Why this chess grandmaster left Google behind
Tal Shaked left Google after 17 years to bring machine learning to the masses
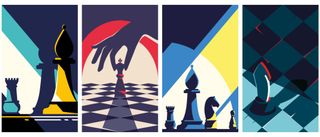
The chess career of Tal Shaked began by chance at the age of seven, when an inconvenient carpool arrangement led him to join his local club. But his natural talent quickly became clear.
After collecting an embarrassment of regional trophies in his home state of Arizona, Shaked was crowned national youth champion in 1997, propelling him into the world of professional chess alongside the likes of Gary Kasparov, Vladimir Kramnik and other grandees.
At the age of nineteen, victory at the World Junior Championships earned him the title of chess grandmaster, one of the two most sought-after accolades in the sport, second only to world champion.
However, the problem with professional chess is that making a handsome living is far from trivial, irrespective of grandmaster status. Only a small minority at the pinnacle of the game are able to earn big, Shaked told TechRadar Pro. The rest have to supplement with tutoring and other side hustles.
The other problem is that players typically reach their peak in their mid-30s, after which they are swiftly displaced by new talent rising through the ranks.
To put his parents’ minds at ease (they worried he might turn into a “chess bum”, with few long-term prospects), Shaked retired from the professional game at 20 and returned to school to study computer science. As it turned out, he was rather good at that too.
Google comes knocking
When Shaked arrived at Google in 2004, the company had just 3,000 employees and looked nothing like the sprawling megacorporation it is today. He was brought on as a junior engineer to work on Google Search.
Are you a pro? Subscribe to our newsletter
Sign up to the TechRadar Pro newsletter to get all the top news, opinion, features and guidance your business needs to succeed!
At the time, Google’s search rankings were not powered by any form of intelligence. Instead, a dedicated team of engineers was tasked with managing a complex rule-based system designed to serve up the best and most relevant results to users.
Although the system was sophisticated, it was also overly-intricate and therefore intractable, and Shaked thought he had a better way. Alongside Yoram Singer, now a world-renowned expert in the field, he developed a machine learning-based rankings system, called Rankboost.
“The ML system worked really well, outperforming the other system in all the metrics we could measure. But there were problems when it came to the metrics we couldn’t really measure, [where an element of subjectivity was involved],” he explained.
It would take a number of years before the company found an effective way to deploy the search ranking system Shaked had developed. But over the course of that time, he moved between various internal departments – from Search to Ads to YouTube - deploying ML to help the company optimize its various revenue streams.
Thanks at least in part to the work of Shaked, Google is today among the handful of companies operating at the bleeding edge of machine and deep learning, which underpins practically all of its products in some way or form.
But despite the significant impact of his work at Google, Shaked had come to learn an uncomfortable truth; the expertise he and his peers had accrued over the years was to some extent locked inside the company.
“Having been at Google for roughly 17 years, it was time to consider new opportunities,” he said. “It was an interesting challenge, to create value in the outside world by leveraging what I had learned.”
ML beyond Google
Shaked already had a “flavor of what ML looked like for the rest of the world” from a brief interval during which he worked for ride-sharing company Lyft.
What he found there surprised him; the resources available at Google meant that each engineer could effectively act autonomously, whereas other companies require whole teams to execute each step in the ML development process, from data processing to modelling to productization.
Shaked told us this creates “unnecessary back and forth” that puts a significant tax on agility, apparently a “common issue” for regular organizations.
When he left Google for a second time to join cloud data platform Snowflake, roughly nine months ago, his ambition was to “enable the rest of the world to build ML-powered products”. It wasn’t necessarily about replicating what Google is doing in another setting, but rather helping other companies more effectively reap the benefits of ML.
Shaked had been tapped up by Grzegorz Czajkowski, SVP at Snowflake, with whom he had worked at Google on a number of projects. Out of respect for his previous employer, Czajkowski imposed a one-year moratorium on poaching talent from Google, but made a move for Shaked shortly after. And since then, yet more Googlers have followed.
This kind of mass migration is a relatively common phenomenon, Shaked explained. After all, it’s “tough to go to a new place where you don’t know anyone”.
“I actually think it’s really good for the industry; a lot of these tech-first companies have learned a lot and hogged a lot of the talent, but there is so much innovation that can happen when these people apply their learnings in a different way,” he said.
“It’s a good thing when you get these talent migrations, because there is a whole set of problems that Snowflake and other companies face.”
Democratizing machine learning
Although Shaked is involved in all manner of ML projects at Snowflake, both internal and customer-facing, one of the primary factors in his decision to join was the development of the Snowflake Marketplace.
The ambition behind the marketplace project is to help democratize access to ML resources, by making it simple for customers to purchase large-scale datasets from trusted providers for use in the training of their own models.
And should training an ML model fall outside their area of expertise, the marketplace can also facilitate the purchase of ready-made apps and models submitted by members of the customer and partner network.
Separately, Snowflake is investing heavily in data clean room technology, which allows multiple parties to collaborate on shared pools of data without exposing sensitive business information, unlocking various new opportunities from an ML perspective by improving the quality of data fed into models.
"Our objective is to make it as easy as possible for customers to leverage advanced ML models without having to build from scratch, because that requires a huge amount of expertise," said Shaked.
"Through projects like Snowflake Marketplace, we want to give customers a way to run these kinds of models against their data, both at scale and in a secure way."
Asked whether he believes companies like Google have an unassailable lead in the ML space, Shaked explained that we were examining the situation from the wrong angle. In reality, Google is operating on a completely different plane.
“When [a company] goes from no machine learning to some, the revenue gains can be enormous, in the region of 10-100%,” he explained.
“At Google, they’re now trying to squeeze out 1% here and another there, which accounts for billions of dollars at that scale. But the rest of the world doesn’t need the state-of-the-art models Google has, they need something that will work reasonably well.”
It may even be the case that the system at Google is too complex for Google itself. Once a system has been optimized to a certain degree, it becomes inflexible and increasingly difficult to experiment on top of, Shaked told us.
“This shouldn’t be seen as a competition; everyone is building different products for different users. There is a ton of value to be had and we want to make it as easy as possible for companies to grab that value. It’s about helping more people solve more interesting business problems.”
Joel Khalili is the News and Features Editor at TechRadar Pro, covering cybersecurity, data privacy, cloud, AI, blockchain, internet infrastructure, 5G, data storage and computing. He's responsible for curating our news content, as well as commissioning and producing features on the technologies that are transforming the way the world does business.