Data products and how they can drive greater business efficiency
Businesses need to adopt data product, not project mindset
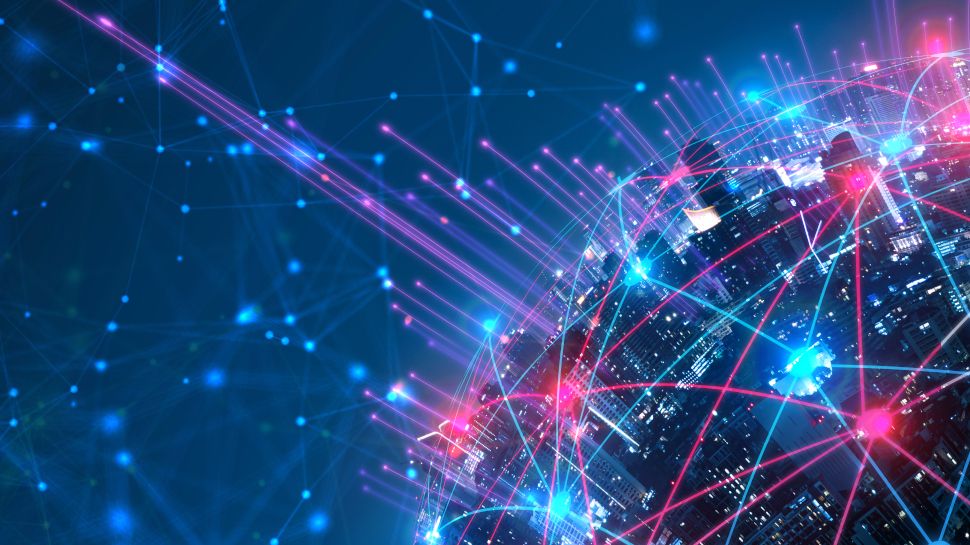
Data is the fuel powering everything we do and has the potential to be a business’s greatest asset. That’s hardly a new line of industry thought, yet it remains solid and backed firmly by calculated forecasts: for example, it’s widely estimated there will be 175 zettabytes of data in the global datasphere by 2025. This equates to every person on the planet producing at least 1.7 MB of data every second for the next two years.
From humans, machines and Internet of Things (IoT) devices to edge systems and beyond, organizations are sitting on a mountain of data. It is everywhere, and its value to businesses is undisputedly essential in enabling them to thrive in today’s fast-paced world.
Enterprises have the opportunity to turn data into a major competitive differentiator. However, the way in which many are currently approaching data is suboptimal.
Most companies continue to see data with a project mindset. Each time they encounter a problem that it wants to solve with data, a new initiative is launched to acquire that data, cleanse it, prepare it, and then analyze it for a specific use case.
What is a newer line of industry thought however – and unfortunately so – is that this approach has several drawbacks. It’s slow, leads to duplicated work, and the outputs from each project can’t typically be repurposed to solve other use cases.
What do we actually mean by ‘data products’?
Instead, organizations should be looking to manage data like a product, shifting focus away from individual challenges and towards those solutions capable of solving them on a repeat basis. In other words, they should embrace a product (not project) centric approach to data.
In simple terms, a data product delivers a high-quality, ready-to-use set of data that people across an organization can easily access and apply to different business challenges.
Typically, data products are built on scalable platforms by teams that have skills across the entire data lifecycle. Comprising three key components – data, public cloud technology, and custom developed business logic – they provide an individual with everything they need to understand and use data to solve a problem.
Critically, they’re designed with the entire organization's data needs in mind. Unlike data projects, data products can be repurposed to support numerous use cases across multiple functions.
It is also worth noting that they can come in a variety of asset types – reusable data sets, machine learning models and dashboard reports are all forms of data products, for example.
Sean Poulley is COO at Keepler Data Tech.
Striving for data-driven intelligence
The desire for businesses to make decisions based on data and become data driven has been around for decades, whereas data products as a solution may seem like a relatively new concept. But they are very much already in existence – we just weren’t aware. There are simple examples literally in the palms of our hands, such as Google Maps. They collect data on different routes you can take to get from A to B and provide optimized answers for time, or cost to reach your destination. Another example would be ChatBots like ChatGPT (applications that answer your simple questions in online chat) in customer service that reduce costs and time to respond. A final example might be Expedia who have transformed the experience and time to book travel. Expedia continuously assesses changing flight and hotel prices and their availability to offer suggested best combinations for our needs.
So what has changed in the last 10 years? The evolution of public cloud, enabling a step function in the creation of largely unstructured data – be it files, video or web information, or IoT data – that is now stored on a previously unimaginable scale. Not only that, but public cloud has also made it much more cost effective to analyze vast quantities of data in near real-time thanks to dramatic improvements in scalability and performance.
Resultantly, the groundwork has been laid for the data product concept to evolve significantly in the last three to four years as firms seek to integrate analytics into business processes and become more intelligent. And we’ve seen firms that have been successful in developing data products reaping the rewards.
When organizations manage data like a consumer product, they’re able to realize the near-term value of their data investments and pave the way for quickly getting more value tomorrow. It facilitates the democratization of data, meaning teams using data products don’t have to waste time searching for data, processing it into the right format, and building bespoke data sets.
Time to insight is dramatically reduced where data products have been pre-built and, typically, are backed by well-defined interfaces with an emphasis on UX, while users can also reliably trust these models in the knowledge that they will have undergone rigorous testing.
The difference between insight and instinct
Indeed, organizations taking a product approach to data become empowered to improve decision-making, boost efficiency and achieve lower cost-per-use than data projects, all while unlocking new revenue streams as they become increasingly data driven.
Critically, these benefits are not exclusive to any specific company, industry, or region. In the oil and gas sector, we’ve seen data products used to facilitate dynamic gas pricing, enabling one firm to increase gas prices without reducing demand, for example. Meanwhile, we’ve also witnessed a utilities company successfully deploy data products to achieve a 60% reduction in the time taken to detect and solve network anomalies.
The true value of data lies in the ability of enterprises to interpret data and drive intelligent decision-making in this way: those businesses that have greater knowledge of their customers and products can improve the services they offer and differentiate themselves from competition.
This is precisely the edge that data products offer – they are often the difference between companies making changes based on informed insights versus misinformed instincts.
Transforming a data culture
So, how exactly can an organization shift from a culture of data projects to data products?
There’s no question that many firms need to advance their capabilities on several fronts. They will need to work on improving data quality and eliminating inconsistent data, developing more robust and reliable models, and increasing big data competencies to manage increasingly demanding processes, for example.
Further, firms should work to implement best practices in the form of regulation and commitments to ensure that technologies are applied as transparently, ethically and fairly as possible. This means building datasets that have sound representation, checking for bias by defining metrics across different subgroups and conducting model sensitivity analyses.
With that said, however, these things will likely only be achievable if companies first secure, implement and leverage the right skillsets, technology strategies, and partnerships that will propel them soundly into a space that’s relatively new for them. For any data product strategy to be successful, there must also be a combined investment into training from companies, and a personal effort made by individuals to learn and embrace new skills.
Without question, vital efforts like this will help to form the foundations from which effective data products – capable of empowering organizations of all shapes and sizes to tap into data and drive intelligent decision making – can be built.
Are you a pro? Subscribe to our newsletter
Sign up to the TechRadar Pro newsletter to get all the top news, opinion, features and guidance your business needs to succeed!
Sean Poulley is COO at Keepler Data Tech.