Enabling Edge AI to be truly transformative for industry and society
Enabling Edge AI to transform
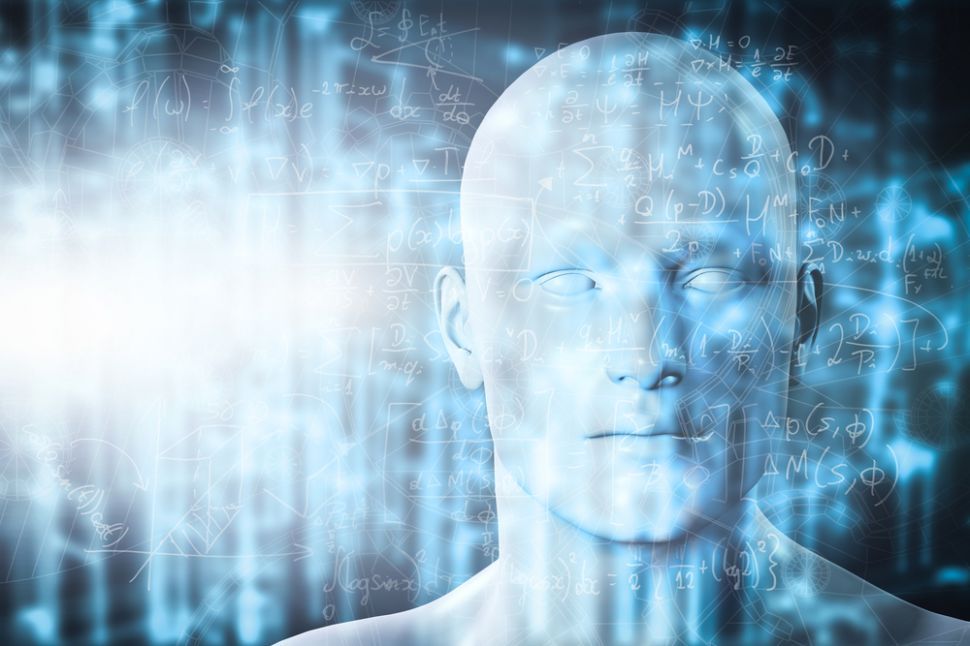
We are starting to see the adoption of Edge AI increase across various industries, and as this trend develops it will be transformative for businesses and broader society.
Freed from Cloud-based constraints, such as data congestion, connectivity faults and transference costs, decision making will become faster and more reliable. By keeping data closer to its source, where it will be harder to breach, data processing also becomes more secure.
In an industrial setting, such as an oil rig, the adoption of Edge AI could identify patterns that indicate increased risk and react accordingly, to prevent potentially dangerous situations. In agriculture, farmers could maximize yields by enabling machinery to make autonomous decisions based on environmental conditions.
From a societal perspective, imagine enabling a drone swarm to conduct a search and rescue over difficult mountainous terrain – using multiple sensors to detect shapes, sounds, heat or movement to recognize signs of life. At Mobica, we have been heavily involved in developing Edge AI models that can monitor human motion to detect signs of fatigue or injury. This technology is now being deployed by one of the world’s largest sports equipment manufacturers.
Przemysław Krokosz is an Edge and Embedded Technology Solutions Expert at Mobica.
Bringing intelligence to the Edge
As machine learning (ML) at the Edge develops and evolves, the possible AI applications will grow exponentially.
Facilitated by specialized AI hardware accelerators, such as graphics processing units (GPUs), field programmable gate arrays (FPGAs), tensor processing units (TPUs), neural processing units (NPUs), and application-specific integrated circuits (ASICs), it’s estimated that the Edge AI market will grow from almost $12Bn in 2021 to more than £107Bn by 2029.
It’s anticipated that initial growth will be led by manufacturing, with major deployments also taking place in automotive, healthcare, consumer products, IT and the energy sectors. But if Edge AI is to proliferate, there are numerous technical hurdles that will need to be overcome.
Location barriers
As with any Edge computing project, whether it involves ML or not, the organization deploying this technology will always face challenges around how to provide power and connectivity to devices that will be operating in hard to reach or remote locations. In addition, there will need to be agreements on the standards required to enable IoT devices to talk to each other.
There are also security concerns. While Edge AI has limited reliance on the internet, many personal Edge devices, such as home assistants and wearables, will want to support applications that require some cloud connectivity, which introduces an element of risk.
There are a range of power and connectivity solutions, however, such as long-life batteries, low power hardware architecture, 5G, low power hardware architecture, etc. Alongside security solutions which include dedicated security zones and private AI based on homomorphic encryption. So while location does create obstacles, the hurdles created are not insurmountable.
The AI chip challenge
Instead, where we currently find the major brake on this emerging technology is in the cost, performance and power requirements of AI chips. In some industrial scenarios, the number of IoT devices involved could extend the chip requirements to the hundreds of thousands.
Any deployment on this scale would need a careful evaluation of cost vs performance ratio, and at current prices this could prove prohibitive. At the same time, the performance vs power ratio is also too high when using existing AI chips on battery-operated edge devices.
Until we see a significant improvement of these AI computing factors, we are only likely to see small scale ML models with limited problem solving capabilities. It is conceivable that new technical advances will lead to radical improvements, however. So this is unlikely to be the biggest problem over the long term.
Enabling an educated Edge
A more significant future challenge will be how we train all these autonomous AI-enabled devices. If you look at the recent developments in Generative AI (GAI), systems such as GPT are being trained on extremely large data sets available on the internet. This requires huge effort to collect and process the information. As we look to enable educated decision making at the Edge, a lack of relevant specific training data will be a problem that needs to be solved.
If we look again at recent developments in GAI, however, the solution may have already revealed itself. One approach would be to use the ability of the generative models to produce large amounts of synthetic training data, based on a few examples provided – and then use this data to train smaller models more quickly. Another approach, perhaps further down the line, is to train a large generative model directly on live training data (if available), and also use this to train a smaller, Edge AI model.
This approach has already been seen in action, as in the case of Orca 13B, a much smaller model that has been able to learn from larger foundation models, such as GPT-4 – and is producing remarkably similar results. Many observers of recent AI developments claim that we are on the brink of a “Cambrian explosion” of small, purpose-built AI models – and these could be embedded in Edge devices to provide superhuman abilities for specific tasks.
Machine to machine learning
We could also enable faster learning by managing an interconnected, self-improving fleet of AI-enabled Edge devices from a centralized system. Having models that can be incrementally trained while being “on the assignment” and share important discoveries with others is a feasible solution in many cases.
Similar to how we share best practice across a business or industry, machines could do the same when it comes to identifying patterns that guide behavior.
Of course, to some, the idea of a fleet of autonomous machines, which are controlled by an intelligent central entity, might seem like the starting premise of a dystopian science fiction story. So, like anything involving ML, which remains in a nascent stage of development, behavioral parameters would need to be imposed.
But, it’s entirely possible that in the not too distant future, automated Edge devices, with the ability to learn from each other, will have the capacity to make increasingly educated decisions on our behalf - and this will have a transformative impact on industry and society.
Are you a pro? Subscribe to our newsletter
Sign up to the TechRadar Pro newsletter to get all the top news, opinion, features and guidance your business needs to succeed!
Przemysław Krokosz is an Edge and Embedded Technology Solutions Expert at Mobica.