Harnessing the power of Generative AI by addressing hallucinations
Gen AI models intrinsically hallucinate
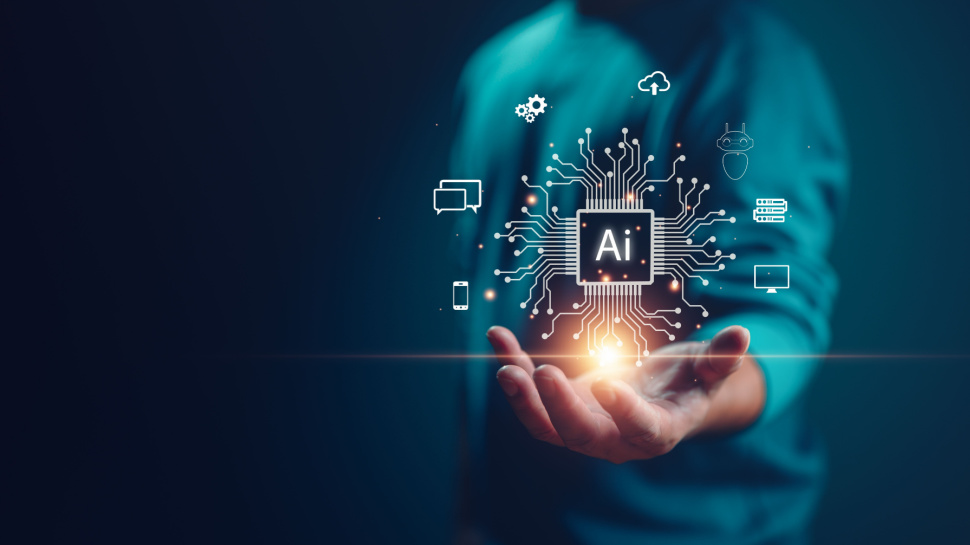
There seems to be no limit to the future of Generative Artificial Intelligence and its use cases as its applications and full power become more understood. However, Gen AI models intrinsically hallucinate, which is both a major strength and weakness.
The power of a Gen AI model comes from its ability to fabricate content that is not found in its training data. This ability is key to generating new text, images, audio, video, and even to summarize or transform existing content. On the flip side, it is a problem when such generated content is not rooted in the data provided by the user or in real-world facts. The problem is especially acute if the generated content appears plausible, because unsuspecting users then accept it as fact.
Co-founder and CEO of Simbian.ai.
The meaning of hallucinations
The term ‘hallucination’ is commonly used when a Gen AI model generates content not rooted in facts. Since most organizations are looking to harness the powerful benefits of AI, it’s important to understand the main causes of hallucinations. These include:
1. Inference Mechanisms: LLMs generate text by predicting the next word in a sequence based on patterns learned during training. Sometimes, these predictions can lead to coherent but incorrect outputs.
2. Model Overconfidence: AI models can produce outputs with high confidence, even when the underlying data does not support the conclusion. This overconfidence can result in the generation of false information.
3. Prompt Ambiguity: Vague or ambiguous user inputs can lead the AI to make assumptions, which can result in hallucinations when it tries to fill in the gaps.
4. Overgeneralization: AI models sometimes apply learned patterns too broadly, leading to incorrect inferences and information generation.
Sign up to the TechRadar Pro newsletter to get all the top news, opinion, features and guidance your business needs to succeed!
The problem with hallucinations cannot be overlooked as organizations quickly ramp their application of AI technologies. Hallucinations can cause many issues, including:
1. Misinformation and Disinformation: Hallucinations can spread false information, contributing to the proliferation of misinformation and disinformation, especially when AI outputs seem plausible and are trusted without verification.
2. Erosion of Trust: Frequent hallucinations can erode user trust in AI systems. If users cannot rely on the accuracy of AI-generated information, the utility of these systems diminishes significantly.
3. Legal and Ethical Implications: Incorrect information generated by AI can lead to legal liabilities, especially in sensitive industries such as healthcare, law, and finance. Ethical concerns also arise when AI outputs cause harm or propagate biases.
4. Operational Risks: In critical applications, such as autonomous vehicles or medical diagnostics, hallucinations can lead to operational failures, posing risks to safety and efficacy.
Addressing hallucinations
There are a number of steps organizations can take to help mitigate the risks of hallucinations. If you are building your own AI tools, the following techniques can help. If you are using a solution from a vendor, ask your vendor how their solution addresses these topics:
1. Grounding the prompt and response: Making prompts as unambiguous as possible goes a long way in ensuring the LLM response is aligned with the user’s intent. In addition, the responses can be grounded by providing sufficient context as part of the prompt. Such context includes data sources to use (Retrieval Augmented Generation) and the range of valid responses. Additional grounding can be accomplished by validating the response against the expected range of responses, or by checking for consistency with known facts.
2. User Education and Awareness: Educating users about the limitations of AI and encouraging them to verify AI-generated information can reduce the impact of hallucinations. Users should know how to accurately frame clear and precise prompts to minimize ambiguous or vague queries that can lead to hallucinations. Implementing explainable AI (XAI) techniques can help users understand how the AI generates its responses, making it easier to identify and correct hallucinations.
3. Feedback Loops and Human Oversight: Implementing systems where AI outputs are reviewed by humans can help catch and correct hallucinations, while providing continuous learning and improvement for the model. Continuous feedback loops can also help improve the model’s accuracy over time. Organizations should encourage users to report incorrect or suspicious outputs, which will facilitate the identification and correction of common hallucination patterns.
4. Enhanced Model Architectures: Developing models with better understanding and contextual awareness can help minimize hallucinations and enable models to better interpret and respond to inputs accurately. That said, developing or fine-tuning models correctly takes deep expertise, and making them safe needs significant on-going commitment. Therefore, most organizations should think twice about this option.
5. Improving Training Data Quality: If you develop your own model, ensuring that the training datasets are accurate, comprehensive, and up to date can reduce (but not completely remove) the incidence of hallucinations. Regular updates and curation of training data are essential. Removing erroneous and biased data can significantly reduce hallucinations, while incorporating verified and high-quality data from trusted sources can strengthen the model’s knowledge base.
6. Model Evaluation and Testing: Organizations should also conduct extensive testing of AI models using diverse and challenging scenarios to identify potential weaknesses and hallucination tendencies. Ongoing monitoring of AI outputs in real-world applications will help detect and address hallucinations promptly.
Conclusion
Generative AI is a huge enabler in every walk of life. Everyone should actively embrace it. Be aware of the limitations of Generative AI, especially hallucinations. The good news is that it is possible to minimize hallucinations and to contain the impact of hallucinations, with the practices listed above. Whether you build your own solution, or buy one from a vendor, checking for these practices will help reduce hallucinations and enable you to harness the full potential of Generative AI.
We list the best AI art generators.
This article was produced as part of TechRadarPro's Expert Insights channel where we feature the best and brightest minds in the technology industry today. The views expressed here are those of the author and are not necessarily those of TechRadarPro or Future plc. If you are interested in contributing find out more here: https://www.techradar.com/news/submit-your-story-to-techradar-pro
Ambuj Kuma is co-founder and CEO of Simbian.ai.