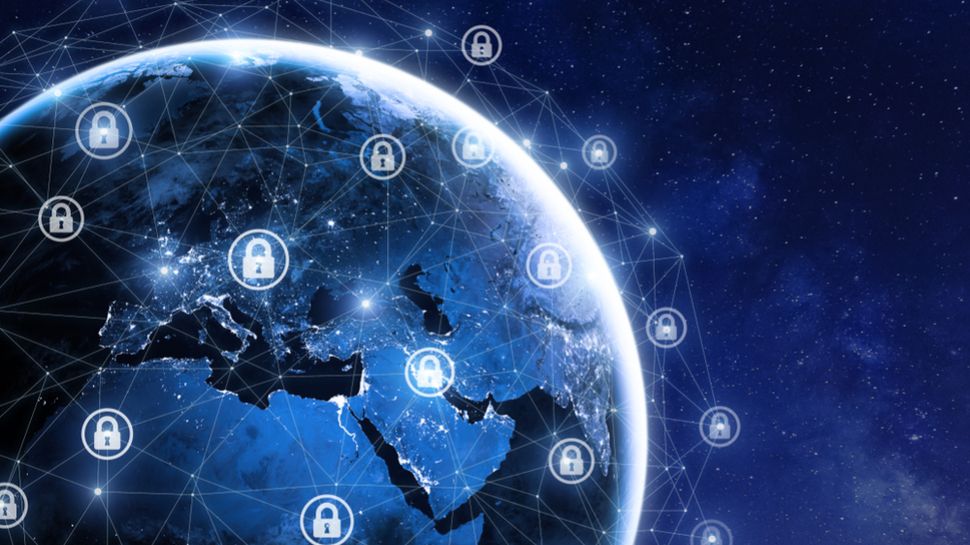
Our unabating demand for generative AI is fueling the rise of a new type of network model: ‘edge networking’. We are now witnessing the global development of edge data centers positioned closer to the end-user to meet the real-time responsiveness and low latency performance demands of GenAI. To put this into figures: analyst firm, IDC, predicted that spending on edge computing is expected to reach $232 billion in 2024, up 15.4% from 2023.
Edge data centers are small data centers and compute locations that form part of a distributed network of data centers. They are based close to the areas they serve – significantly reducing latency and enhancing the performance of applications that require real-time data processing. This decentralized approach also helps to balance loads, ensures flows of data in the event of an outage and improves the overall resilience of a network.
Chief Technology & Operating Officer, Neos Networks.
Supporting GenAI
The case for edge networking is clear. AI applications are both data-heavy and compute-intensive. However, edge computing promises to overcome these technical challenges by enabling real-time decision-making with reduced latency, local data storage and processing and reduced data transfer to the cloud. This is particularly pertinent when it comes to the need for inferencing and localized processing of data.
With GenAI requiring even faster processing, there will be many existing and new applications where networks will need to deliver ultra-low-latency. The more time-critical the application is, the more that data should be stored and processed at the edge. Take AI inferencing (using an AI model to conclude from new information or data) for example.
Processing data at the edge can reduce the time for a result from a few seconds to a fraction of a second. In addition, several other emerging industry use cases highlight why compute must be placed close to the end-user – whether that be content generation applications like ChatGPT, interactive customer service agents, immersive AR experiences, smart healthcare and smart retail and predictive maintenance. In these scenarios where every millisecond counts, the user will enjoy a higher quality experience if the compute is hosted as close as possible to them.
The sustainability argument
A recent TechRadarPro article argued that we do not have the power to handle the current data center demand boom, due to AI. This is why we must build out data centers outside central locations at the edge. According to Goldman Sachs, a ChatGPT enquiry requires almost 10 times as much electricity to process as a Google search. Despite the inevitable spike in electricity expenditure from GenAI, edge data centers offer the advantage of reducing grid power consumption in central locations. By distributing the computing burden across the network, power demand is spread, not concentrated. By running applications at the edge, data can be processed and stored nearer to the end user’s devices, rather than relying on data centers that are hundreds of miles away.
Investing in an AI-ready network
Investment in high-speed connectivity will connect edge sites in the network more practically and sustainably. Fiber-optic cables provide significantly lower latency and higher bandwidth than traditional copper cables. This allows for faster data transfer rates. High-speed fiber networks are easily scalable so as demand for data grows, additional bandwidth can be provisioned without significant infrastructure changes. Fiber networks also consume less power than traditional infrastructure, contributing to lower operational costs and a smaller carbon footprint. With advancements in pluggable optical technology, the same economic, sustainability and technology benefits derived from fiber are now being delivered inside the data center.
Are you a pro? Subscribe to our newsletter
Sign up to the TechRadar Pro newsletter to get all the top news, opinion, features and guidance your business needs to succeed!
However, while projects such as the UK’s Project Gigabit and the US’ Broadband Equity, Access and Deployment (BEAD) program are a necessary step in the right direction, governments must prioritize building out the network edge and better connecting data centers – not just expanding fiber-to-the-home (FTTH).
The key to unlocking AI success
As countries race to become leaders in AI, bolstering start-ups and defining regulation parameters are top of the agenda. However, AI success will depend on the country’s fixed network infrastructure and its ability to carry significant amounts of data with little to no latency. If networks cannot cope with the influx of traffic generated by ‘always on’ Large Language Models (LLMs), AI ambitions may falter.
This is why respective AI strategies must focus on the size, location and quality of the underlying network infrastructure. Despite widespread investment in ‘traditional’ data centers snowballing across the world, such as Google’s new $1 billion data center in the UK announced earlier in the year and Microsoft’s AUD$5 billion investment in building data centers in Australia, there has been less of a focus on edge data centers. To meet AI demands, data center buildout needs to be supplemented by edge buildout.
A hybrid model?
A hybrid approach of strategically placed data centers at the edge of the network, in combination with central data centers, will be essential to manage the rapid information flow cost-effectively and sustainably. This is particularly crucial for AI inferencing, where data flows go to the edge for processing and then back to core data centers for distribution. Time-critical applications will be served better closer to the edge of the network, but data-heavy and less time-critical applications will be better served in central data centers.
With major companies like Microsoft having ambitious targets to triple its data center capacity within the next year to deliver AI, we must also see edge data centers being a considered part of the strategy – not only to meet the low latency requirements of GenAI applications but to take the power pressure off the central grid.
This article was produced as part of TechRadarPro's Expert Insights channel where we feature the best and brightest minds in the technology industry today. The views expressed here are those of the author and are not necessarily those of TechRadarPro or Future plc. If you are interested in contributing find out more here: https://www.techradar.com/news/submit-your-story-to-techradar-pro
Matt Rees, Chief Technology & Operating Officer, Neos Networks.