AI hype is real in manufacturing – successful adoption journeys are scarce
AI success is dependent on the operational approach taken
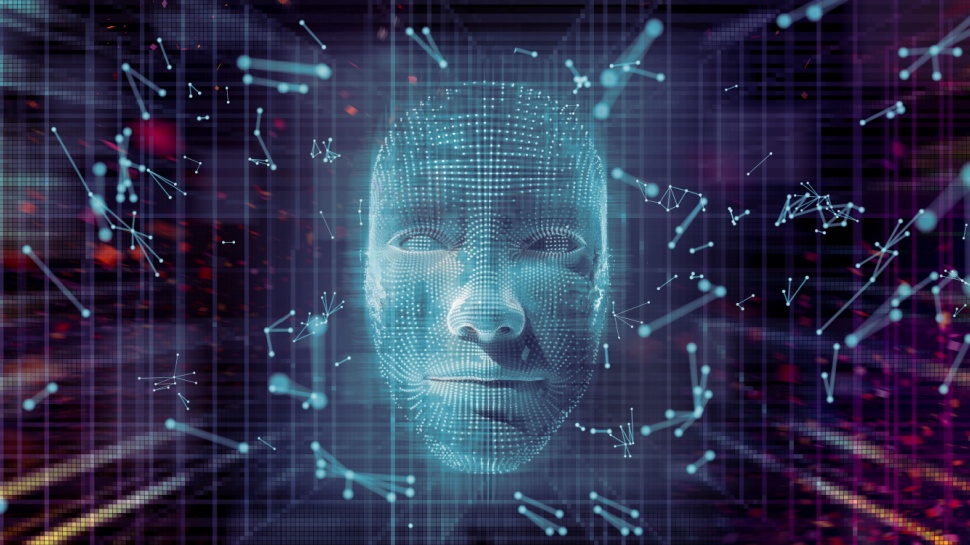
How can manufacturers ensure a smooth integration?
Pressure from customers to produce highly complex and good quality products quickly is mounting on manufacturers. AI may seem the answer, but when nearly 80% of AI projects fail to reach targets or get completed – is it really the solution to manufacturing problems? For Nicholas Lea-Trengrouse, Head of Business Intelligence at Columbus, success is dependent on the operational approach taken. AI projects must deliver value at every level to be a success. So how can manufacturers achieve this result?
AI experiments are a thing of the past. AI and machine learning are on the rise with over 85% of UK manufacturers already invested and wanting to incorporate AI tools full-time into their operations. UK manufacturers are primarily using these technologies in four key areas: quality control (38%), cybersecurity (37%), logistics (34%), and customer service (32%).
The AI hype is here but on the factory floor, the success of working adoption frameworks and journeys are limited. This is causing new adopters to be skeptical, but with the right product development plan manufacturers can smoothly adopt AI into all levels of their operations. Here’s how.
Head of Business Intelligence at Columbus.
1. Avoid stalling at the first phase
A recent Gartner AI survey revealed that only 54% of projects progress from the pilot phase to production. So, what’s behind this? Manufacturers often identify use cases for AI and conduct proof of concept or pilot projects, but these efforts frequently stall – a phenomenon known as pilot purgatory.
Gartner’s hype cycle for AI shows we are currently at the peak of inflated expectations. AI projects fail because they often overlook the anticipated value and the true implications of implementing the technology within the organization. So, how can manufacturers avoid falling into the AI void?
What’s the secret to AI success?
AI adoption success lies in a product development roadmap that helps organizations scale effectively. The key is to aim for early wins by identifying business areas already primed for AI success and where significant impact is possible.
Sign up to the TechRadar Pro newsletter to get all the top news, opinion, features and guidance your business needs to succeed!
Consider this scenario: a manufacturer aims to increase its profit margins by 10% next year. Achieving this goal depends on meeting three objectives: reducing machine downtime, minimizing wastage, and addressing supplier irregularities.
From this assessment, the manufacturer can pinpoint opportunities to use AI analytics to predict machine failures, detect product quality issues, and optimize supplier routes. The focus is on understanding which processes can be transformed to successfully adopt AI and create new value, which will benefit both the bottom line and the workforce.
2. Finding the right data and insight balance is crucial in avoiding analysis paralysis
One of the biggest challenges in AI adoption for manufacturers is data management. Organizations often collect data from multiple disparate sources, including Excel spreadsheets, manual entries, on-premise servers, and cloud-based systems. Manufacturers that attempt to integrate all this data to gain a comprehensive view of the business and train AI models are left with the significant hurdle of analysis paralysis.
To overcome this challenge, manufacturers need a robust data strategy that ensures seamless data integration and accessibility. This requires manufacturers to standardize data formats, implement centralized data storage solutions, and employ advanced data processing techniques. A unified data ecosystem allows organizations to improve data quality, streamline workflows, and enhance the accuracy of AI models.
Cut through the data clutter with robust data management
When it comes to AI use case scenarios, manufacturers have limited reference examples, so they must assess the data they have available and determine what additional data might be needed to train the AI tools. Organisations are inundated with data, but quality matters as much as quantity. To ensure data quality, manufacturers need to implement robust processes and policies for managing data correctly, ensuring its usability, consistency, and integrity.
This is where the outputs of machine learning models and associated decision-making data in end-to-end solutions can make a significant difference. These outputs can be integrated into dashboards tailored for everyday business use, seamlessly fitting into user workflows to provide actionable insights. Manufacturers can then use these insights to optimize operations, improve decision-making, and drive better business outcomes.
3. Employee pushback is to be expected – AI is there to empower not replace!
One of the initial hurdles manufacturers face with AI adoption is employee resistance. When businesses introduce changes, especially technological ones, the immediate concern for many employees is, “Will this take my job?” However, manufacturers aim to harness AI not to replace roles but to make staff more efficient, reduce repetitive tasks, and enhance overall productivity. So, what can be done to increase acceptance?
Strong leadership and communication can ensure a smooth adoption through the pilot stage and beyond
It’s time for leadership to step up and demonstrate how AI implementation will benefit everyone. The focus should be on how employees can use the technology to enhance job functions, improve working conditions, and create new opportunities for career growth rather than being replaced by it. Leadership teams need to align AI initiatives with business objectives, working backward from desired outcomes to identify applications that drive these goals. This product-based approach helps organizations understand what their people need from AI and how it will integrate into larger operational frameworks.
At this stage it is vital to involve employees in the AI implementation process to boost acceptance. Manufacturing companies can seek employee input and demonstrate how AI can address their concerns and make their tasks easier and more efficient to create a sense of ownership. Manufacturers can encourage cross-functional teams to work together and share insights in order to ensure a smoother AI integration process and alignment with organizational goals.
To ensure success beyond the initial pilot phase, leadership teams must evaluate AI products using more than just standard performance metrics. User adoption rates can provide valuable insights. By examining the proportion of the target audience actively using the product, the repeat usage rate, and how well AI helps employees resolve initial issues, manufacturers can assess whether both employees and the company are realizing the long-term value.
4. A growing data skills gap is holding AI implementation back – what’s the answer?
A third (33%) of UK manufacturers report that a lack of skills is the most significant barrier to implementing smart manufacturing technologies like AI. This highlights a substantial gap between the demand for these technologies and the ability to implement them effectively. So, how can manufacturers address this skill divide?
The skills are already at a manufacturer’s disposal they just need to realize it!
One approach is to invest in training programs to upskill current employees. IBM research found that reskilling and workforce development (39%) are among the top AI investments for organizations exploring or deploying AI. Many manufacturing organizations often don't realize they already have employees with the technical or transferable skills needed for AI roles. For instance, a logistics coordinator could pivot to being a data engineer or data analyst because they already understand the data, processes, ERP systems, and CRMs.
What’s often missing is a bit of targeted learning and support in areas such as data engineering, data lakehouses, data warehousing, or coding. With this additional training, manufacturers can transform their employees into data engineers and effectively use AI to augment and enhance their work.
Additionally, manufacturers can look to hire new talent with expertise in AI and smart manufacturing. A team built with a mix of experienced employees and new hires with fresh, specialized knowledge can create a dynamic workforce capable of driving technological innovation.
Organizations that are able to tap into the existing skills within their workforce and provide the necessary training to new hires, can more smoothly integrate AI technologies, improve operational efficiency, and ultimately enhance their competitiveness.
AI roadmaps are key to success
Manufactures that approach AI project with a two-pronged approach will see lasting success. First, they need to break down their adoption into manageable stages. Second, they will also need an AI roadmap which allows them to see where AI can add value, streamline operations, and improve production. Brought together, these two steps will help manufacturers gain success from implementation and avoid becoming another statistic of failed AI projects.
We've featured the best AI phone.
This article was produced as part of TechRadarPro's Expert Insights channel where we feature the best and brightest minds in the technology industry today. The views expressed here are those of the author and are not necessarily those of TechRadarPro or Future plc. If you are interested in contributing find out more here: https://www.techradar.com/news/submit-your-story-to-techradar-pro
Nicholas Lea-Trengrouse is Head of Business Intelligence at Columbus.