Machine learning in the cloud: beyond Kinect and Cortana
The brains behind Microsoft's Azure Machine Learning
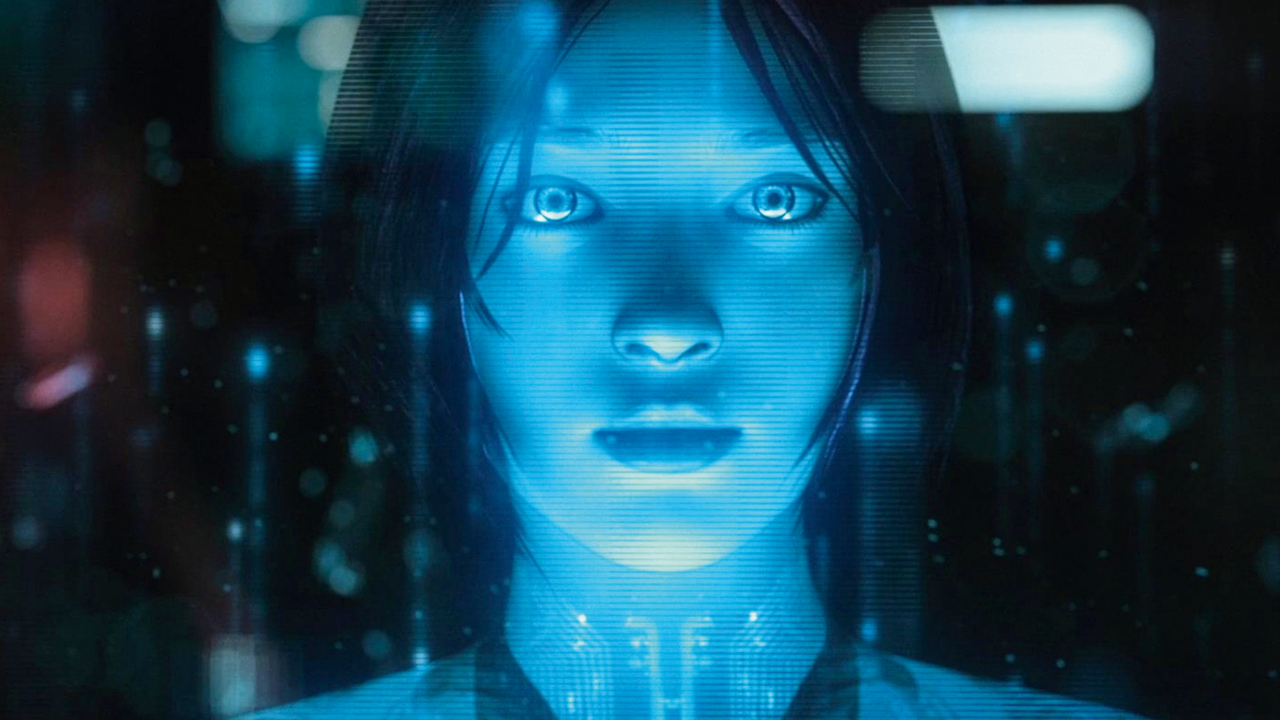
Machine learning is behind more and more of the technology we use every day - and it's not just voice recognition in Kinect and Cortana or Microsoft's futuristic language translation in Skype.
Every time you get directions from your GPS or make a credit card transaction or search for a product online, machine learning is predicting the best route, working out whether you're likely to be using a stolen credit card and suggesting what else you might like to buy.
So far you've had to be a company with the resources of Amazon or Yahoo to take advantage of machine learning. With its new Machine Learning (ML) Studio service running on Azure, Microsoft is hoping to open it up to anyone who understands statistics – and make it easy to use the predictions from the machine learning models you come up with in the apps where they will be most useful.
"I'm building this to be easy enough for a high school student to use," Microsoft corporate vice president Joseph Sirosh told TechRadar – and he knows how hard machine learning can be, having built Amazon's recommendation engine.
With ML Studio, Microsoft is giving businesses access to the tools it uses internally. Microsoft has been working on machine learning for two decades, Sirosh points out. "It's integrated into Bing, into Xbox, into the fabric of most key products we have – including Cortana. We have a tremendous amount of experience with machine learning and how to do it at internet scale and we're bring a lot of that experience into the product."
Gaining XP
You will still need to be a data scientist or at least have mathematics and statistics experience to get the most from the service, which you'll be able to try out in preview next month. But that's not what made McKinsey say businesses can't find the hundreds of thousands of data scientists they want to employ.
"It's not that people don't exist with the math knowhow; every graduate in engineering or mathematics or statistics will have some of the background to be productive data scientist and machine learning people," says Sirosh. It's that they haven't had good, fast, cheap, simple tools to work with.
Are you a pro? Subscribe to our newsletter
Sign up to the TechRadar Pro newsletter to get all the top news, opinion, features and guidance your business needs to succeed!
"Today data scientists have to know so many complex tools; they have to be both a data engineer and a mathematician to get things done," he explains, adding that they might need 10 different packages to try enough machine learning models to solve one problem.
"And those tools are extremely expensive and have a big learning curve; you have to be spending a huge amount of time to get productive with them. It's a huge stumbling block.
"What we're doing here is to make this so much simpler; you just have to know your data, know how to set up and frame your problem and then build the machine learning model. And for deployment, previously you had to hand it over to IT or to an engineer with lot of sophisticated programming experience to hook up. Now the data scientist can do it.
"The way we are changing the game is we allow you build these scalable systems in the cloud that can handle any transaction load, that allow you to do sophisticated deployments with very little effort and that is incredibly empowering. What we're changing with this tool is extending the reach to a very broad class of developers. You can hook it up to a web site and it will just work!"
That's a far cry from the complexity of big data systems, which is part of the reason Gartner's hype cycle report has just said big data is not delivering the benefits to most companies that have been claimed for it.
"We are really hoping to pull big data out of its trough of disillusionment," says Sirosh, "and the reason for that disillusionment is that today big data allows you to store big data but analysing it and making use of it is incredibly hard, and this it is very hard to hook it up in operational systems. That's key.
"At the end of the day if you want to get real benefit out of it you have to hook it up to systems that actually affect customers or help you anticipate things and create benefits in automated ways. That kind of automation is what our tool really excels at."
Mary (Twitter, Google+, website) started her career at Future Publishing, saw the AOL meltdown first hand the first time around when she ran the AOL UK computing channel, and she's been a freelance tech writer for over a decade. She's used every version of Windows and Office released, and every smartphone too, but she's still looking for the perfect tablet. Yes, she really does have USB earrings.
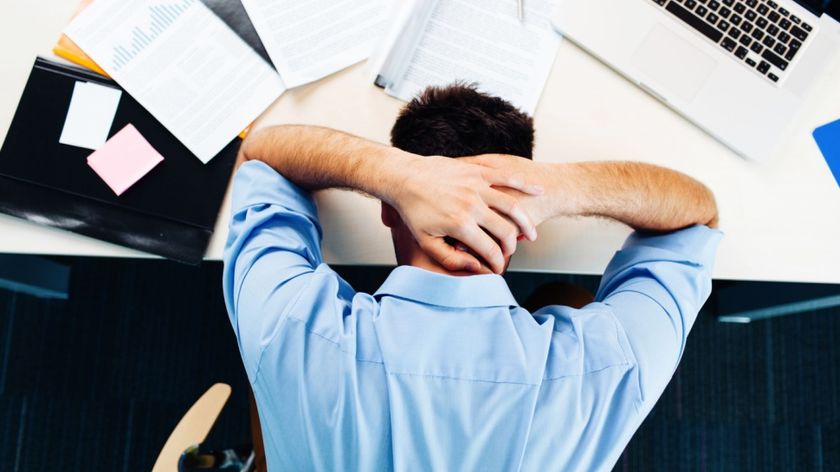
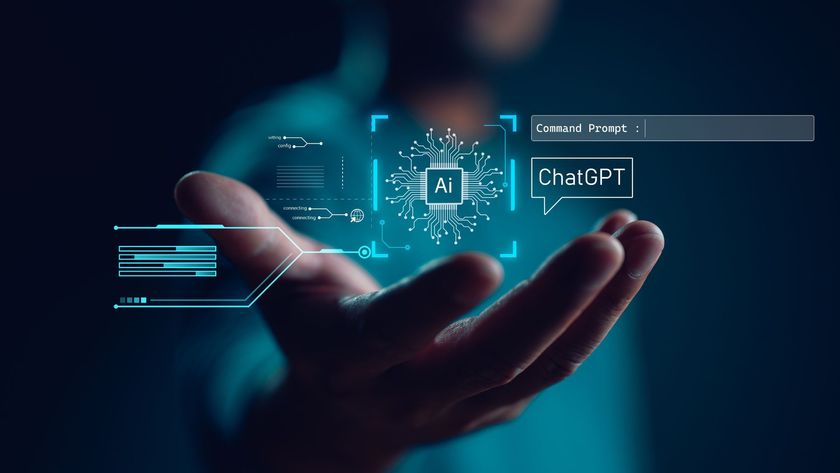
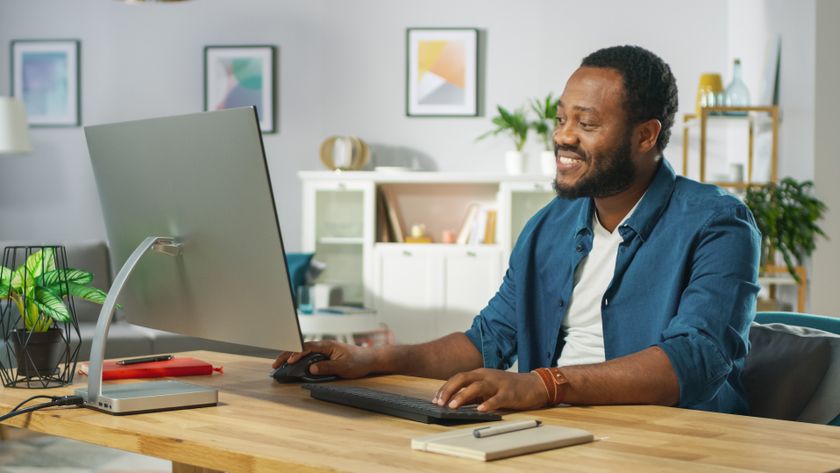
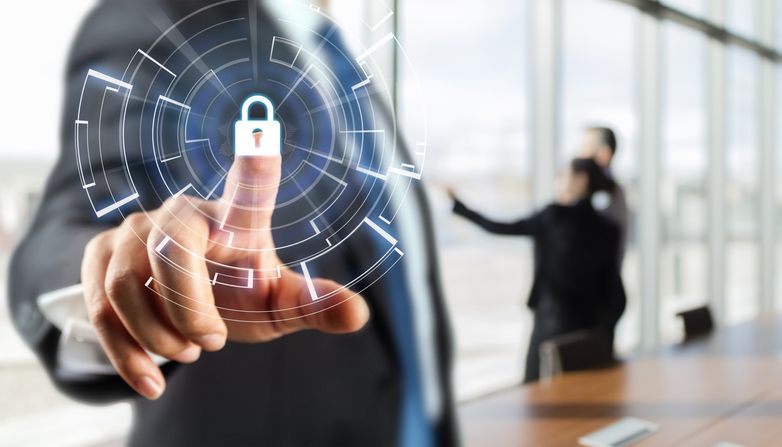
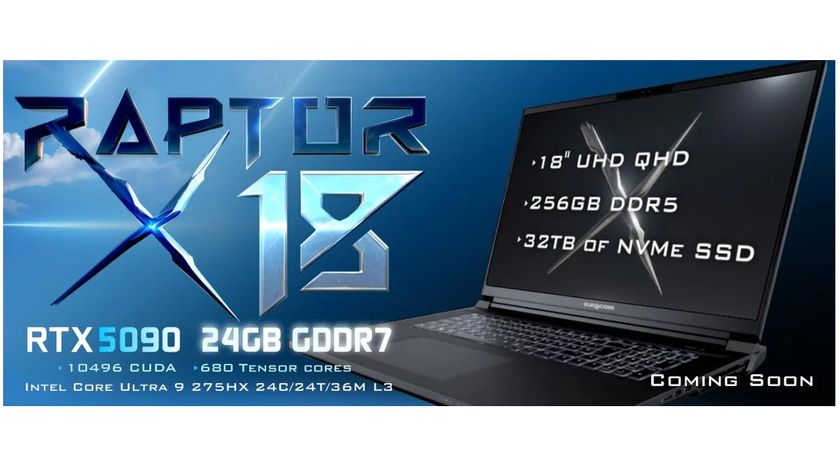
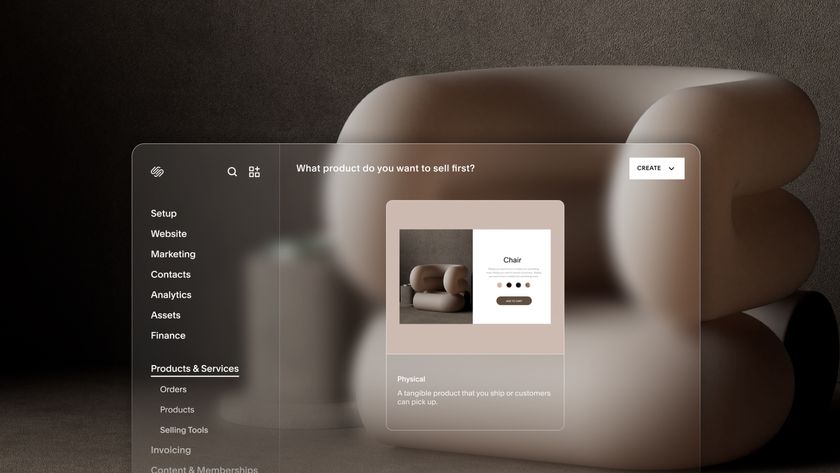
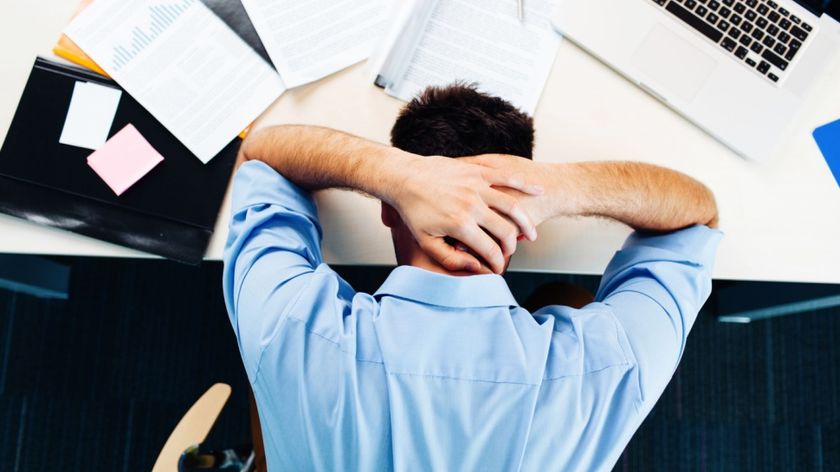
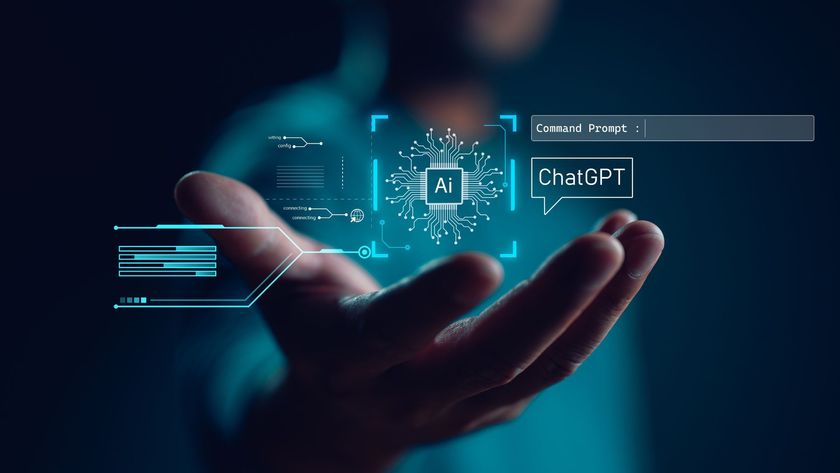
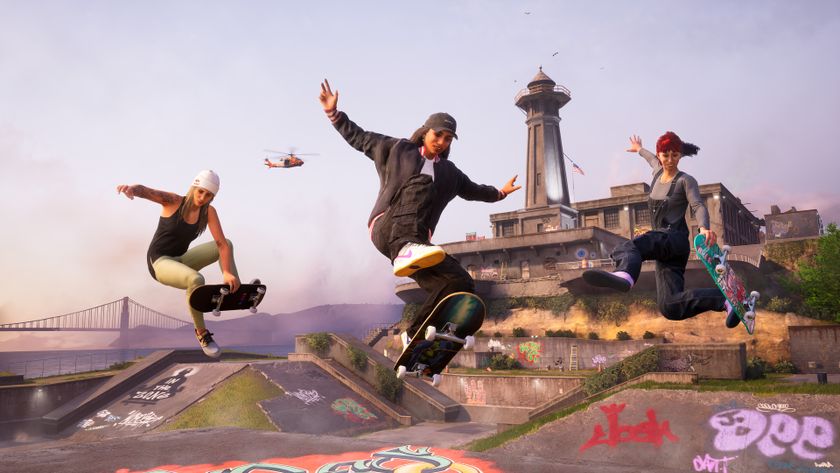
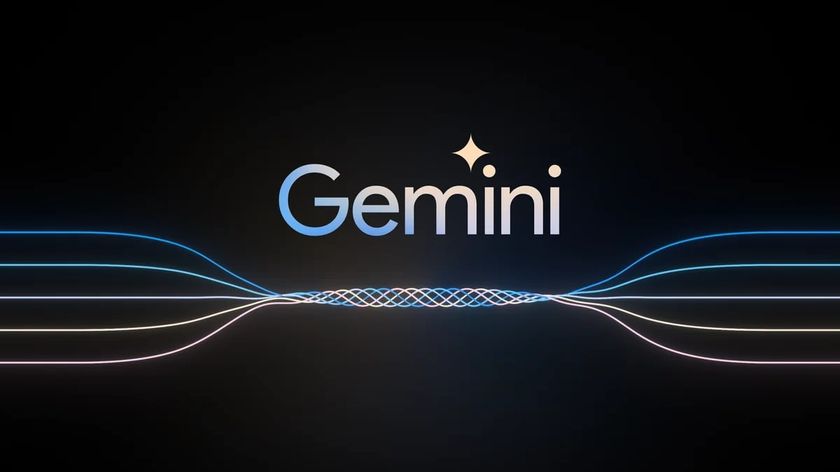
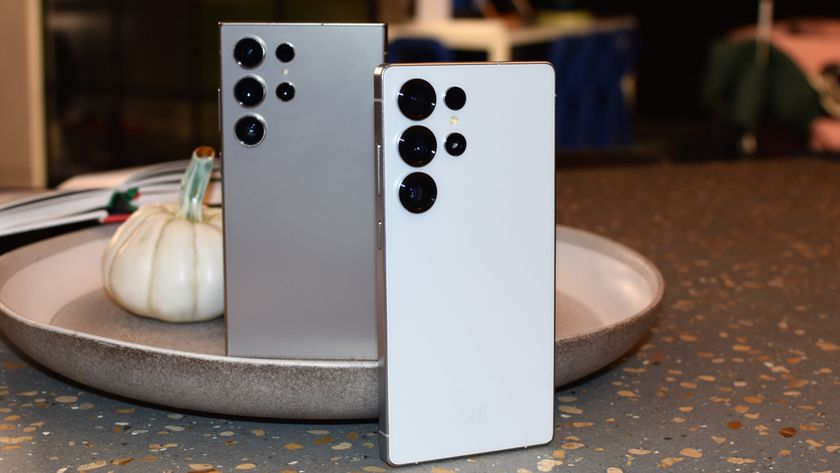
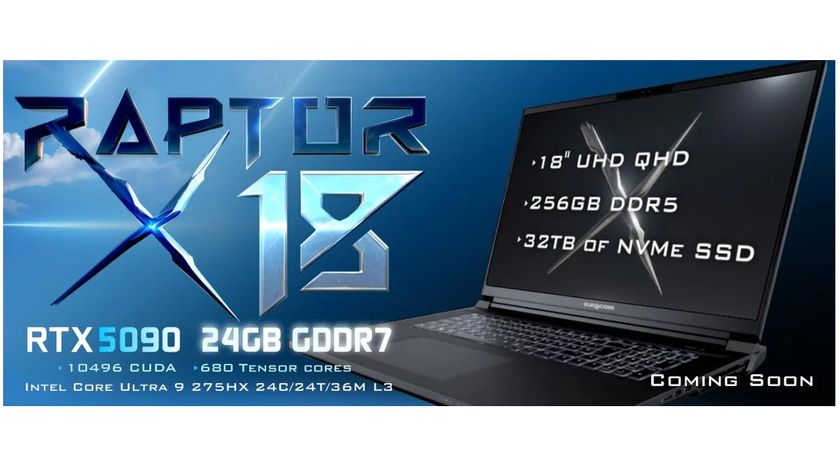