analyzing millions of images - was paid special attention to, with two teams - one in Riga and one in Zurich - of “specially trained data annotators” being assigned to the task. Dr. van Dijk remarks that Daedalean “invested a lot in this because the process of how they work with data is also subject to certification requirements.”
These annotated images are then used as the training data that is fed into the neural network which “analyzes each pixel on them and finds statistical dependencies between the state of each of those pixels”, to determine whether or not an aircraft is present and its exact location within the image.
“There are millions (again, literally) of the parameters it counts in its statistical equations; that’s why no human is able to monitor it and influence what it does. This is the role of another special computer program (training algorithm). It fiddles with the parameters asking the neural network again and again and comparing its answers to the answers done by humans (in the form of those annotated images). Until the NN answers become reliably similar to the known answers.”
The next phase is testing, where the newly-trained algorithm is fed yet more images it has never seen before - again annotated by humans - and tasked once more with making identification decisions.
Once it passes this stage, it gets installed onto a “data collection aircraft or drones (when we are testing lighter versions of hardware).”
“Often we have two aircraft flying – one with VXS onboard and the second one to act as a target. They play different scenarios, encountering from different directions, at different altitudes, directions, velocities. The system records everything that its cameras see during the flight.”
From here, the AI is assessed after its flight: “we have special algorithms AND humans analyzing it second by second and image by image. We analyze where the neural network was mistaken and why and use this knowledge for the next cycle.”
And so, “after many cycles of lab testing, improving, real flight testing, improving again – the whole application gets frozen and released.” What or when that release might be depends on being certified by the appropriate authorities.
Cleared for takeoff
Daedalean is looking to get its AI certified with both the FAA (Federal Aviation Administration) and EASA (European Union Aviation Safety Agency): “We are working with the European and American regulators simultaneously to get a certificate covering a selection of aircraft models in 2023.” If it achieves this, then this will be a world first for AI.
But Daedalean is doing more than trying to get its own product off the ground. It is an active participant in conducting research and reports on the use of AI and ML in aviation, working with both the aforementioned authorities closely to ensure AI is applied safely to the industry.
“EASA and Daedalean collaborated and published two joint reports on Concepts of Design Assurance for Neural Networks (CoDANN) (2020, 2021). The reports discuss how classical software design assurance can be adapted for ML in safety-critical settings. The results of this research partly led to EASA's first guidance for Level I AI/ML in aviation.”
And then, in 2021, “the FAA studied, in collaboration with Daedalean, the applicability of the CoDANN findings to a real application. This project resulted in a report published by the FAA in 2022.”
Although there is a lot to go through to become certified, Dr. van Dijk is optimistic about the future, confident that growth will come:
“Based on the demand we are currently seeing for our Eval Kit (the demonstrator we offer to selected customers possessing permission to install experimental equipment), we expect a hundred annual installations annually in the first and second year (this is a significant number for the General Aviation industry), and an exponential growth after that, since the air taxis will be getting their operation permissions and arrive to the market at the scale of thousands.”
- For AI you can get your hands on, why not try one of the best AI writers?
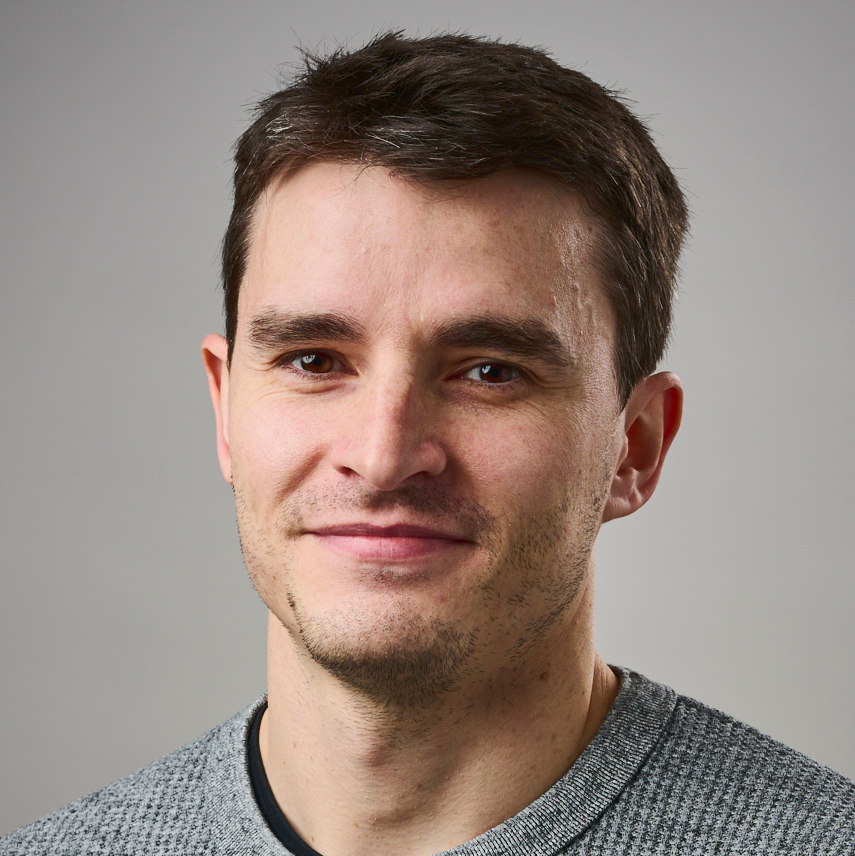
Lewis Maddison is a Reviews Writer for TechRadar. He previously worked as a Staff Writer for our business section, TechRadar Pro, where he gained experience with productivity-enhancing hardware, ranging from keyboards to standing desks. His area of expertise lies in computer peripherals and audio hardware, having spent over a decade exploring the murky depths of both PC building and music production. He also revels in picking up on the finest details and niggles that ultimately make a big difference to the user experience.