The lake and the stream: analogies to assist your big data strategy
Understanding the storage and flow of data
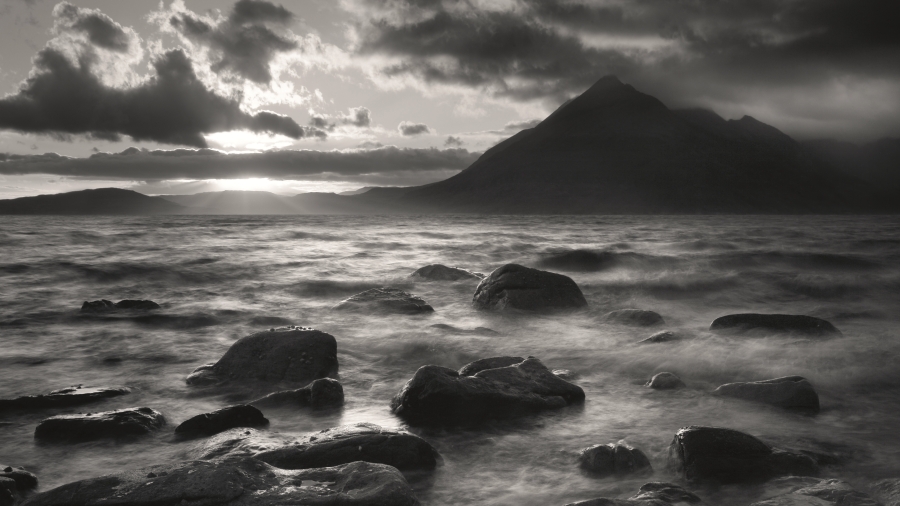
'Data lakes' and 'data streams' are becoming increasingly common analogies in the discussion on big data strategies. As in nature, both lakes and streams have their individual characteristics and are each important to the overall ecosystem. The question is: how do they relate to each other in the context of big data, and how can we best use them for better business outcomes?
Data lakes represent large pools of data that have accumulated over time. In the same way that man-made lakes are formed by the construction of a dam to store water for later use, data lakes are formed by a deluge of information being diverted into a large repository, where it can be held for a long period of time until needed.
Lakes by their very nature are dependent on being fed by new water flowing into them, to keep the environment vibrant, otherwise the lake could stagnate. Similarly, data lakes must be constantly enriched by current flows of information, in order to assure that the overall data set remains relevant.
However, this means that the storage capacity of the lake must be continually expanded to accommodate all of the new data being added to it. This presents a tough challenge – namely how best to analyse these vast bodies of information meaningfully, without getting bogged down in irrelevant data.
Casting a wider net
One way of thinking about meaningful analysis from a data lake is like fishing for a particular type of fish. If you only use a single fishing rod, then your chances of catching that one specific fish is small, unless you spend a significant amount of time and effort.
But by using a wide net, you can increase your chances, by covering a larger area at once. However, bound up with any catch, there is a high probability of a lot of extraneous material along with the data that is most relevant, so you have to spend even more time sorting through the insights.
In both cases, the fact that you are only fishing in one area means that you could miss new input that may be flowing into a different area. As such, there is a strong possibility of missing new data or information that could have changed the analysis.
Are you a pro? Subscribe to our newsletter
Sign up to the TechRadar Pro newsletter to get all the top news, opinion, features and guidance your business needs to succeed!
This is not to say that data lakes are not useful, just that their use must be tailored to their characteristics. As a result of their vast nature, data lakes are best used in situations where a lot of historical perspective is required, such as in cases where trends need to be examined over a longer period of time.
The stream analogy
Data streams on the other hand, involve a fundamentally different approach to analysis than data lakes.
As data streams are constantly flowing, analysis has to take place in real- or near-real time, circumventing the lake altogether. As such, the analogy here is that working in data streams is much like panning for gold. As the data stream passes by, analysis occurs in parallel, seeking to capture the relevant nuggets of information to best address specific questions or areas of concern as they happen.
The main advantage of this approach is that information can be accessed quickly and insights can be pulled out rapidly. Given the fast-paced and dynamic nature of modern business environments, it is imperative that anomalies or real time trends can be understood quickly, so that appropriate action can be taken before they have a significant impact on business processes or revenue.
Data stream analysis is the most effective solution to manage in this challenging real-time environment.
However, it can be difficult to take advantage of data streams and extract the most valuable overall insights from the torrent of data; streams often flow very quickly and are composed of many different elements, to the extent that many operations fail to deliver meaningful real-time analytics.
Fine-tuning analytics operations
With so many big data players pouring into the market, it's more important than ever to closely evaluate all the different approaches to assess who has invested appropriately in order to truly manage data stream analysis.
An effective system for data stream analysis must be able to handle billions of transactions on a consistent basis, whilst being able to analyse several streams simultaneously. By combining information from a number of sources, analytics teams can form a full, high-value perspective on the situation, rather than a single isolated viewpoint.
Finally, taking maximum advantage of the data stream requires more than just being able to handle the fast running flow of information. The analysis methodology must be able to pick out the most relevant data points for the business situation. This equates to creating the right type of 'sieve' that can quickly pull out the proper pieces of data and discard the mass of other material that is extraneous.
The art and science of performing this type of analysis requires a very thorough understanding of the business environment, intersected with the complexities of data science. This is a unique set of capabilities, but without this, the gold will not be extracted.
Appreciate the differences
Data lakes and data streams are both very valid approaches to big data analysis. However, they are both very different, and are best applied in different situations to extract the most value.
Analysing data lakes is most appropriate when broad, long-term historical perspectives and trends are required. On the other hand, data stream analysis is best suited when real-time analysis is required, such as dealing with customer complaints.
With this difference in mind, enterprises can appropriately devise their big data strategies based on their immediate and long-term business needs.
- Rob Chimsky is VP of Insights at Guavus and has over 30 years in the telecommunications industry.