The path from predictive to prescriptive analytics
Businesses are using advanced software and machine learning insights to automatically take action
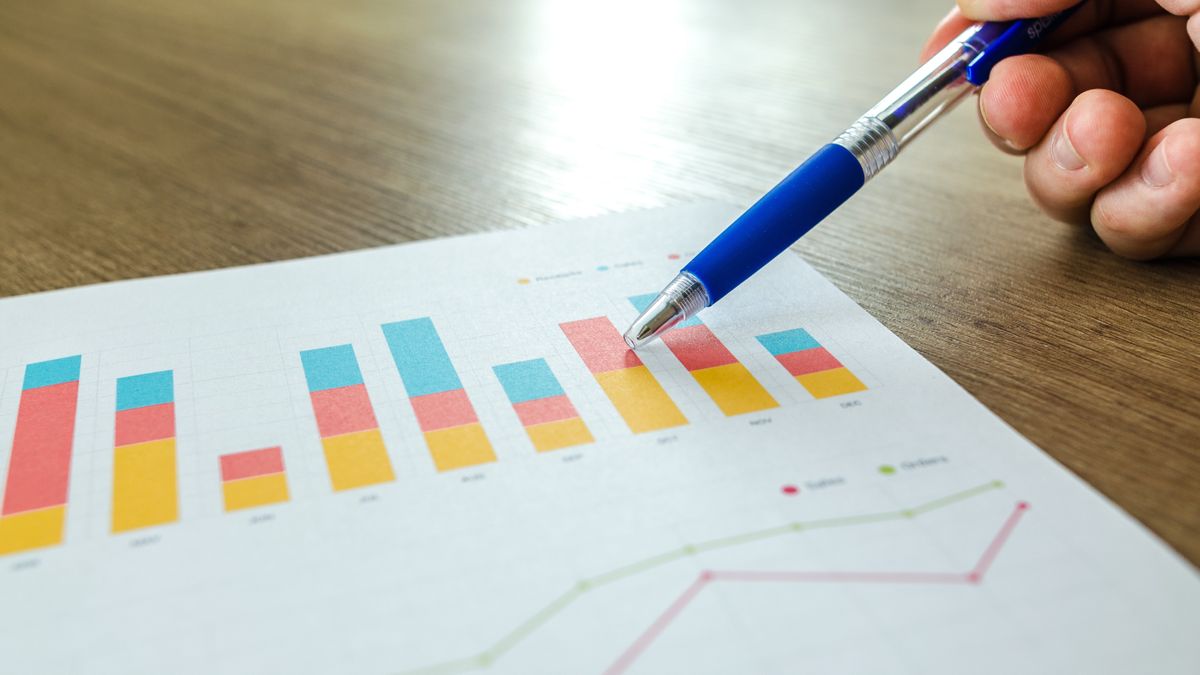
As enterprises strive for ways to better interpret data, they traditionally have relied on predictive analytics. However, as business becomes more competitive, organizations have realized they need to tap into even deeper insights and are beginning to look at the next level. By going beyond predictive analytics to leverage more advanced tools, such as prescriptive analytics, enterprises are able to leverage recommended actions that address uncovered insights.
- We've also highlighted the best cloud databases
What is traditional analytics and what is advanced analytics? Is there a natural progression toward advanced or are they different deployments?
The promise of analytics has always been about freedom of information and knowledge. The challenge has been how to glean meaningful knowledge from data and present it to an end user in a way they can understand and use to make critical business decisions in a timely manner.
Traditional analytics has focused on providing humans with tools to assist us with understanding data so we can become the interpreters of data. Human minds are very bad at detecting trends and patterns from raw data, so we create tools that give us the ability to manipulate data into visual constructs that the brain can better interpret. The next level, advanced analytics, gives us new solutions with advanced statistical techniques to uncover patterns in the data and serve up results to the end, rather than relying on humans to build visualizations (which can be subject to bias).
There is a natural progression towards advanced analytics – it is a journey that does not have to be on separate deployments. In fact, it is enhanced by having it on the same deployment, and embedding it in a platform that brings together data visualization, planning, insight, and steering/oversight functions.
Business analysts who start this journey will need advanced analytics features to be easy to use and accessible in their data visualization or planning tools at the click of a button. This allows enterprises to take the first step towards advanced analytics without needing significant retraining or upskilling. Users just need to know what kind of advanced analytics problem they are trying to solve and then the software should help solve it and provide the working model.
What is the main differentiator of predictive analytics, machine learning and prescriptive analytics?
This can be viewed as part of a continuum – predictive analytics is about the application of mathematics to data to uncover patterns and relationships. The question becomes “who is building these mathematical models?”
In the case of predictive analytics, there is a human or team of humans that are explicitly building these models. Machine learning is about removing the human element from the model creation so that software can build the models on behalf of the end user, and wherever possible, automate all other steps in the advanced analytics process.
Are you a pro? Subscribe to our newsletter
Sign up to the TechRadar Pro newsletter to get all the top news, opinion, features and guidance your business needs to succeed!
Finally, prescriptive analytics is about taking the results of those models and making a recommended action for addressing the uncovered insights. Prescriptive analytics further removes the human element from the decision-making process and instead allows software to identify and suggest the next course of action.
Are they used in different business processes or is one more advanced than the other?
They are used in the same business process, and enable users to automate at significant scale. However, prescriptive analytics makes possible the pursuit of new business processes and those are definitely more advanced than what a user can do with traditional analytics or even predictive analytics on its own.
For example, if someone can automate the end to end decision making process with prescriptive analytics based on live data coming from sensors in the manufacturing plant, why not take that one step further? Use the software to automatically take action and record the results of those actions without human assistance. The human effort is then focused on a higher level, ensuring process execution is effective in the aggregate.
Are businesses currently utilizing both or just one?
Business are using a mix of both to varying degrees of maturity. Some applications have embedded predictive models developed with machine learning techniques, which expose the results directly in the application and deliver suggested recommendations for the next action to take. These sit side by side with the workflow driven approach that would be more common in an organization.
What are the biggest barriers to successfully leveraging predictive analytics across an organization?
The biggest barrier has been a lack of human resources capable of building predictive analytics in a usable timeframe. In many ways, this is similar to what the traditional analytics market experienced. Data was locked away in databases and only those database administrators who could code structured query language were able to access it. Because of that shortage, and the importance of that information, tools became available to make it possible for a non-technical person to access that data and create meaningful visualizations.
What are the challenges of prescriptive analytics and how are some businesses preparing to offset those?
The most obvious challenge with regards to prescriptive analytics is governance. If you are removing humans from the decision-making process and your model is flawed (either because the situation has changed, or because you have outlier situations), then you will not only make mistakes, but you will make them automatically and at scale. In areas of sensitivity or human risk, it is becoming common to enable a human with the insights and benefits of prescriptive analytics, but ultimately the human makes the final decision.
How can companies prepare to go from predictive analytics to prescriptive?
Companies can prepare for this by changing the way they frame traditional analytics questions. Instead of thinking only about how to get a result to answer a business question, companies need to think about the new types of business questions they can ask. In other words, if you could look at historical patterns over time and project what is likely to occur in future periods, where would you apply this? Next, empower business analysts to actually solve these problems with tools that give them the ability to easily use machine learning techniques to develop the models.
David Judge, Vice President of Leonardo | Analytics at SAP
David Judge is the Vice President of Leonardo at SAP. He is currently focused on driving strategy and market awareness for SAP Intelligent Enterprise automation technologies including Intelligent Process Automation, Conversational AI, and embedded Machine Learning.