Why computational video is the next big battleground for smartphone cameras
Computational video poses a fresh set of challenges
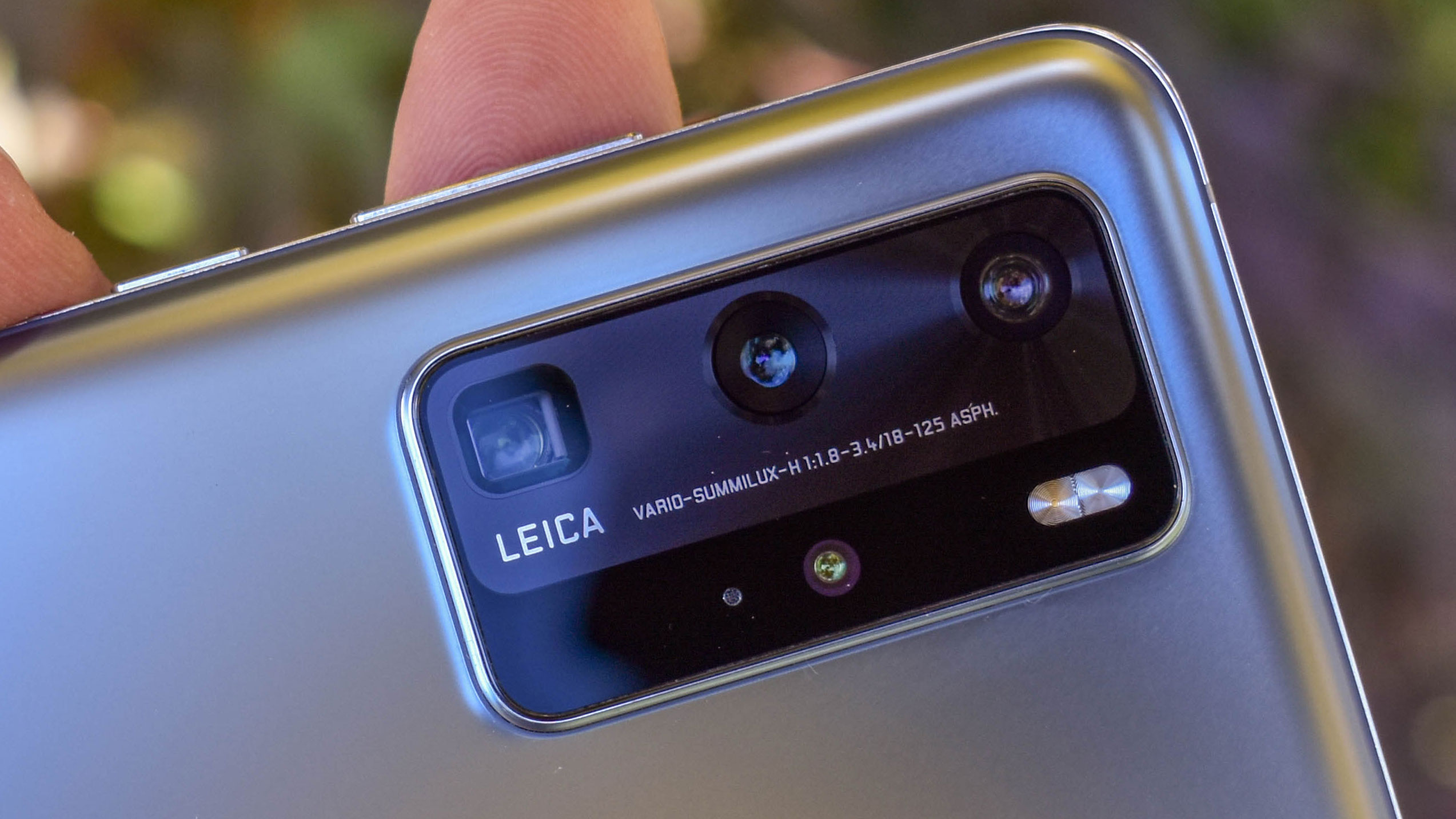
Computational photography has transformed how we take pictures with our phones. You may not have noticed, though, because the final effect is we simply no longer need to think about the limitations of phone cameras, and whether a particular scene is too much for them to handle.
When we look back to the early years of smartphones, what we used to think represented a "good" phone camera seems almost comical today.
Overexposed parts of pictures appeared more often than not, and even the best cameras took dismal low-light pictures compared to an affordable mid-range smartphone in 2022.
It's all down to radical dynamic range enhancement and multi-exposure night modes, powered by computational techniques. They often let phones with camera sensors the size of a baby's fingernail produce results comparable with a DSLR image, after it has been edited and fine-tuned in Photoshop too.
The key scene types that benefit the most are low-light environments and those with ultra-high contrast, where in the old days parts of the picture would be too bright or dim. Sunsets, for example.
Waiting for the computational video revolution
However, until now we have not really had the same experience in video. High-end phones are exceptional for stills, but most fall down when capturing video in trickier lighting. The great photo experience only highlights the issue. It's what computational video is here to solve.
Computational video poses a fresh set of challenges, because time is not on our side. A phone can take several seconds work out how a still image from its camera should look, construct it out of a dozen separate exposures and spend significant time putting them together.
Get daily insight, inspiration and deals in your inbox
Sign up for breaking news, reviews, opinion, top tech deals, and more.
We don't have that luxury with video, where each second of footage might contain 30 or 60 separate images per second. A phone can't make each frame of a video out of multiple exposures because there simply is no time to capture them, let alone process them.
Finding a fresh set of solutions for computational video is a top goal for every major manufacturer of higher-end phones at present. And this has become one of the big jobs for NPU processors, which are scaling up in power faster than any other part of phones at the moment.
The tech behind the software
An NPU is a neural processing unit, which handles machine learning and "AI" workloads. When they first started appearing, we though they would be used to power augmented reality experiences, like games where play pieces are rendered into the view of the real world seen by the camera. They are, but it turns out the ways AI, machine learning and neural processing can be used for photography are actually far more important.
The specific strength of these neural processors is they can handle a huge number of instructions in a short window of time while using very little power. As we've already discovered, this is exactly what we need for computational video.
However, that is just the resource. What can phone-makers do with it and which techniques can make computational video as strong as stills? Let's look at some of the techniques they can use.
A set of techniques
Stripping right back to the essentials we have 2DNR and 3DNR, two dimensional and three-dimensional noise reduction. This is the process of separating detail from noise, in an attempt to smooth out noise without reducing genuine visual information in the picture.
All cameras use noise reduction already, but greater neural processing power lets new phones employ more advanced NR algorithms to do the job more effectively.
What's the difference between 2D and 3D NR? In 2D noise reduction you analyze a frame on its own. You may bring to bear machine learning techniques informed by millions of similar-looking pictures others have taken, but each frame is effectively treated as a still picture. Pixels are compared to nearby clusters of pixels to identify and zap image noise.
The additional dimension added by 3DNR is not depth but time. Noise reduction is based on what appears in successive frames, not just the image data from a single one.
Computational video's task is to make both types of noise reduction happen, at the same time, but using the right technique in the correct parts of the scene. 3DNR works beautifully for relatively still areas of the image.
When shooting in low light, the high sensitivity levels required can make the picture appear to fizz with noise. Temporal 3D noise reduction gives a phone a much better chance of keeping genuine detail without making it appear to zap in and out of existence frame-to-frame.
However, 3DNR is not a great solution for moving objects, because you end up trying to compare sets of fundamentally different image data. The phone needs to separate parts of the image in motion, still areas, apply different forms of processing and be ready to change those areas from half-second to half-second.
And, of course, the intensity of the processing also has to switch gears as the light level changes mid-clip.
Going to the source
We also need the phone to capture better data, to generate less noise in the first instance. How do we do that without just employing a larger sensor with higher native-light sensitivity?
Better use of optical stabilization (OIS) is one route. In phones this typically involves a motor that moves the lens slightly to compensate for any motion in the user's hands, although sensor shift stabilization in phones now exists too. The latter is a core feature of high-end mirrorless cameras.
This motion compensation lets a phone use a slower shutter speed while avoiding blurred image data. When filming at night, the longer the exposure, the better data a phone camera has to construct a frame. And when shooting at 30fps, the maximum theoretical window is 1/30 of a second, obviously.
Computational video can make dynamic use of this concept of the maximum exposure window, with the help of lens-stabilizing OIS.
In some low-light situations a phone will benefit from reducing the frame rate to 30fps even when you selected 60fps capture. This doubles the maximum exposure time, letting the camera capture frames with greater detail and less noise.
The "missing" frames can then be generated through interpolation, where interstitial images are generated by looking at the difference in image data between the frames we do have. This may sound like heresy to traditional photographers, but it gets to the heart of what a computational approach to imaging is all about.
The results matter. How you get there matters less.
Why stop at 30fps? A phone could even drop to a much lower frame rate like 15fps and still create a 60fps video, which could look great if the scene is reasonably still.
The lower the frame rate, the longer the maximum exposure window becomes. At that point we're talking about theoretical techniques, though. To our knowledge no phone goes that far yet.
Exposing problems
However, there is a problem. OIS, the tech we're relying on to make a slow shutter speed viable, can only compensate for motion on one end. It can avoid handshake blur, not the motion blur of someone running across the frame.
Just as we saw with noise reduction, the best computational solution may change from moment to moment depending on what's happening in the frame. One of computational video's roles is dealing with this, varying the rate of capture on the fly.
There is also a hardware technique that can help, called DOL-HDR. You may well be familiar with "normal" HDR modes for stills. It's where several frames are collated to make one picture. In the phone world this could mean anything from three to 36 images.
With video there's no time for this, and minimal time to account for the subtle changes that happened in the scene as those exposures were captured — which causes an effect called ghosting in poorly handled HDR modes. DOL-HDR avoids these issues by taking two pictures at the same time, using a single camera sensor.
How? The data from a typical camera sensor is read line by line, like a printer head working its way across a piece of paper. One row of pixels follows the next.
DOL-HDR records two versions of each line at a time, one of a longer exposure image, another of a shorter exposure image. This kind of HDR can be put to great use in scenes where there's masses of contrast in the light level, such as a sunset.
However, if it can be used to capture sets of video frames with different shutter speeds, rather than just differing sensitivity settings (ISO), DOL-HDR can also be used to maximize the motion detail and dynamic range of night video.
Picture the scene we mentioned earlier. We are shooting a relatively still low-light video, but a person runs through the frame and we don't want them to appear a motion-blurred mess.
With DOL-HDR, we could use the short exposure to get a sharper view of our moving figure, the longer exposure to yield better results for the result of the scene. The "HDR" in DOL-HDR may stand for high dynamic range, but it can be useful in other ways.
Computational video's job is to cycle seamlessly between countless different shooting style and techniques, and take on their ever-increasing processing burden.
The ones we have outlined are likely just a few of the ones phone-makers will employ too. The question now is which phones will handle computational video best in 2022 and beyond?
Andrew is a freelance journalist and has been writing and editing for some of the UK's top tech and lifestyle publications including TrustedReviews, Stuff, T3, TechRadar, Lifehacker and others.