Fueling driverless navigation with AI
Artificial Intelligence systems will be at the helm
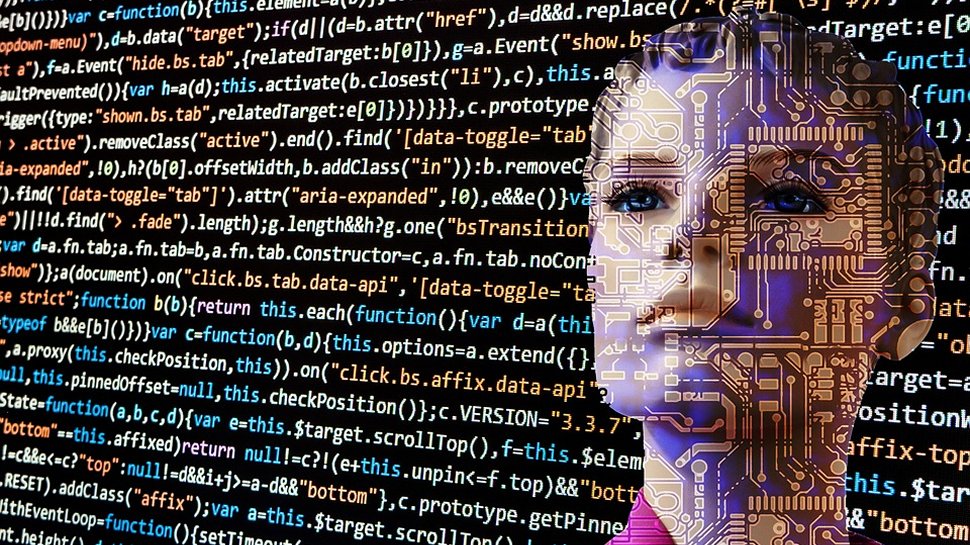
Generally, Artificial Intelligence (AI) helps to make products and procedures more efficient, but it also handles intricate tasks that can’t be easily managed by humans. When navigation comes into play, AI can help to give drivers the very best directions, despite the complexity of the route.
Pierluigi Casale is Group Data Scientist at TomTom.
Powerful, accurate maps can be created by deep learning models, able to process masses of images no human cartographer could ever manage.
AI is nothing without data
By leveraging data and the Internet of Things, the majority of car navigation systems are able to alert drivers of any travel disruptions and readjust the journey route accordingly. Yet few are complex enough to anticipate how the traffic situation will change during the travel time on any possible route. In the European road network alone, a hundred quadrillion routes are theoretically possible. Machine learning, therefore, allows for this through a process called dynamic routing, which helps AI navigation systems to actually predict how traffic will change and how the journey will be disrupted.
With dynamic routing, drivers and automated vehicles can drive with foresight. However, building the AI models needed isn’t the hard part. It’s data that makes the difference. For instance at TomTom, immense quantities of image data depicting street views are needed to create high-definition maps. To ensure the navigation system is responsive, there’s also a need for data on the same streets under a wide variety of environmental and weather conditions. The more data there is, the more accurate the maps will be.
An enormous amount of data is required to train AI models so that they truly represent reality. The job of photographing every stretch of road in every weather and lighting condition is obviously impossible. Abstracting the process through AI, however, can help us achieve such “impossible” but vital tasks. Through the use of novel generative algorithms, it’s possible to train AI to take one image and apply different conditions to it. For example, the AI could simulate the same street at night or during a blizzard. Thus, when an AI-enabled navigation system encounters atypical conditions on the road, it can adapt rather than lock up. This is a crucial step in helping AI not only to recognize roads but also respond to them.
Increasingly, car and mapmakers also need to keep privacy and security in mind. Few data is as sensitive as customer location history, proving a challenge for those who rely on large-scale data to ensure the accuracy of their maps. Privacy-aware machine learning, made up of AI algorithms that learn from anonymized raw data, is the answer. Once trained, the models can be shared, allowing companies to continue to train and enhance the full pool of shared models with new ones.
Community driven map-making
Keeping digital maps accurate and up-to-date is essential, and to do so community engagement becomes increasingly important. Car manufacturers now need to develop – or seek out - navigation systems with a built-in community of people who are willing to help keep maps accurate. Whether it’s through app development or an interface which allows people to contribute, drivers can share images of where reality doesn’t match what has been recorded, such as road closures or road signs. This then alerts mapmakers to check and fix the map as necessary.
Are you a pro? Subscribe to our newsletter
Sign up to the TechRadar Pro newsletter to get all the top news, opinion, features and guidance your business needs to succeed!
The challenge of building this community, however, is consumer suspicion. As the world becomes increasingly more aware that services such as online mapping tools can often be ad-funded, customers are less likely to share their data. How can we trust these service providers to send us the quickest route when in-fact what they’re doing is sending us on a detour past one of their advertisers? That’s why car manufacturers need to seek out a navigation system that isn’t advertiser funded.
As our transport systems continue to develop, and as we travel ever closer to a fully autonomous future, AI based navigation systems will become a critical component in our vehicles. In order to ensure their success, manufacturers will need to ensure the data used to inform these systems is as detailed and accurate as possible. Ensuring data comes from a range of trusted sources is the key to unlocking the full potential of digital maps. If this data is then abstracted through modelling and crowd sourced information, the future of AI-assisted navigation is looking brighter than ever.
- We've featured the best fleet tracking solutions.
Pierluigi Casale is Group Data Scientist at TomTom. He has proven experience in using data as a strategic asset and finding the relevant business value, innovator in building data products, machine learning solutions and analytics infrastructures.
Pierluigi is very pragmatic and results focused, and he support teams to make decisions around algorithms, architecture, data strategy, hiring, and organizational structure. As a leader, Pierluigi coach teams in the design and creation of data-driven solutions, and in executing projects in fast-pasing environments. He is an excellent communicator, able to forge solid relationships with strategic partners and build consensus across multiple organization levels. He is also a technical evangelist that strives for the wide adoption of data-driven methods.