Neural nets clean up music libraries
Ballroom-trained software can accurately classify music by genre
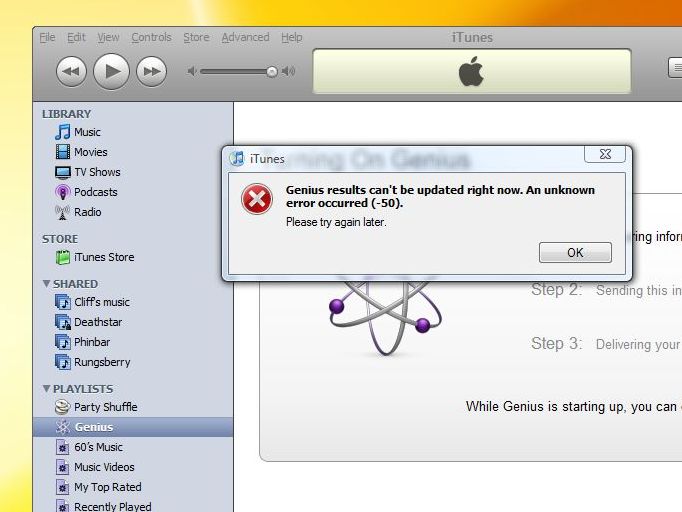
Is M.I.A. pop, electronic or hip-hop?
Getting your digital library organised can be a nightmare - and it doesn't help that some record companies forget to add genre tags to MP3 files altogether.
Now computer scientists in Taiwan have devised a neural network program that can automatically classify computerised music files based on their beat and tempo.
Computerised critic
Chang-Biau Yang of National Sun Yat-sen University plays the music file to his neural network, which analyses the beat and tempo using two main approaches to classifying music - the Ellis and the Dixon methods - named for their inventors. The software then outputs a general musical genre.
In this initial learning phase, the researchers correct the misses and feed the hits back into the neural network so that it builds up an audio profile of how different music files sound in each different genre. Once the neural network has been trained it can then classify a whole collection of music files.
The next step is then to use the Ellis and Dixon methods to further confirm the genre of each neurally classified group of music files. These methods using different signal processing approaches to listen to the music file and to determine the position of peaks that correspond to the musical beat. They can be used to estimate tempo and beat pattern.
Get daily insight, inspiration and deals in your inbox
Sign up for breaking news, reviews, opinion, top tech deals, and more.
The Taiwanese team has tested their approach on a collection of several hundred ballroom dance music files. Their system has classified different music styles, such as cha-cha-cha, jive, quickstep, and tango, with varying degrees of success. Yang suggests that further training of the neural network with classical, jazz and pop music files will allow it to classify more diverse music collections automatically.