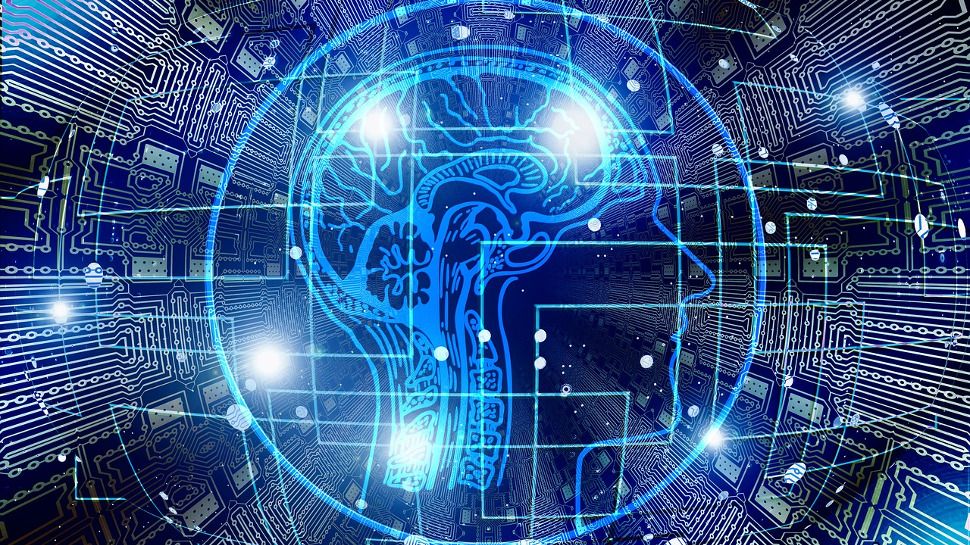
Lately it seems that AI is our knight in shining armor, the missing link between our past and our future. AI is now being used to steer the direction of hedge funds and drive much needed efficiency upgrades to our supply chains, for example. The machine learning society introduced a face recognition algorithm that is able to distinguish gender with an accuracy of up to 91%. AI is becoming so commonplace, in fact, that our electronics are often using it in the background to improve our experience, from taking a picture or securing our devices, without us even knowing it. Smaller AI startups are introducing AI services that can help sales teams surface talking points that closes deals.
AI isn’t just changing our future. It’s prompting new trends in hiring and speeding up acquisitions. According to Element AI, a specialty lab in Montreal, “in the entire world, fewer than 10,000 people have the skills necessary to tackle serious artificial intelligence research.” The skills shortage, combined with immense industry hype, has prompted a heated race for the biggest technology companies to invest heavily in AI, even acquiring AI startups in their earliest stages of research and funding.
Yet AI adopt outside of the tech sector often remains in an experimental stage. In one McKinsey survey of 3,000 AI-aware C-level executives across 14 business sectors, only 20% said they “currently use any AI-related technology at scale or in a core part of their business.” When asked why, most executives admitted they are uncertain of the business case or return on investment. Behind the hype and “AI is everywhere” feeling, the majority of businesses remain on the fringe, unsure of what move to make next.
- The foundation for your AI future
- AI’s memory is perfect for insight into collective behaviour
- Three ways CIOs can successfully scale AI
As we continue to tell the futuristic tales of AI, the excitement will only continue to grow—and rightfully so. In the last 5 years, the advent of cloud computing and easy access to large data sets has taken many of these futuristic AI scenarios and made them both possible and affordable. And it seems we are only at the beginning. But how can business leaders determine if it’s practical and realistic to leverage AI and machine learning today?
The nuts and bolts of AI
AI is entirely dependent on access to quality data. Researchers at McKinsey admit that there are no shortcuts for firms, when it comes to utilizing AI. Its dependence on a digital foundation and access to quality data means that organizations must have the proper systems and data sets in place before they can dive in. If and when businesses declare their hopes and dreams for AI, they might as well include a disclaimer that reads “success dependent on access to large volume of good data.”
In a consumer-focused AI scenario, it’s easy to see why we’d need broad sets of data to see technological advancement. For example, it only makes sense that to find trends in data sets of cancer patients and apply machine learning to predict cancer, you’d need data about patients who have cancer. But for the 99% of companies who are looking to leverage AI across their own data sets, how can we derive value?
In short, data is only valuable as the information it provides. This is precisely why, in the race to win in AI, we should be intently focused on customer data. This huge chunk of valuable data is often thrown away by companies, even though the most valuable data is just sitting in these conversations between businesses and their customers.
Are you a pro? Subscribe to our newsletter
Sign up to the TechRadar Pro newsletter to get all the top news, opinion, features and guidance your business needs to succeed!
Conversational AI- the next frontier
At RingCentral, we’re taking a beneficiary-first approach and stepping back to ask our customers what their customers want and need. In short, they want to be able to talk with their communications platform, and interact with it. As we build with the intent of solving for the end users’ problems, it’s easier for our customers to immediately see value from our platform.
Applying a customer-first approach to AI development has led us to some unique learnings. Consumers who are speaking to Siri, Alexa, and Google Assistant now expect to find this same well-refined and ease-of-use across their workplace tools and technology. In fact, we believe the same consumer technology cross-over we saw with mobile devices and apps will also happen with virtual assistants, and we have invested accordingly (hyperlink our Alexa products)
A customer-first approach also focused us on driving ‘practical innovation’; that is, applying interesting new technologies to use cases that will actually change business outcomes. For example, AI enthusiasts love talking about sentiment analysis and automated note taking when it comes to communication, but “so what”? What do those capabilities do to impact what businesses care about? What type of AI-based scoring will help you surface important patterns that you want to replicate across your workers? How will having a running record of a customer’s tone improve sales outcomes, speed up case closures, or improve overall customer experience?
Finally, we need to be aware of a major difference between consumer versus business use of AI. Although most consumer services have access to large volumes of user data, the AI models built are typically highly generalized. This may be okay when the consumer application used are narrow in scope (for example, “Alexa turn on my kitchen lights”), but in business context the AI models likely requires more tailoring (for example, “Identify conversations about GDPR requests from our Xavier customers this fiscal quarter”). Individual businesses likely will need transcription, topic extraction, and other AI use cases to leverage models to be specific to themselves, or at least their industry.
Like many of our peers, we’re working quickly to incorporate AI and machine learning into our platform, in order to reap the biggest benefits. It makes sense that technology and telecommunications companies are leading the AI charge; our customer data is rich and we have room to explore. We continue to learn, through partnerships with Gong.io, Chorus.ai and others, that to win the AI game, you have to bring it back to the basics. What data do you have at your fingertips and what value can you deliver to customers? The answer to that question can almost always be found in the conversations you’re already having with customers.
David Lee, VP of Platform Products at RingCentral
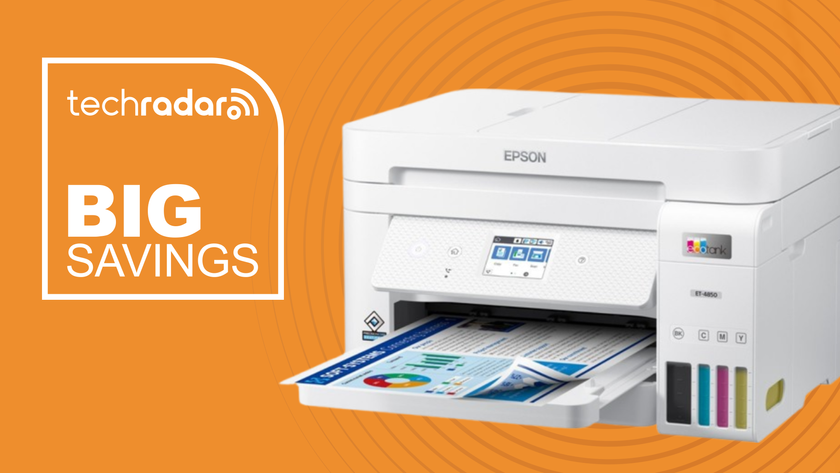
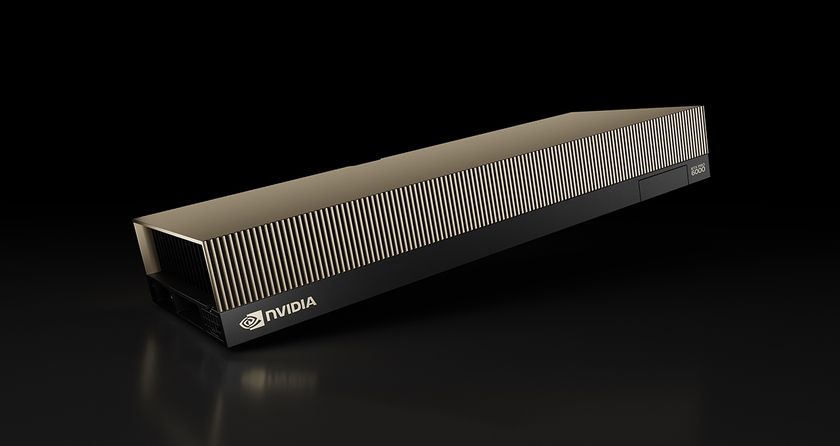
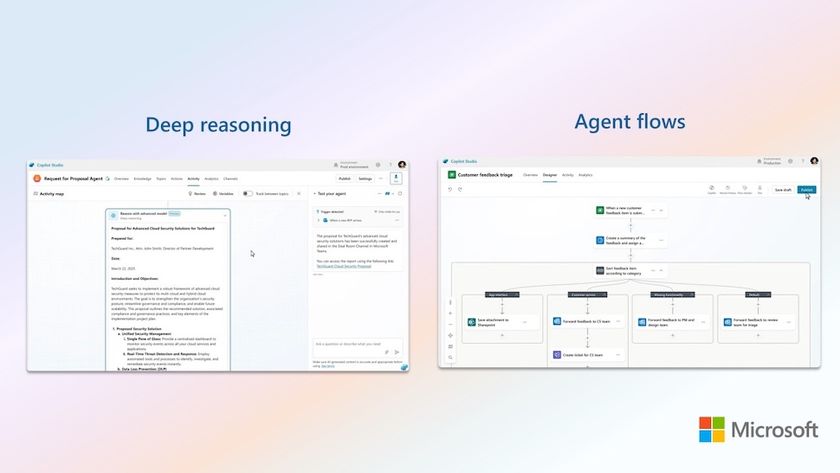
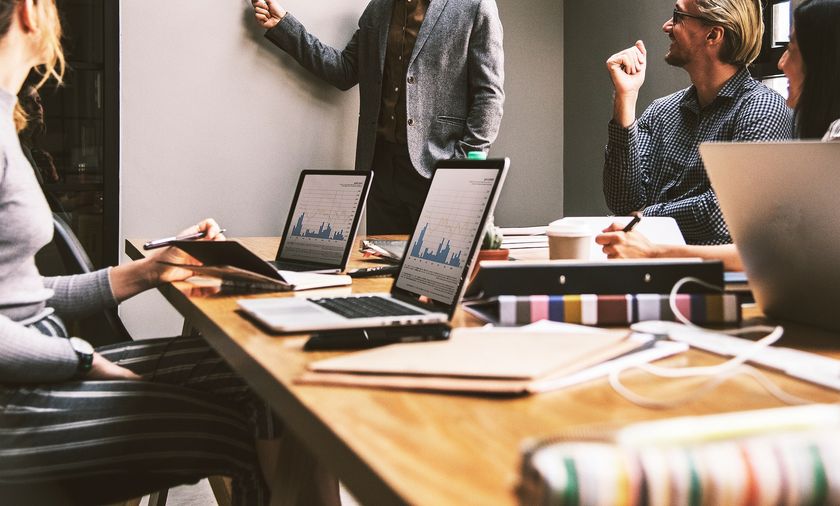
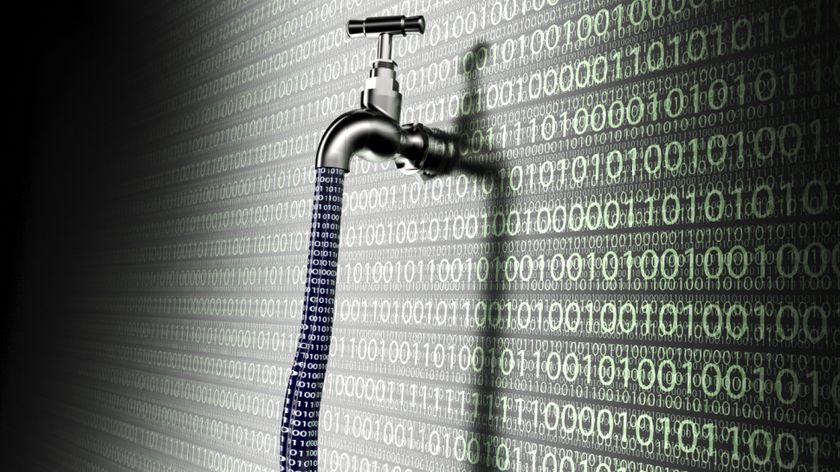
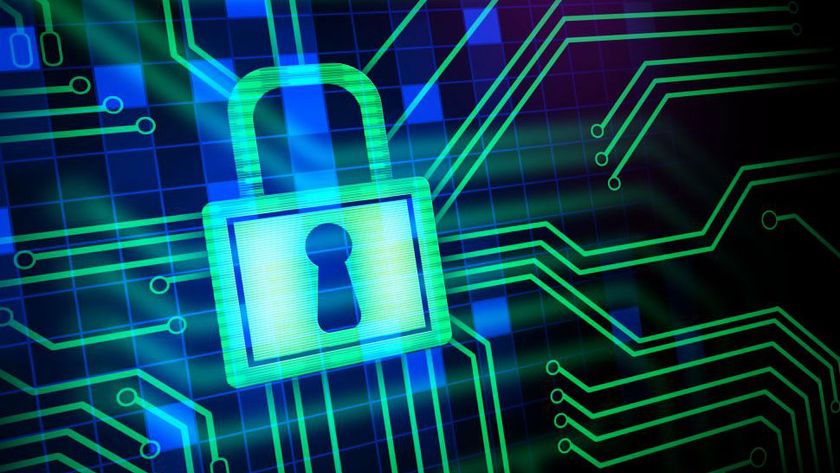
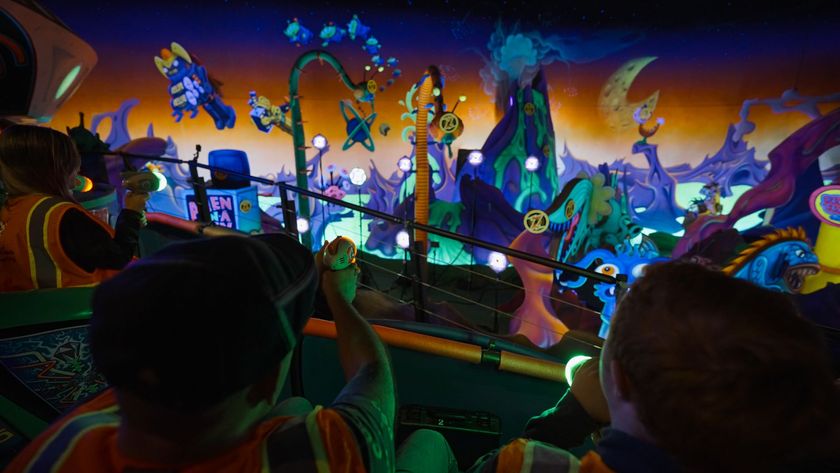
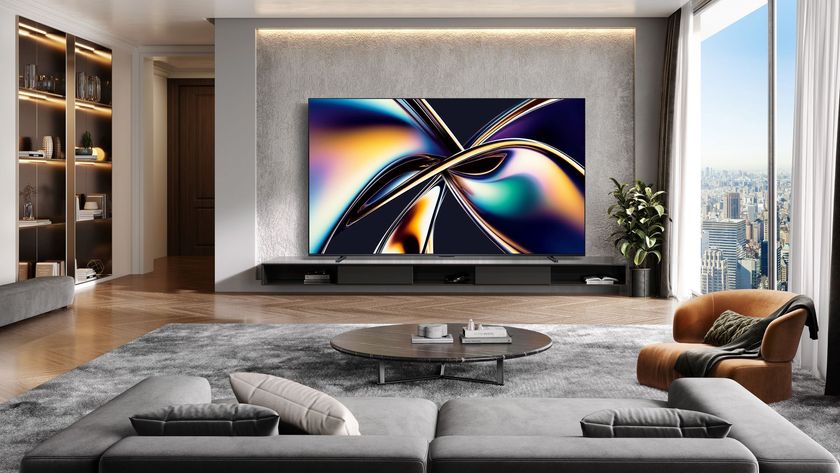
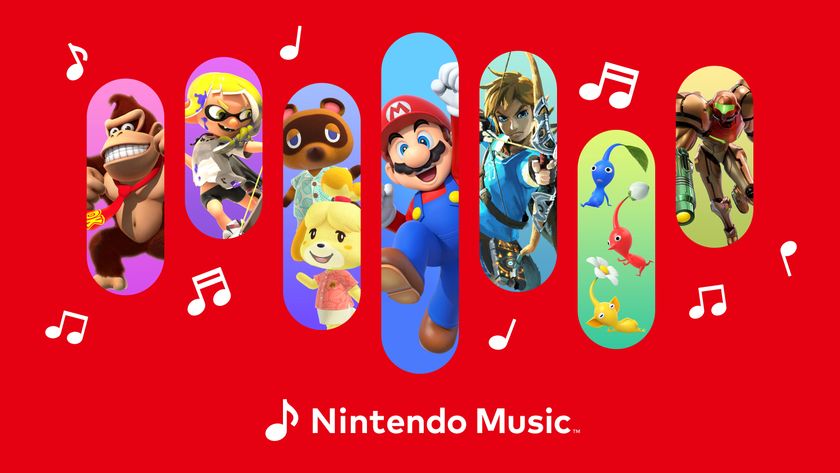
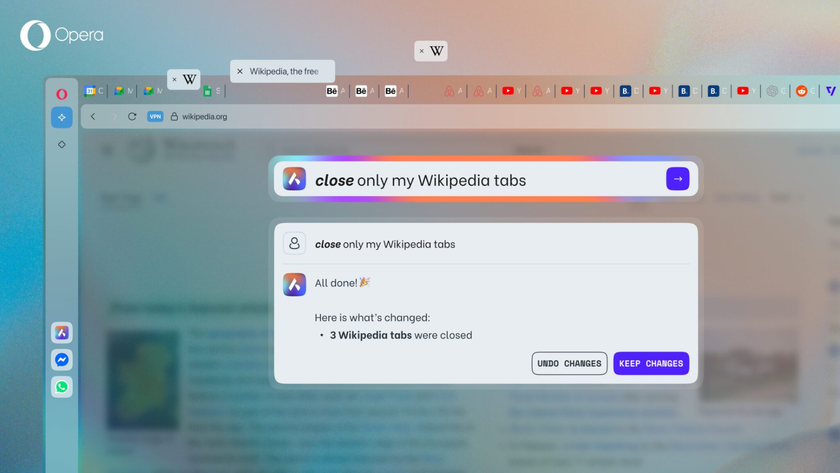
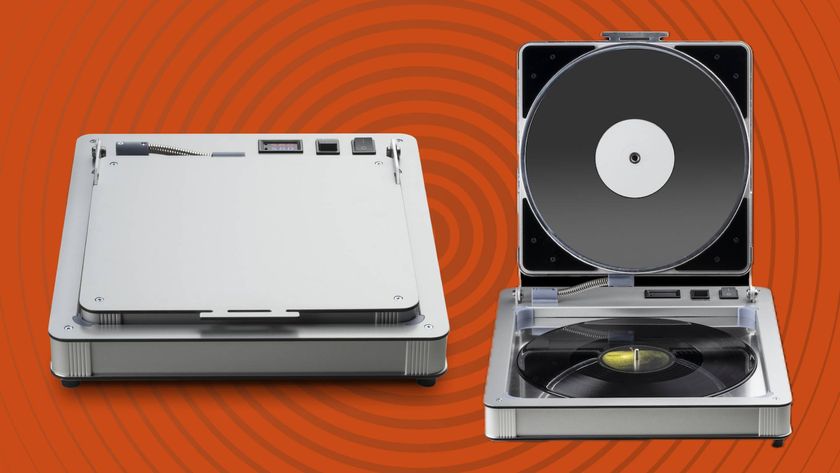
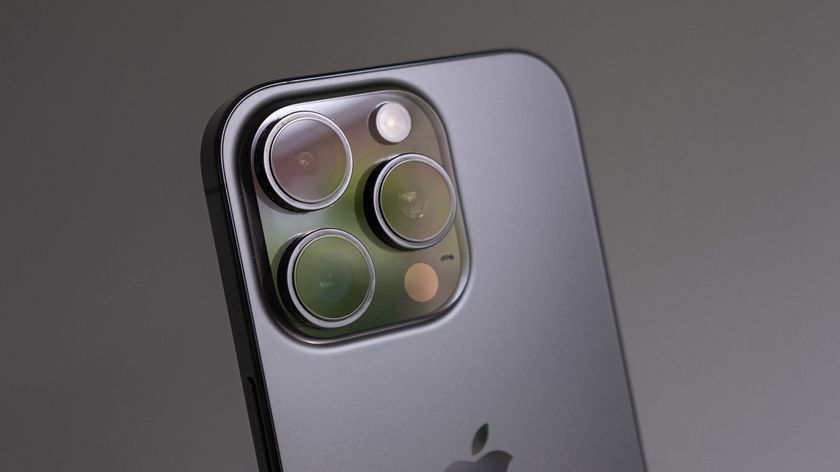