AI’s impact on the auto industry has only just scratched the surface
There's much for the automotive industry to learn from AI
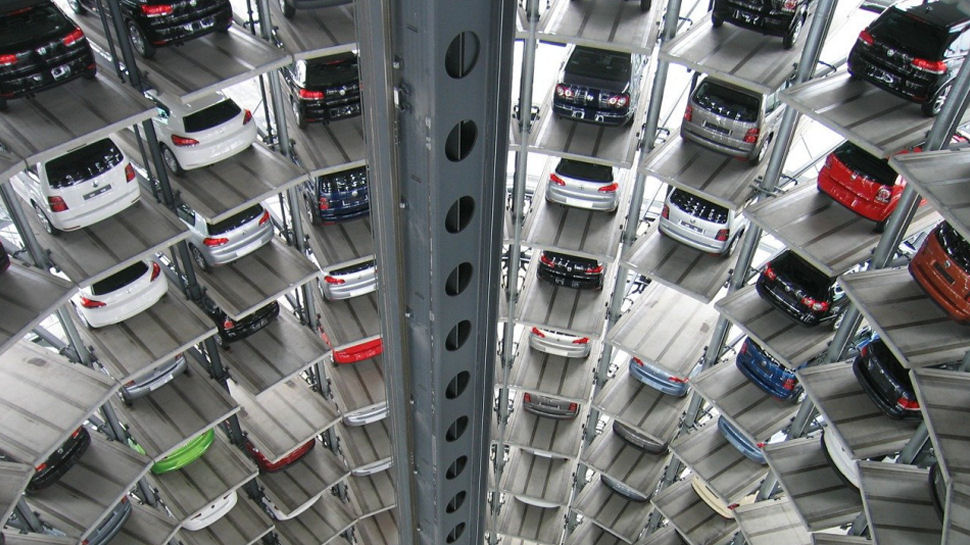
When it comes to Artificial Intelligence’s (AI) impact on the automotive sector, the key focus areas to date have been in the pursuit of innovations in self-driving cars and the improved efficiency of batteries. Whilst there has been progress in both these areas, the rise of the autonomous vehicle and growth of the electric vehicle (EV) market more broadly, has not materialized at the pace many had anticipated, even with the rise of AI.
Tesla’s most recent results and the poster child for the EV revolution shows the challenges the market faces, missing Bloomberg analysts’ average sales estimate by the biggest margin ever. Apple recently announced the abandonment of “Project Titan”, its decade long electric vehicle project, further proof that even one of the world’s most valuable companies cannot pivot into the notoriously competitive automotive sector. The fact that Apple plans to shift the funding towards building AI, is a sign of things to come.
The slowdown in global EV sales might paint a bleak picture as governments aim to meet their net zero targets and the automotive industry aims to phase out the internal combustion engine, but there are positive technological developments in the works that will help the automotive industry clear their current hurdles.
CTO at Monumo.
The impact of AI
Take a step back and it's clear to see the impact AI has had on society more broadly, in such a short space of time, with the likes of picture generation ((Dalle-2) and text (ChatGPT) showing us that AI systems are here, and learning quickly. These great AI advances, which use Large Language Models (LLMs) have progressed, because there was plenty of good quality, readily available data to train those models. When we look at the limits of AI’s impact on the automotive sector, it's because we are not looking beyond LLMs for the answer.
For example, Porsche currently has, what they call, the “Recommendation Engine”, which uses AI to determine unique and suitable options for a customer’s car, from billions of possible combinations. Add to this, that every leading manufacturer now has their own equivalent of Apple’s AI assistant Siri and you have two examples of how LLMs have helped streamline some processes. This is all well and good for the automotive industry, but for the real impact of AI to be seen, we need to go one step further, to what we call Large Engineering Models (LEMs).
Within engineering, there are - as in any discipline - rules that need to be followed, the basics being the laws of physics and manufacturing constraints. What AI has the potential to do, is find the optimal design within certain parameters. As Henry Ford revolutionized the automotive industry with the introduction of the production line, LEMs have the potential to revolutionize the stalling EV market, and find the solutions to make them as accessible and competitive as they need to be.
Training of LEMs at a scale similar to LLMs, will require large volumes of engineering data, therefore development of high fidelity, scalable engineering simulators capable of generating the necessary synthetic data is critical for LEMs development. The synthetic data can of course be augmented with smaller values of real-world data.
Are you a pro? Subscribe to our newsletter
Sign up to the TechRadar Pro newsletter to get all the top news, opinion, features and guidance your business needs to succeed!
The aim of LEMs is to unlock for engineering and motor design what ChatGPT and Dale-2 have achieved for text and image generation. One major difference is that the output of LLMs is like that of a human, but LEMs will go well beyond the capabilities of human engineers. In electric motor design for example, LEMs will unlock exploration of motor designs that combine unintuitive free-form motor geometry shapes, together with harmonization of all the power-train components to deliver ground-breaking innovation.
Improving battery technology
The scale of effort that is currently going into energy and battery technology globally is extraordinary and there are clear signs that we will begin to see the results of these efforts in the very near future. Whilst the promise of solid state batteries is yet to materialize, companies like Toyota claim they will be able to sell cars with them, by 2026 and increase driving range to more than 800 km on a charge of 20 minutes or less. One of the key challenges in improving battery technology is the screening of material combinations. Microsoft is using AI to screen over 32 million candidate materials, accelerating the material discovery process from “years to weeks to just days”. It's clear that solutions through AI are coming.
We saw the explosion of AI in 2023, and the media focused heavily on generative AI tools such as ChatGPT when this happened, but the application of AI to achieve results that are beyond human ability will be far more exciting. The automotive industry has not yet become the glamorous place it was predicted to be and we’re not yet flying around in autonomous vehicles, equipped with million mile batteries. For this to be a reality, we need Large Engineering Models to be embraced by the automotive industry.
We've featured the best AI website builder.
This article was produced as part of TechRadarPro's Expert Insights channel where we feature the best and brightest minds in the technology industry today. The views expressed here are those of the author and are not necessarily those of TechRadarPro or Future plc. If you are interested in contributing find out more here: https://www.techradar.com/news/submit-your-story-to-techradar-pro
Jaroslaw Rzepecki is CTO at Monumo.