Federated Learning delivers AI to hospitals
While protecting patient data
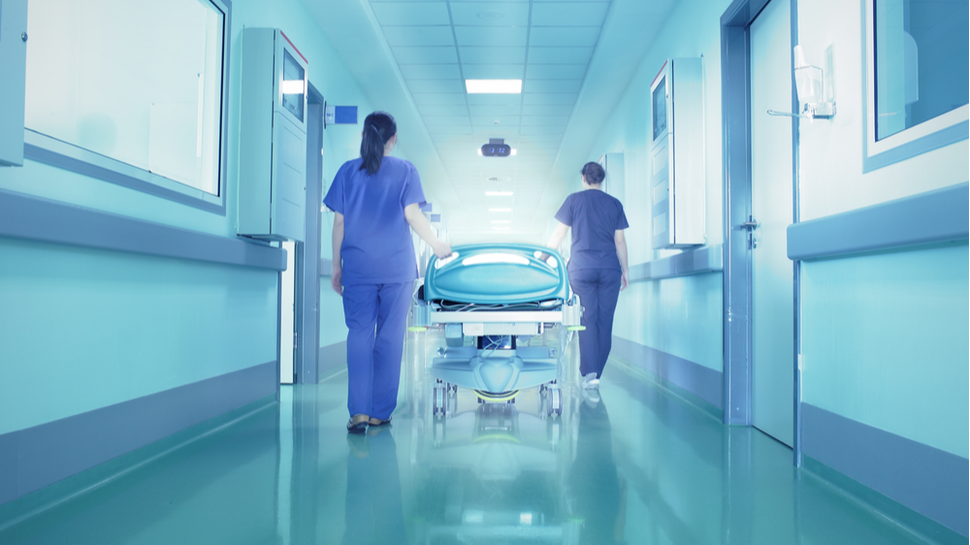
Experience is the key to success - and an almost invaluable asset in medicine, especially with regard to diagnostics. What was once learnt during studies, to translate into concrete findings, therapies and applications, is crucial for good patient care! But how can this be optimally achieved and even guaranteed?
Artificial intelligence (AI) is currently regarded as the number one future technology in many areas. It clearly shows how experience can be defined, generated and made useful. This is about the "experience" of the AI algorithms. It is fed by large, high-quality data sets. The problem, especially in the healthcare sector, is that data of the necessary scope and quality is difficult to generate.
Craig Rhodes, EMEA Industry Lead for Artificial Intelligence Healthcare and Life Science at NVIDIA.
Many institutions have to rely on their own medical records as data sources - e.g. for patient security, demography, therapies, means and methods or also for clinical specification. However, if this is not enough, they must be able to access external data pools.
This is where a new term comes into play that can offer completely new impulses: Federated Learning. This approach allows AI algorithms to turn data from different sources into exactly the "raw material" that is so valuable for medical practice management: experience. In this way, several organizations can work together to develop appropriate models without having to transfer sensitive information - such as information about individual patients' health records. In a collective effort, the collaborations involved can achieve far more than each one individually if they rely solely on the data collected in-house.
Experience – cannot be replaced
Anyone who calls themselves an expert in his field has at least 15 years of professional experience and processes around 15,000 cases per year - that is around 225,000 over his entire career. But even after three decades on the job, most people come up with a maximum of 100 particularly rare cases that make up the real expert.
How can 30 years of experience be virtually substituted? Is that even possible? If the challenge is to be tackled with AI, a correspondingly high number of cases is required, which must first be fed into the data set on which the system learns. They must all accurately reflect the clinical environment in which they were acquired and treated.
This sets the quality bar very high. At present, there are "only" 100,000 cases in the existing data sets - despite the impressive number still too few.
Are you a pro? Subscribe to our newsletter
Sign up to the TechRadar Pro newsletter to get all the top news, opinion, features and guidance your business needs to succeed!
High spectrum
It's not just the quantity that counts. The range must also be right: samples of patients of different ages and sexes from different environments are required. The archives of the individual hospitals may contain hundreds of thousands of pieces of information - but they are isolated. This is to be welcomed from a data protection perspective. However, the use on a broader front is thus ruled out.
And this is exactly where Federated Learning comes into play. This method makes it possible to share the AI algorithm without sharing the patient’s data, which is still contained in hospitals. For example, three hospitals want to join forces and develop a model for the automated analysis of brain tumors. As part of a client-server approach, a central server would house the joint Deep Neural Network - and each participating clinic would receive a copy to train it with its (protected) data.
Effect in both directions
Once the respective model has been adapted to the local circumstances and the corresponding processes have been repeated often enough, the participants can send their trained version back to the central server while the underlying data set remains in-house.
The server aggregates the different submission model versions. The updated and upgraded parameters are then available to all participants for further training. If one of the participants drops out, this does not automatically mean the end of the project for everyone else. New participants can also be added to the corresponding program at any time.
It is only an example, but it shows: Federated Learning allows you to gain general insights from local (and locally remaining) information. Everyone benefits from this.
- Feature: the best medical alert systems.
Disruption potential for the health sector
Federated learning could mean a revolution for the training of AI models - with an impact on the entire healthcare ecosystem.
Larger hospital networks can collaborate better and more closely, while benefiting from allowing access or sharing to their secure, data. This also and above all benefits smaller hospitals, such as those in rural areas: they can access much larger AI resources than would otherwise be possible.
This would bring artificial intelligence directly to the point-of-care. Doctors would have access to helpful AI algorithms based on data material that includes various patient profiles and also rare cases that do not commonly appear in all hospitals. In addition, the participants can also make their own contributions - for example as feedback if they are not satisfied with individual external results.
Healthcare start-ups would be able to accelerate groundbreaking innovations to the market with the help of secure access to various AI models and the resulting learning effect. And research institutes could better tailor their work to the needs of hospitals.
- Feature: the best medical transcription services.
First platforms already in use
In the U.K., NVIDIA is partnering with King’s College London and Owkin to create a federated learning platform for the National Health Service. The Owkin Connect platform running on NVIDIA Clara enables algorithms to travel from one hospital to another, training on local datasets. It provides each hospital a blockchain-distributed ledger that captures and traces all data used for model training.
The project is initially connecting four of London’s premier teaching hospitals, offering AI services to accelerate work in areas such as cancer, heart failure and neurodegenerative disease, and will expand to at least 12 U.K. hospitals in 2020.
Clara FL (for Federated Learning) is a platform that - running on the intelligent NVIDIA EGX edge platform - already enables several hospitals to "collaboratively" learn, while at the same time guaranteeing comprehensive data protection. The platform acts as the interface between the server and the participating clients, providing them with everything they need - from application containers to the corresponding AI model.
And already today, the platform reveals the broad spectrum of possibilities for users. Among other things, it is used to develop the necessary AI infrastructure for smart sensors in hospitals or to make mobile point-of-care MRI systems practical.
- See the best medical billing software solutions compared.
Craig Rhodes is the EMEA Industry Lead for Artificial Intelligence Healthcare and Life Science at NVIDIA. He is an Experienced Innovation Manager with a demonstrated history of working in the Healthcare and Life Sciences industry. Craig is skilled in IT Strategy, Management, Product Design, Development and Management.
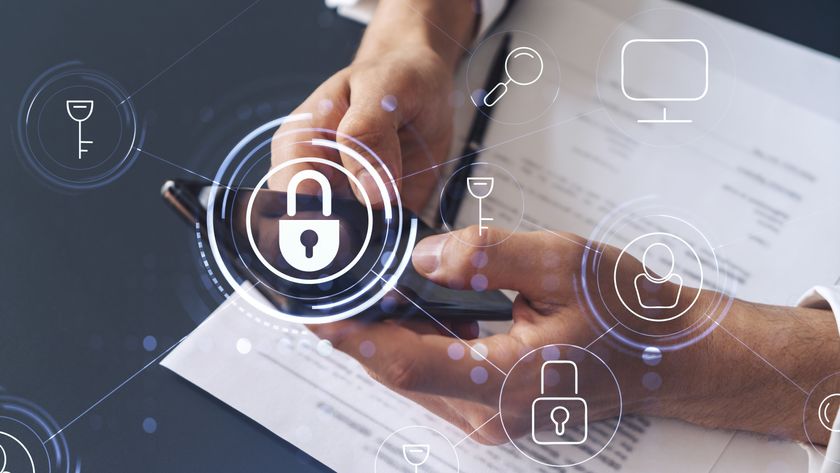
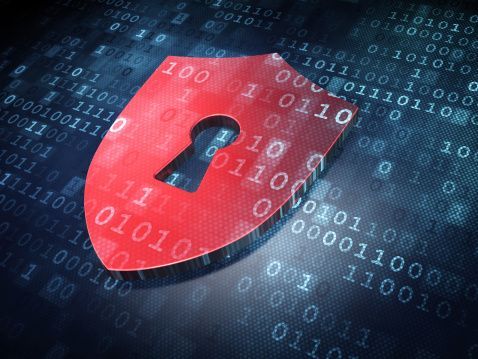
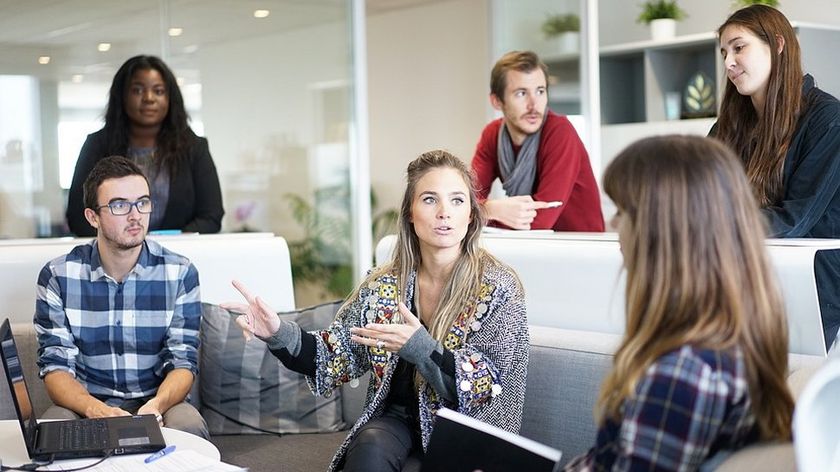
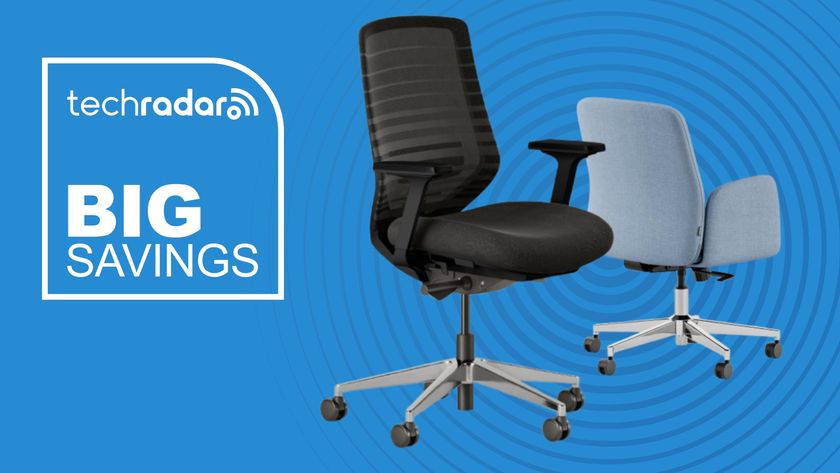
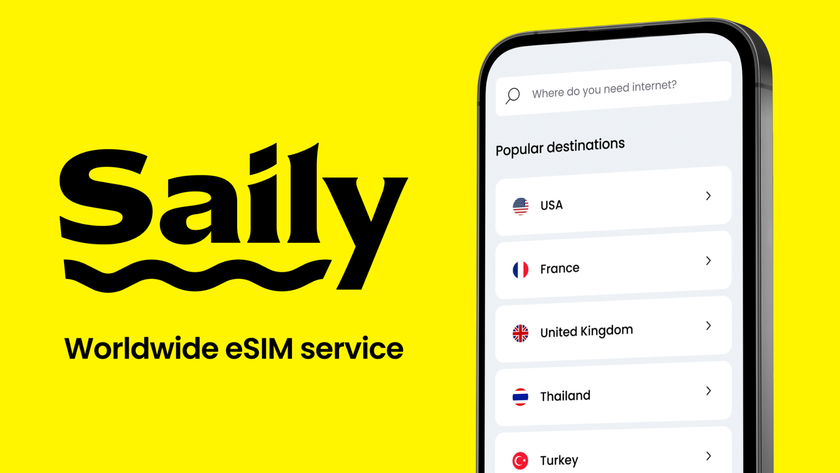
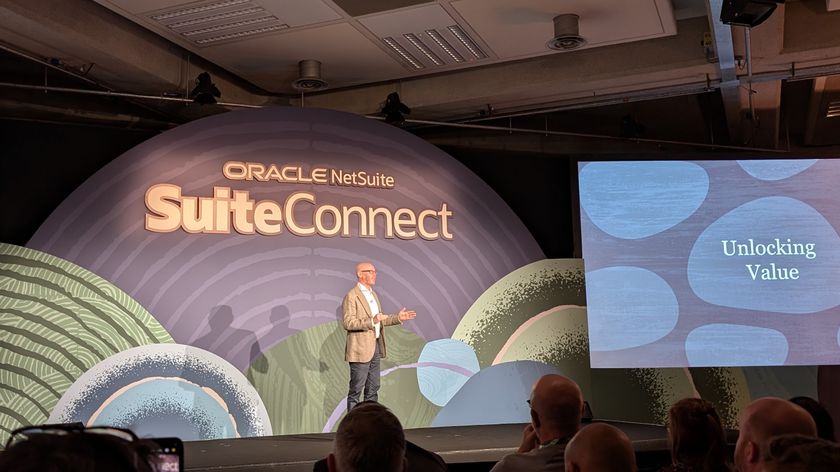
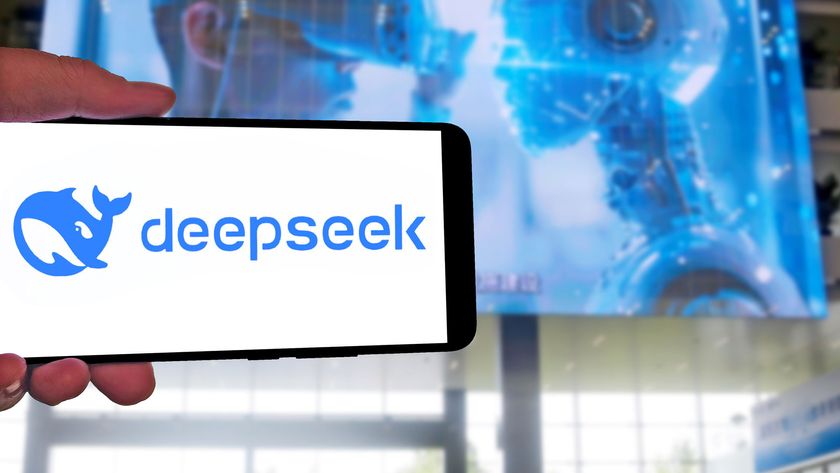
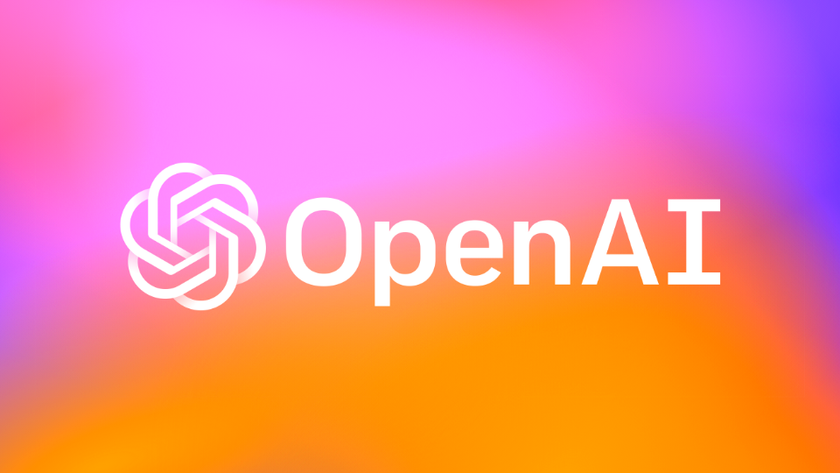
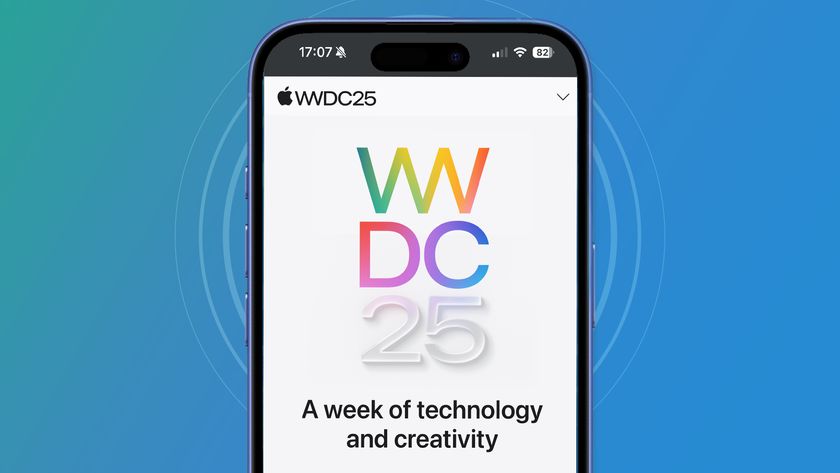
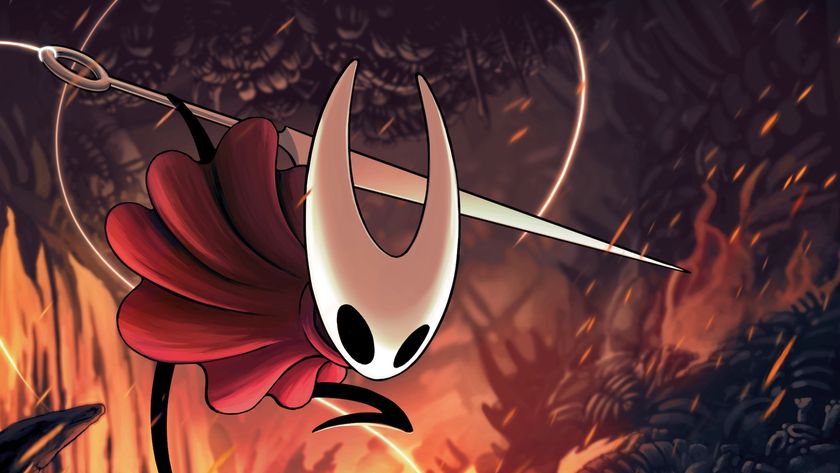
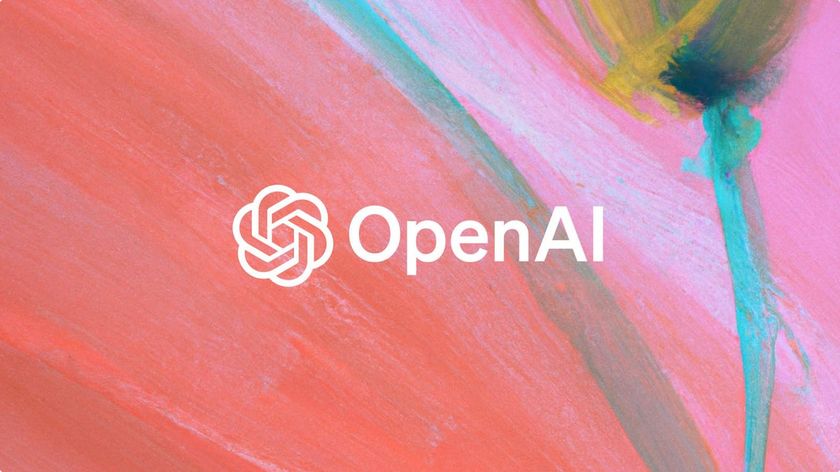
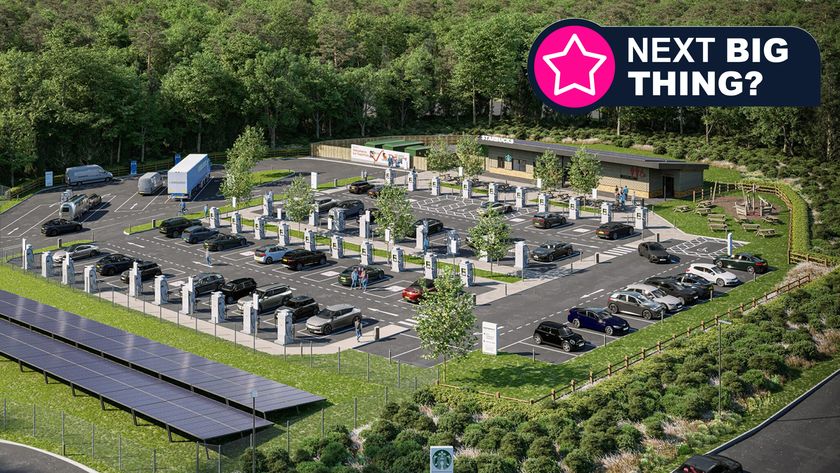