AI, ethics and data bias - getting it right
Ensuring fairness, transparency and safety when deploying AI
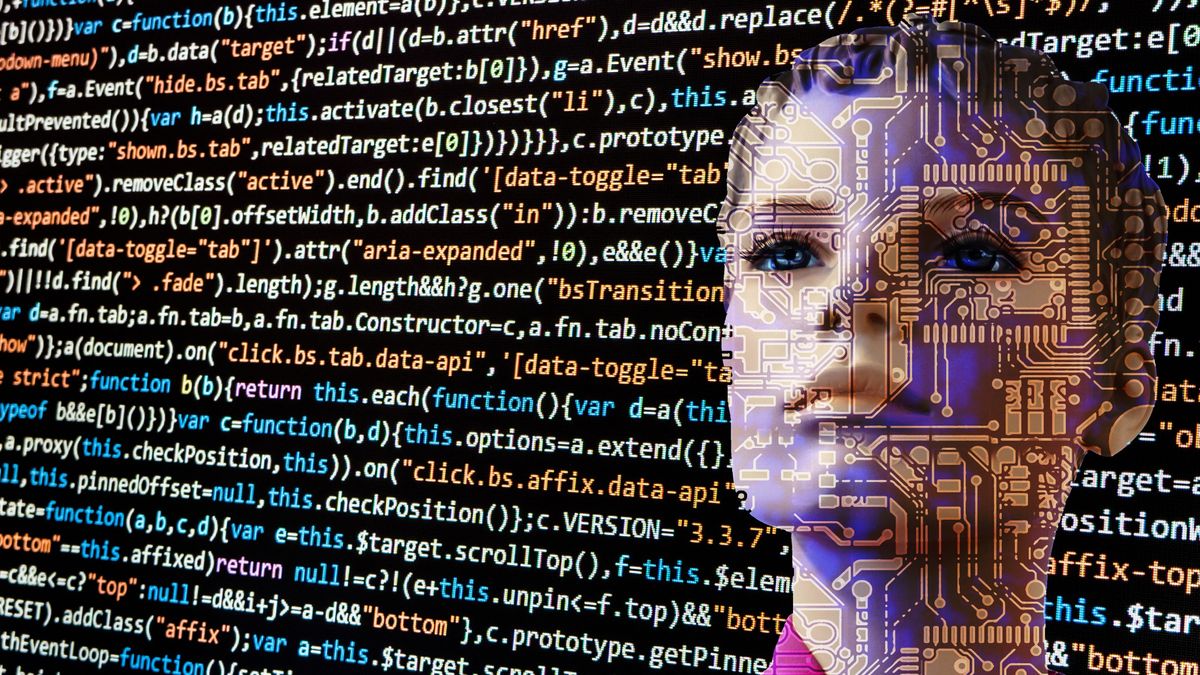
As executives continue to ask how to implement and get value from artificial intelligence (AI), it’s no surprise that AI is top of the C-suite agenda. But with this comes a renewed focus on how we can ensure AI technologies are being created ethically, fairly and without bias. At the highest level we want to be able to answer the question: “How do we get the balance right between those benefits and the risks that go along with them?”
The benefits of AI are clear; we can make harder things become easier, we can automate the mundane jobs, we can support humans to be more creative, we can create new jobs and we can automate dangerous tasks. That said, the concerns being raised are justifiable and I am seeing data bias sat at the centre of these conversations. So how can companies deploy AI in ways that ensure fairness, transparency, and safety?
- AI and ethics: looking inside the black box
- All eyes on AI!
- IBM is opening up the secrets of AI
Understanding data bias
We find it helpful to think about data bias in three levels, the first being bias itself. The first question we need to ask ourselves when thinking about AI is whether the data set reflects the population that you’re trying to model.
For example, there have been various controversies around facial-recognition software not working as well for women or for people of colour, because it’s been trained on a biased data set which has too many white males in it. Or we risk building a system that – because it draws on historical data that reflect historical human biases – doesn’t build in a desired change such as prioritising underrepresented groups in job applications, or moving to a fairer system for parole or stop and search decisions.
You then get into fairness, which is a second level. At this point we need to think about the fact that yes, the data set we’re drawing on to build this model may accurately reflect history, but what if that history was by its nature unfair? So if the data set accurately reflects a historical reality of a population, are the decisions that we make on top of that fair?
Then the final consideration when thinking about ethics and data bias is whether the data sets and models that could be built and deployed could be used for unethical means.
Deploying AI effectively
To make AI work for a diverse range of consumers and businesses, I think executive attention towards these concerns can help create a world in which AI benefits us with minimal risks. Ultimately, it is humans who create the technology, meaning the responsibility lies with us when building AI programmes.
Are you a pro? Subscribe to our newsletter
Sign up to the TechRadar Pro newsletter to get all the top news, opinion, features and guidance your business needs to succeed!
As a leader, thinking about how to manage the risks associated with AI and dedicating a bit of head space to really understand it is an important first step. Then, you need to bring in someone who really grasps the topic – someone whose full-time job is working on the project, who can ask the right questions and has the space to make this their focus.
In my view, we risk not innovating in this space and there is a huge danger of not embracing these techniques and technologies. There is a relationship between risk and innovation that is really important and a partnership between ethics and innovation. We need an ethical framework and an ethical set of practices that enable innovation. If this relationship works, there should be a positive cycle which allows innovation to progress and simultaneously allows for updates to be incorporated into the ethical framework. This is a necessity as we continue to evolve our understanding of AI technology
Chris Wigley, Partner, QuantumBlack a McKinsey Company
Chris Wigley is a Partner at QuantumBlack a McKinsey Company. CEO at Genomics England since October 2019, and currently interim SRO for Data at NHSX supporting the tech response.
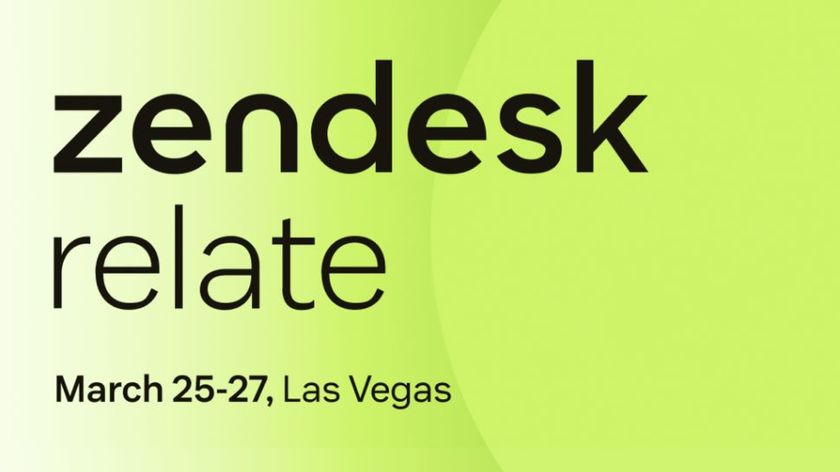
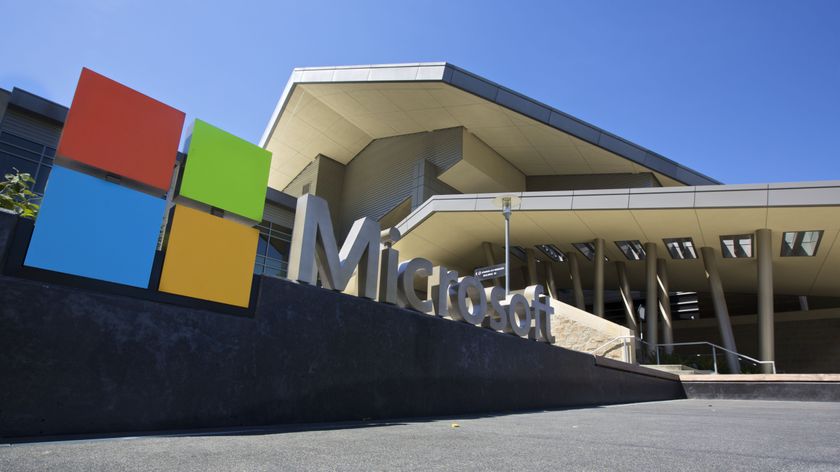
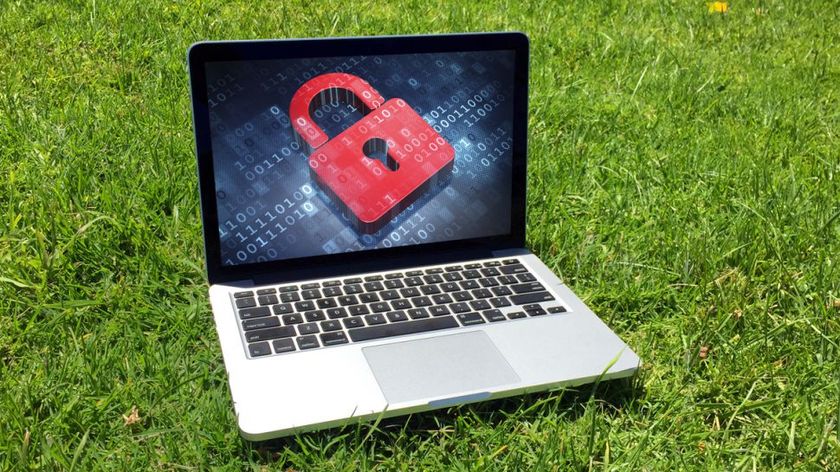
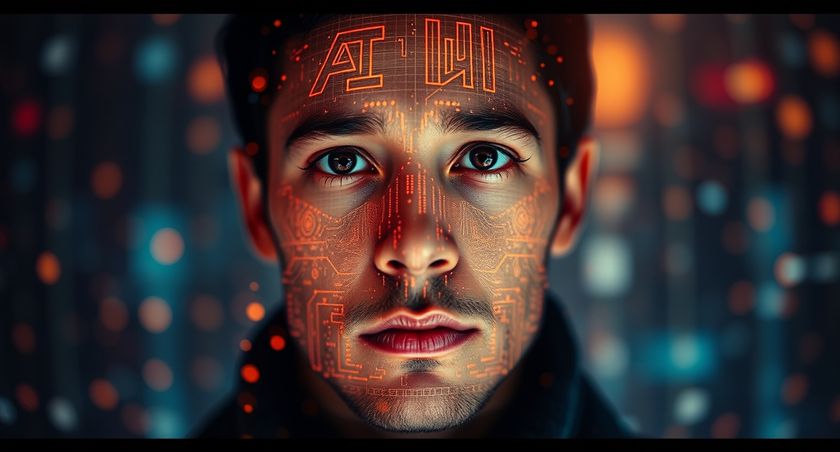
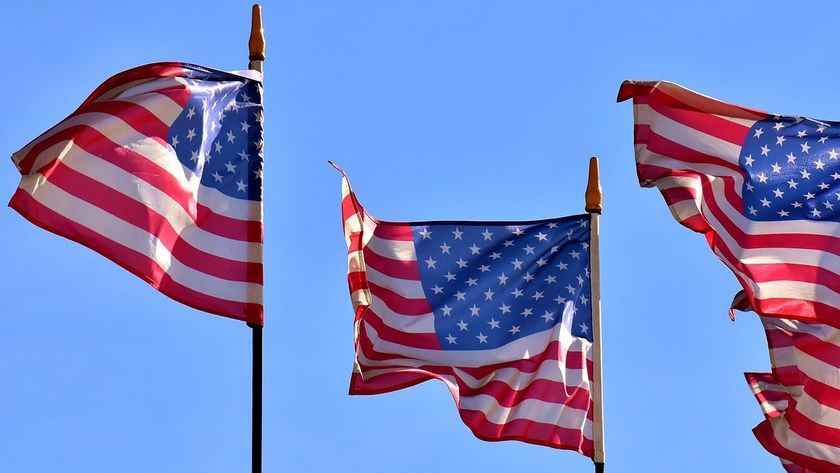
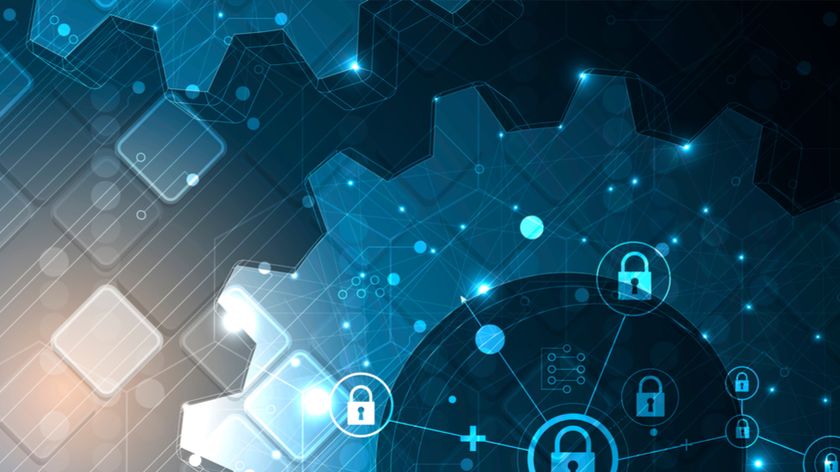
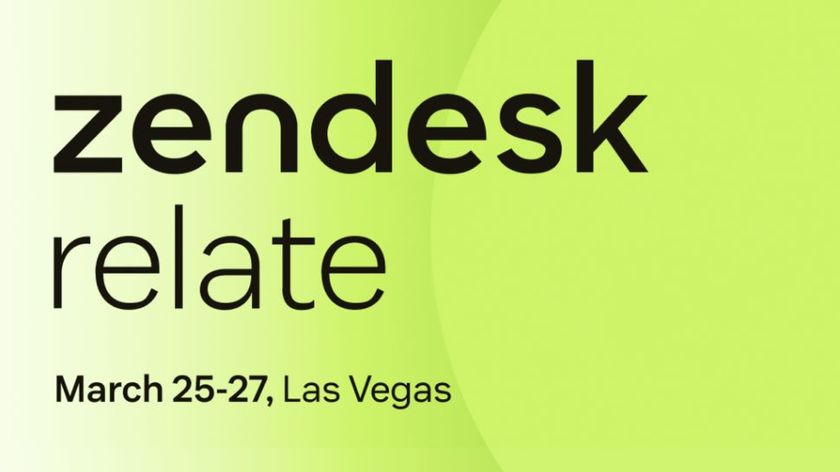
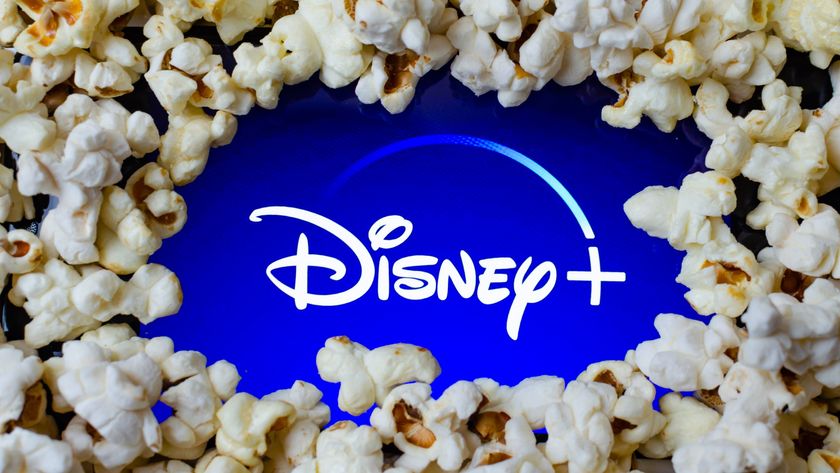
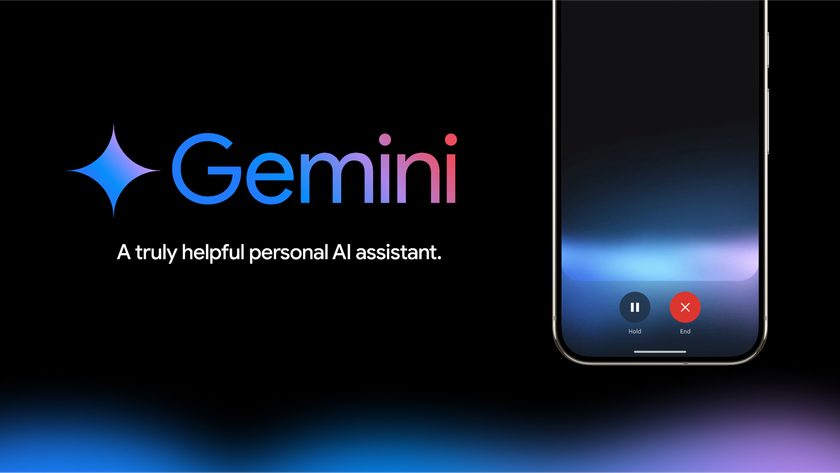
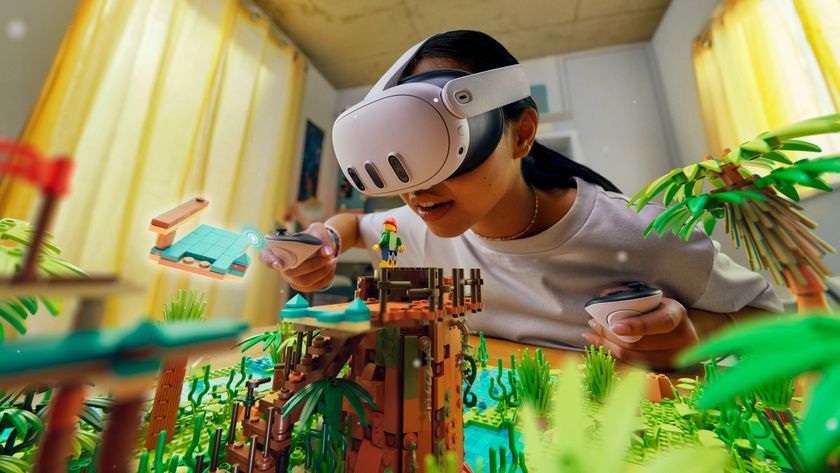
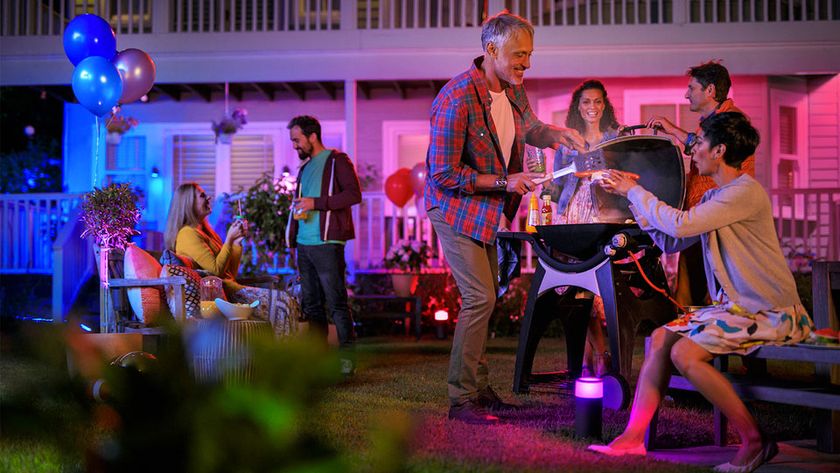
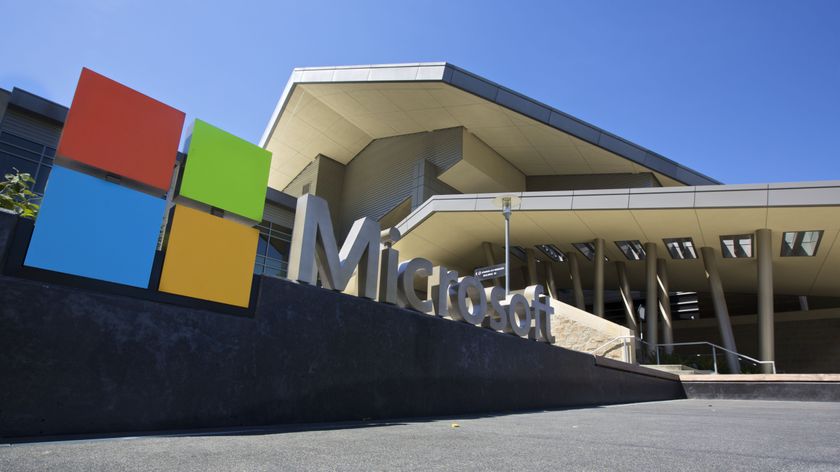