Combating deepfakes with voice biometric technology
How voice biometrics can help combat the influx of deepfakes
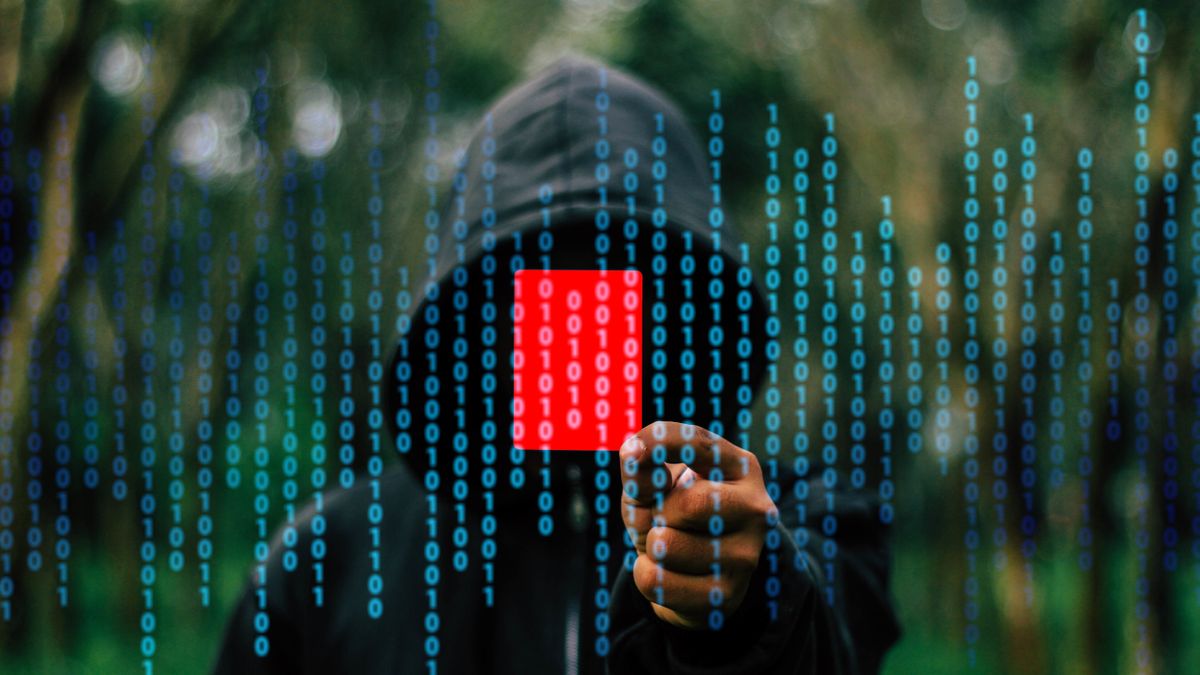
With every advance in technology there seems to be a corresponding advance in the exploitation of that technology for nefarious means. This is especially true within financial services, where the methods of how we interact with our banks have produced a completely new form of “bank robber”.
When transacting consisted solely of visiting the bank branch, the threat of financial loss consisted mainly of the armed robber. However, the advent of the Internet heralded the introduction of web-based banking, a time-saving and technological boon to banks and customers alike. It also introduced a new breed of bank robber in the form of programmers and hackers. The new robbery techniques were not based on guns, but rather social engineering techniques such as phishing, as well as far more advanced techniques such as Man-in-the-Middle and Man-in-the-Browser malware.
Even ATMs, which have become cash dispensing computers, have been targeted by malware attacks. Smartphone apps too, have not been immune from malware aimed at their respective operating systems. Our efforts to thwart these attacks often rely on technology too, such as the use of 2-factor authentication using SMS-based authorisation codes. No surprise then, that these techniques have also been attacked through techniques such as SIM Swap attacks and even the hacking of the global telecommunications network SS7.
- How are consumers protecting themselves against online fraud?
- Reclaiming our digital identity
- Pointing to the future: the next step in fraud prevention
Deepfakes
There is a now a new technology known colloquially as Deepfake that, whilst having its origins far removed from financial services, we believe will have the capability to be used as a new and powerful fraud vector.
Deepfake is the use of Machine Learning to create audio/visual impersonations of real people. It uses a technique known as a Generative Adversarial Network (GAN) which can generate new data from existing data sets. This includes images and audio, so existing video/audio of someone speaking, for instance, can be used to generate new synthetic video/audio of that person, based on what the algorithm learnt from the real video/audio. Whilst initially used to transpose celebrities into pornographic films, the nefarious possibilities of advanced Deepfakes range from weaponizing fake news, i.e. now, we can see the actual target telling us the fake news personally, to election manipulation, misinformation warfare and to a whole new way of digitally perpetrating fraud.
The decline of print media in favour of receiving our news digitally is not only convenient but has introduced far richer content in the form of audio and video. There are virtually unlimited sites we can visit to get news and content. If we see a video clip of a person, unknown or not, delivering a message, we have no reason to suspect that video to be fake. This provides a ready-made forum for those seeking to spread fake news though advanced Deepfakes.
Potential impact on financial services
So why could Deepfake also affect financial services? Just as news is increasingly delivered digitally, so are banking services. Unified Communications and Omni-Channel strategies mean banks communicate with their customers using browser-based video/audio for instance. This could be with a human agent, but in the future also Artificial Intelligence (AI) based agents.
Are you a pro? Subscribe to our newsletter
Sign up to the TechRadar Pro newsletter to get all the top news, opinion, features and guidance your business needs to succeed!
It is not too hard to imagine, therefore, a video/audio conversation between a high net-worth client and their private banker. If the client looks and sounds like him/herself, and of course can provide the answers to any security questions (as they invariably would), why would the banker not acquiesce to any instructions the client gives?
Could the same not also apply on a far greater scale with banks using facial-recognition technology to authenticate customers on web sites and mobile apps? This could involve self-service, interaction with a human agent or with an AI chatbot. If the face matches, and remembering that Deepfakes are not static, they display liveness, then the fraudulent transactions will be executed. These are but two examples involving customer interactions. Inter-bank communications and instructions could be similarly compromised, no doubt in ways the author has not even considered. Being readily identifiable to a colleague or external worker could become the key to exploitation of Deepfake technology. Nobody wants to challenge the identity of a known person who looks and sounds perfectly normal.
Detecting a Deepfake
So how are we expected to detect that what looks real to our eyes and sounds genuine to our ears is actually fake? The answer lies in the audio within a Deepfake and the use of advanced voice biometric techniques. Regardless of how genuine and “human” a Deepfake may look and sound, it is synthetically generated. Even Deepfake videos invariably include an audio component, and it is this audio that is the key to their detection.
Advanced voice biometric algorithms include techniques to detect both recordings, known as replay or presentation attacks, as well as synthetically generated audio. Regardless of how “human” a voice may sound to the human ear, it is not what it sounds like that is important to synthesis detection engines. Their interpretation of whether audio is spoken by a human is very different from ours.
Voice biometrics have always been the strongest and most accurate way to authenticate or identify the actual identity of a human. The ability of the most advanced voice biometric engines to simultaneously identify the distinction between a human and a synthetically generated “human” may prove invaluable if indeed we do see the rise of the Deepfakes.
John Petersen, COO for ValidSoft
- We've also highlighted the best VPN
John Petersen is COO for ValidSoft and comes from a background of Information Technology consulting within the Financial Services industry, both in Australia and the UK. He has over 16 years of working experience.
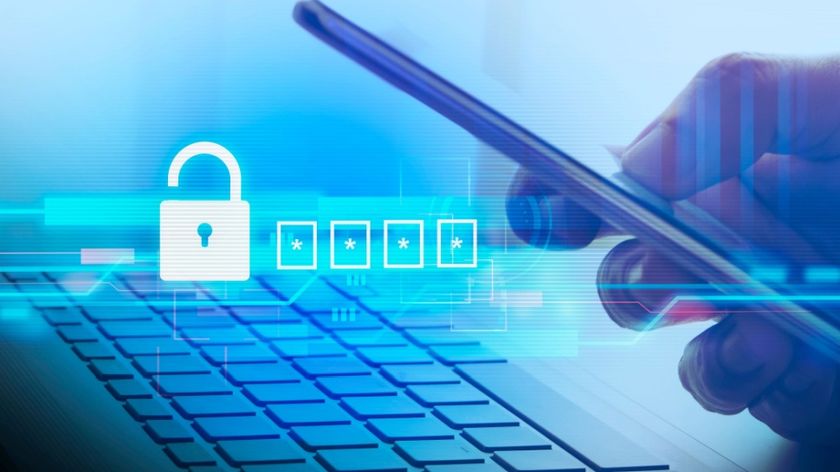
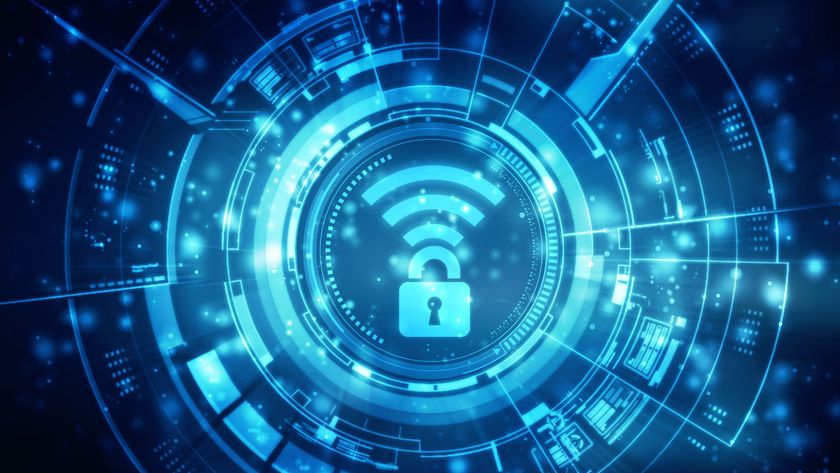
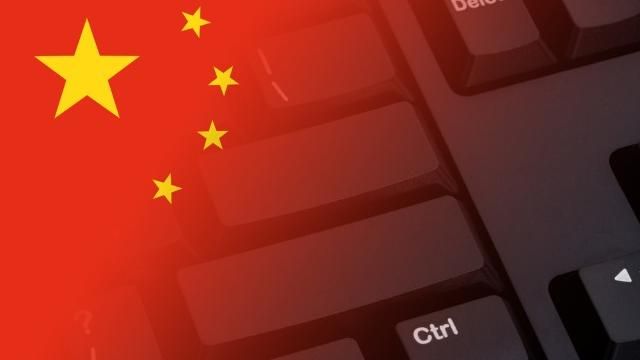
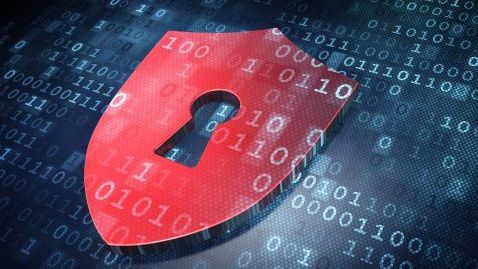
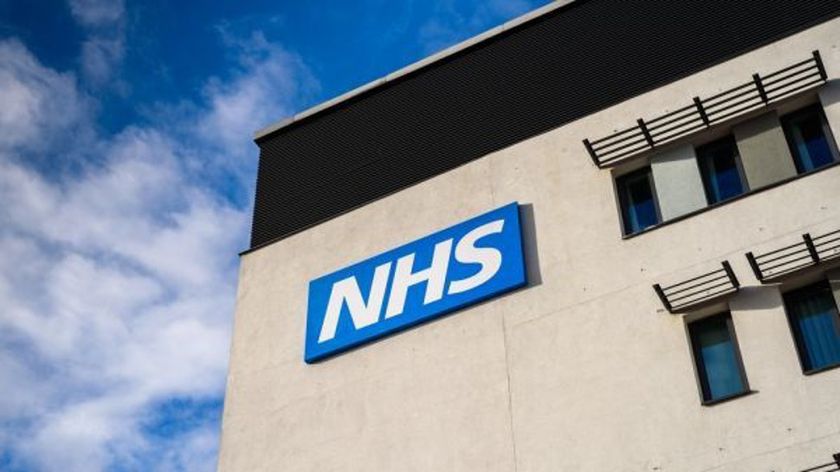
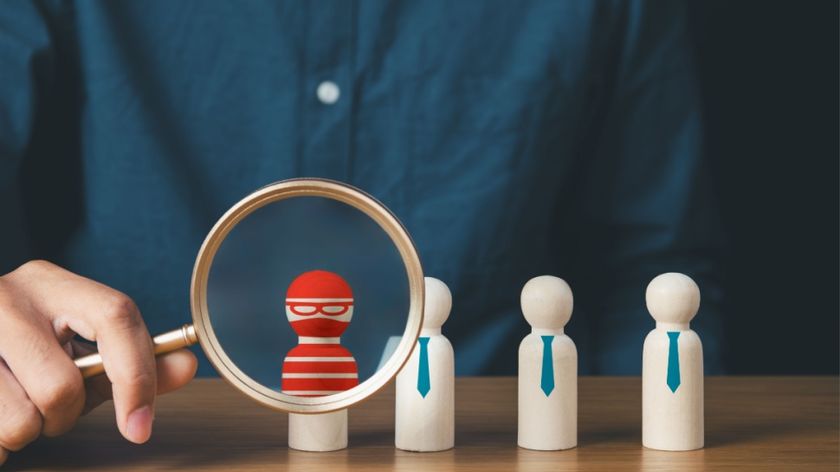
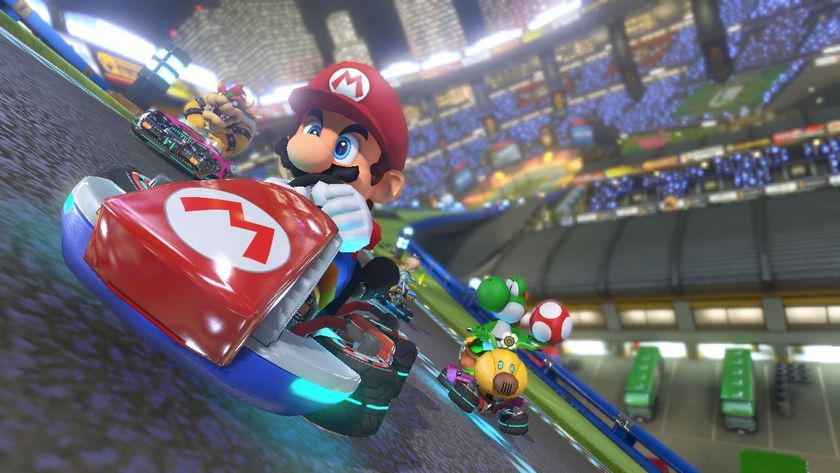
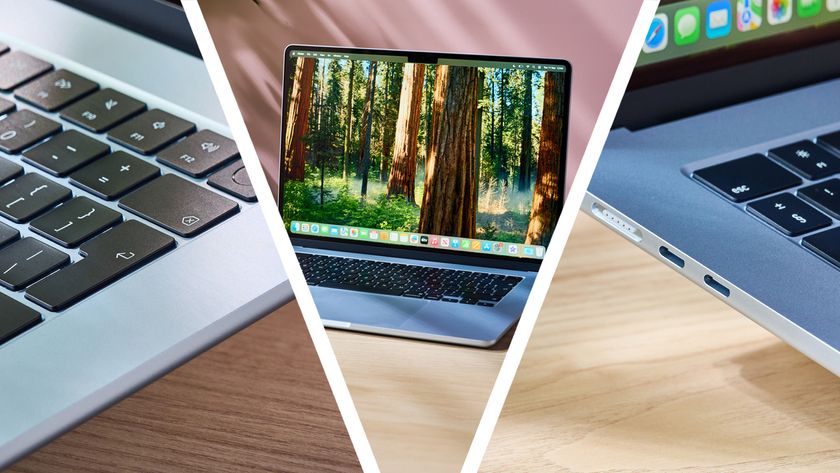
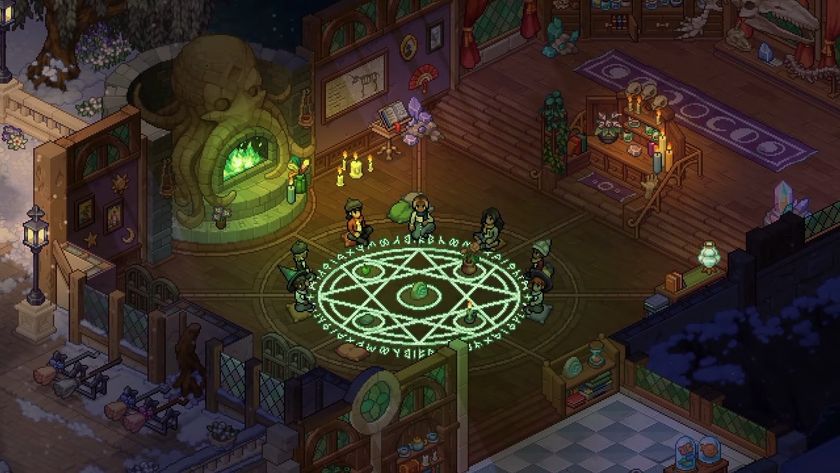
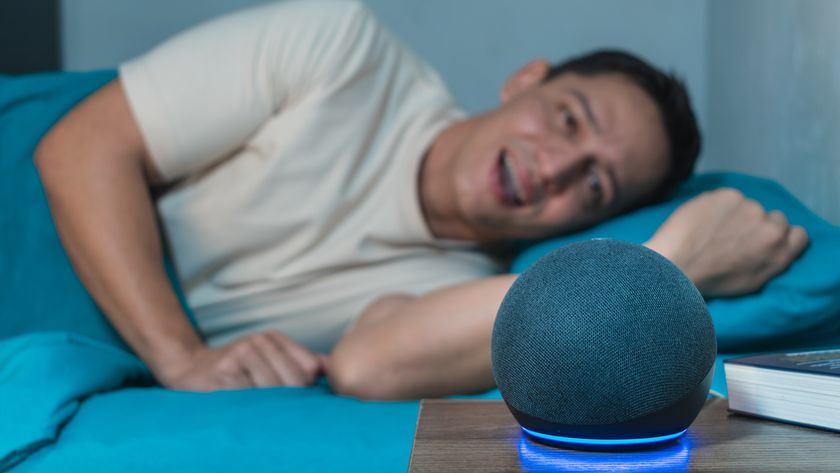
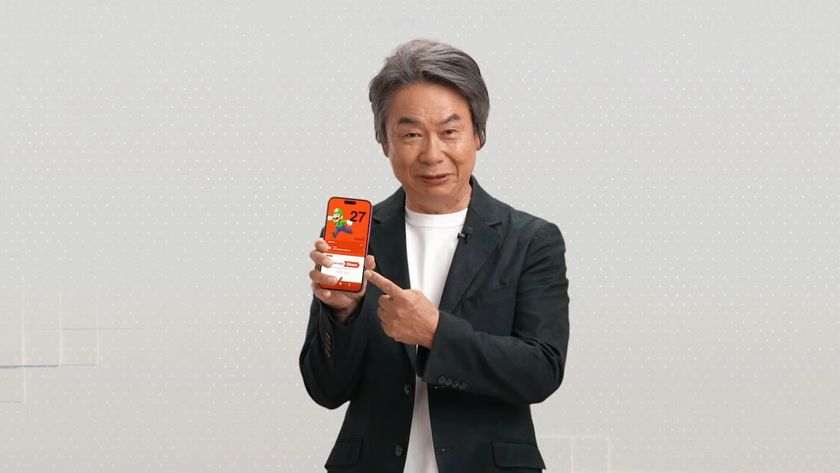
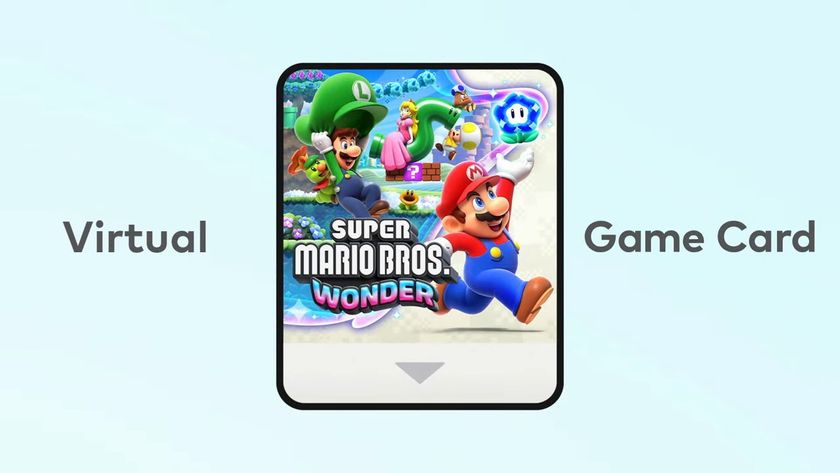