AI Agents: why your enterprise needs a semantic layer for true intelligence
How AI agents can transform raw data into strategic foresight
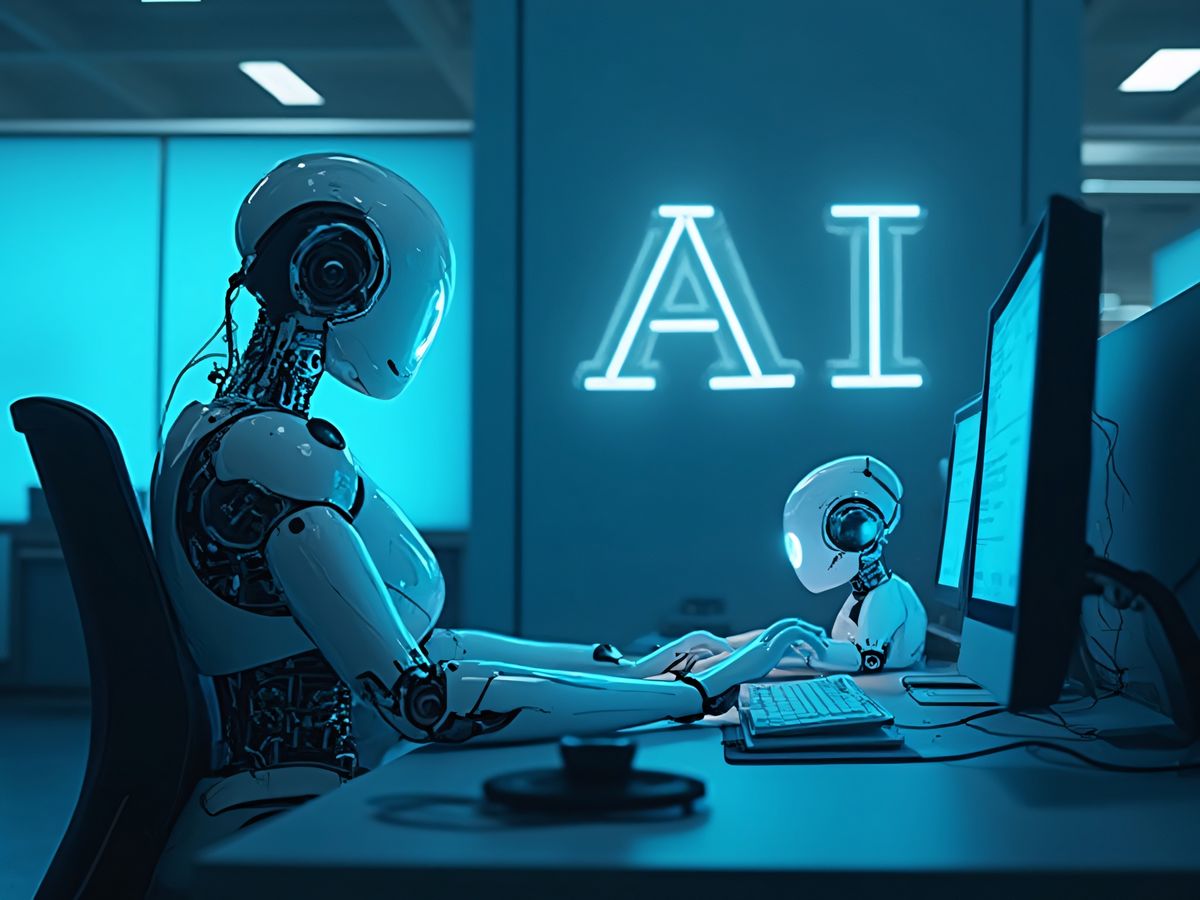
In AI, yesterday’s breakthrough is today’s baseline, so AI agents that simply retrieve information are no longer enough. The real game-changer lies in creating AI agents that truly understand your business context and can transform raw data into strategic foresight.
The key to unlocking this potential lies in the sophisticated interplay between AI agents and semantic layers. This turns data complexity into business opportunities.
VP of Sales Engineering, App Orchid.
Understanding the Semantic Layer: Translating Complexity into Clarity
Think of a semantic layer as the interpreter for your fitness watch's data. While the watch itself collects raw sensor data, like heart rate, steps, and sleep cycles, the semantic layer organizes this data and defines relationships, calculations, and hierarchies that make it easier for you to understand. It doesn’t generate insights automatically but instead provides a structured framework that allows you to explore and interpret your data meaningfully.
In business, a semantic layer acts as an intermediary between raw data and end users. It connects analytics platforms with data sources by organizing facts (data values), dimensions (attributes), and hierarchies (taxonomies). This creates a consistent, business-friendly view of the data, so anyone in your organization can access and analyze it without needing technical expertise.
For example, a semantic layer standardizes the relationship between customer satisfaction metrics and operational factors like response times and staffing levels. This framework enables users to connect the dots to derive meaningful insights themselves.
By ensuring consistency and accessibility, a semantic layer transforms raw data into a structured, interpretable resource. This makes it possible for your teams to focus on decisions rather than deciphering data.
AI Agents: The Personal Trainers of the Digital World
If the semantic layer is the framework that organizes and structures your data, the AI agent is like having a personal fitness coach who helps you act on that data. While the semantic layer makes information accessible and consistent, the AI agent leverages that data to understand your goals, track progress, and provide recommendations based on patterns and performance.
In business terms, an AI agent goes beyond retrieving information, it uses machine learning and contextual understanding to provide strategically aligned recommendations. It interprets data through the lens of your organization’s objectives and adapts its responses based on interactions and evolving patterns.
Just as your fitness coach might notice you perform better with afternoon workouts and suggest adjusting your schedule, an AI agent learns from your business’s unique data estate, workflows and preferences. It identifies trends, highlights key insights, and suggests optimal actions to achieve your goals.
Unlike the semantic layer, which organizes and connects the data, the AI agent drives action by interpreting that data and delivering insights in a way that aligns with your needs, empowering better decisions across your organization.
The Core Components of Modern Intelligence
1. Ontology (The Foundation)
Your fitness watch knows that sleep quality affects recovery time, which influences exercise recommendations. Similarly, a modern ontology maps the relationships between different aspects of your business data. This ensures every insight considers the full context of your business operations.
The ontology enables rich data modeling and connections through its core features. It supports:
Hierarchical traits (Effectively represents structures such as organizational hierarchies and relationships between entities)
Causal relationships (Provides tools to infer or represent relationships where one metric or event may influence another, often via advanced configuration or integration with data models)
Temporal traits (Handles data associated with time through traits like temporal, periodic, and sporadic events, allowing nuanced tracking and pattern recognition over time)
Domain-specific ontologies (Enable domain-specific modeling, leveraging data enrichment, traits, and linguistic features to capture industry-specific standards and practices, though it may require additional configuration to reflect regulatory or operational nuances)
2. Linguistic Models (The Interpreter)
Just as your fitness watch understands different types of activities, from "running" to "high-intensity interval training," cutting edge linguistic engines comprehend various ways users might ask for information. This sophistication can be demonstrated by two key benchmarks:
Industry standard or above accuracy on the Spider benchmark, a widely recognized and respected test for evaluating Text-to-SQL translation. Spider evaluates an AI's ability to convert natural language questions into accurate SQL queries across complex scenarios involving multiple tables and database relations. This benchmark tests "zero-shot" generalization, meaning the system must handle queries about databases it has never seen before, just as it does in real-world business scenarios.
Recent research from 2023 showed that leveraging Knowledge Graphs with LLM-powered question-answering systems boosted SQL query accuracy by an additional 54%, highlighting how semantic understanding enhances these already impressive results.
Successful linguistic models excel at:
- Understanding industry-specific terminology
- Recognizing contextual meaning
- Handling natural language variations
- Adapting to user preferences and patterns
3. Transformational Logic (The Rules Engine)
Like the algorithms that determine your fitness zones based on age, activity level, and health goals, transformational logic ensures data is processed consistently according to your business rules and industry standards. This component:
- Applies business rules consistently
- Ensures compliance with industry regulations
- Maintains data quality standards
- Enables personalized insights based on user roles
From Data to Strategic Intelligence
This sophisticated architecture enables transformative platforms of today and into tomorrow to deliver three key capabilities that transform how organizations leverage their data assets:
Adaptable Knowledge Architecture
Much like a living organism that thrives through interaction with its environment, adaptable knowledge architecture evolves alongside your business with a human-in-the-loop approach. It integrates information and empowers your team to refine and enhance relationships, patterns, and business contexts, ensuring the system continually aligns with your organization’s unique needs.
This type of collaborative model creates a progressively more sophisticated understanding of your organization’s ecosystem while keeping human expertise at the core. This creates a unified intelligence layer across your organization that evolves with your business through guided human input and updates, revealing multi-dimensional relationships across data sources
Cognitive Augmentation
Going beyond traditional analytics, cognitive augmentation acts as an intelligent partner in your decision-making process. It combines machine learning capabilities with deep semantic understanding to identify patterns that might be invisible to human analysts, while providing clear explanations of its insights in business terms your team can understand and act upon. This uncovers non-obvious patterns and correlations, provides narrative insights explaining the 'why' behind trends, and suggests optimal actions based on comprehensive analysis
Real-time Strategic Value
The ability to move quickly from insight to action is crucial. Modern technology doesn't just process data faster, it fundamentally transforms how quickly organizations can identify opportunities, understand implications, and take decisive action based on comprehensive understanding of their business context. This accelerates insight-to-action cycles. Enables proactive decision-making and facilitates innovation through pattern recognition
Conclusion
In an era where data availability often exceeds our ability to derive meaningful insights, the combination of sophisticated AI agents and semantic layers provides the key to unlocking true business value. Innovative technology empowering businesses in today’s world can transform this potential into reality, turning your data into a strategic asset that drives business success.
The question isn't whether to enhance your AI capabilities, but how quickly you can leverage these advanced tools to gain competitive advantages. Innovations that provide the foundation for this transformation will successfully turn data complexity into strategic opportunity.
We've featured the best business intelligence platform and the best data visualization tool.
This article was produced as part of TechRadarPro's Expert Insights channel where we feature the best and brightest minds in the technology industry today. The views expressed here are those of the author and are not necessarily those of TechRadarPro or Future plc. If you are interested in contributing find out more here: https://www.techradar.com/news/submit-your-story-to-techradar-pro
Are you a pro? Subscribe to our newsletter
Sign up to the TechRadar Pro newsletter to get all the top news, opinion, features and guidance your business needs to succeed!
VP of Sales Engineering, App Orchid.
You must confirm your public display name before commenting
Please logout and then login again, you will then be prompted to enter your display name.