Harnessing in-memory computing
A route to exploiting big datasets
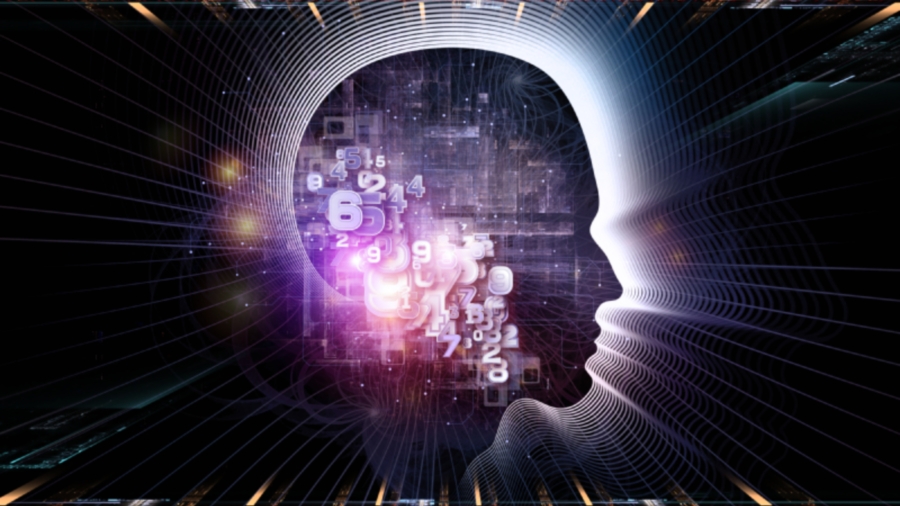
As the quantity of data that a business gathers and analyses increases, new forms of data management are developing, one of the most promising of which is in-memory computing.
It involves the storage of information in the random access memory (RAM) of dedicated servers rather than in relational databases on disk drives, and makes it possible to query vast quantities of data.
With in-memory computing, the data being queried is moved as close to the processor as possible, thus eliminating any bottlenecks that may occur with, for instance, cloud based data warehouses. The typical high latency values of disk based data storage are vastly improved with in-memory techniques.
For IT managers looking to improve the agility of their businesses, in-memory computing offers a potentially huge increase in the speed of data analysis. A recent InformationWeek survey indicated that the number one area of concern among IT managers is the speed of access to data, and the technology can play a significant part in dealing with the issue.
In-memory databases have been in existence since the late 70s, but only now do we have the platforms needed and the high-speed processors to make in-memory computing economic and useful. Currently there are a number of vendors in this sector, including SAP, which offers its HANA platform with Oracle Exalytics, including a Sun Fire server with 1TB of RAM, powered by the Intel Xeon processor E7-4800 with a total of 40 processing cores, 40 Gb/s InfiniBand and 10 Gb/s Ethernet connectivity, and integrated lights out management.
In-memory computing techniques can offer a number of benefits including: improved big data analysis; reduced overall data latency levels; shorter batch processing times; improved business intelligence as big data reveals patterns and correlations; the ability to run entire datasets (not just samples) to analyse potential patterns such as transactional behaviour; and to query unstructured datasets using in-memory analyse techniques.
In-memory analytics
The increase in popularity of in-memory computing is closely linked to the falling price of dynamic RAM (DRAM). With a typical in-memory installation using 1TB of DRAM this is good news for CIOs who want to expand their use of big data silos yet keep acquisition costs to a minimum.
Are you a pro? Subscribe to our newsletter
Sign up to the TechRadar Pro newsletter to get all the top news, opinion, features and guidance your business needs to succeed!
Coupled with this is the increased popularity of 64-bit operating systems, and multi-core processors.
Subsequently, IT managers are suddenly benefiting from a perfect storm of IT developments.
They could be forgiven for thinking that the adoption of in-memory computing is little more than a hardware upgrade to eliminate data processing bottlenecks. But there is more to it to really produce the benefits.
The key consideration is how the big data set it stored and accessed by the in-memory system. Moving what could be dispersed datasets into a single data silo that the in-memory platform can query is a logical first step.
There is also the question of cost. As the market for in-memory is still maturing, many IT managers may need to take a bootstrapping approach rather than invest heavily. However, in-memory runs with commodity servers, which will helps to alleviate the costs.
In addition, an audit of current data silos and how these might expand is critical to the in-memory computing buying decision.
Advanced business intelligence
The size of the datasets that a business is managing is an indicator of need. While big data is still the preserve of relatively few, its adoption is expanding, and companies that do not see in-memory as an imperative today could do so in the near future.
Also, business intelligence is moving towards a complete self-service platform. In-memory computing offers for the first time an almost real time interrogation of vast datasets by anyone within a company.
Add to this the moves towards 'bring your own device' and suddenly in-memory systems offer an unprecedented level of access to data analysis.
The future of in-memory computing looks set to become more cloud based. Already Stanford University is developing what is called the RAMCloud, which uses the concepts of holding data in DRAM and applying these to the cloud.
In effect, the cloud could become a DRAM memory extension that any business could use for high speed querying.
As Stanford concludes in a paper on the subject: "The two most important aspects of RAMClouds are (a) their extremely low latency and (b) their ability to aggregate the resources of large numbers of commodity servers. Together, these allow RAMClouds to scale to meet the needs of the largest web applications."
Business intelligence is now a key component of every company, and in-memory computing complements how it has evolved over the last few years. It's now a question of when and not if a company moves to in-memory data analysis.
Platforms are evolving rapidly, which means all due diligence needs to be applied to the buying decision. Once in place, however, businesses can begin to view the data they hold in a whole new light.