How businesses can break barriers to entry in integrating AI into operations
Breaking barriers to entry in AI integration
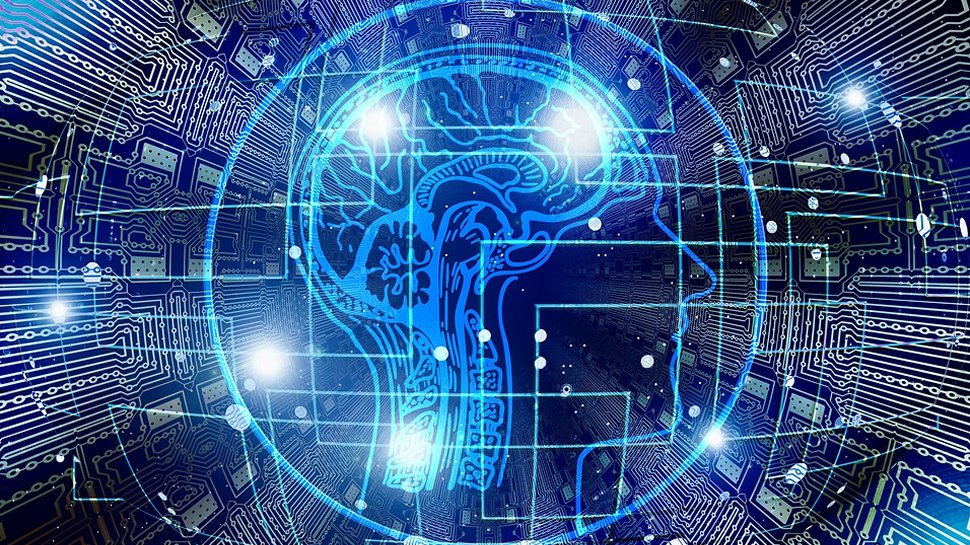
By now, many enterprises are aware that integrating AI and generative AI into their work processes can streamline operations, improve efficiencies, and save them time and money. Some are on their way to meeting this reality; according to Deloitte’s recent generative AI report, 18-36% of organizations say they are already achieving the benefits they expect from the use of generative AI to a “large” or “very large” extent, depending on the type of benefit being pursued.
However, despite the clear advantages of leveraging this ground-breaking technology, integrating fast evolving AI into business operations also presents several challenges. As AI continues to develop, the obstacles facing business leaders when adopting these technologies also continue to grow. There are therefore challenges that must be overcome for organizations to fully harness the benefits of AI and generative AI – but business leaders can rest assured that there are key strategies they can implement that will play a crucial role in enabling successful integration.
Global Business Lead for Hitachi Vantara.
Don’t do AI for AI’s sake
A common pitfall for many AI projects is the absence of a coherent strategy and defined objectives. An AI project will fail to deliver, even with heavy investment, if businesses don’t take the time to align it with their company’s goals and define exactly how it will add value – and how much value – relative to the cost of implementation. After all, there’s no point rolling out a project with the goal of adding five million pounds to your top line, if it’s going to cost you ten million to get there.
Whether you aim to deploy in your own data center or in the cloud, AI projects can be expensive, especially when you factor in both the infrastructure needed for deployment and the services needed to make it all work. Organizations therefore need to be very clear as to why they’re doing what they’re doing and what the return on investment will be. Business leaders should resist the urge to jump on the AI bandwagon and instead pursue thoughtfully conceived projects that align with the overarching goals of their organization.
Part of this means exercising caution against “AI washing” – the exaggerated promotion of overhyped AI solutions – and concentrating on pragmatic applications that deliver genuine value. These may well be smaller, more niche use cases, as opposed to massive process overhauls. For example, a construction company that counts health and safety as a key business priority might install cameras with AI onsite that can monitor workers’ workwear throughout the day. If someone isn’t wearing the right protective gear, the AI will flag to a supervisor who can step in to provide it, ensuring optimum health and safety levels at all times. In this way, the company is leveraging AI in a way that presents them with tangible, measurable business results that are right for them.
To aid them in finding these targeted use cases, organizations should look for partners who can help them to analyze their business from the outside in and identify the areas where AI can really make a difference for them.
Well-ordered data is the backbone of successful AI
Once they have defined their AI strategy, organisations then need to consider how they can successfully implement it. AI is extremely data-heavy, particularly when it comes to some of the latest generative AI use cases being explored. Businesses must therefore be able to locate all their data assets, consolidate and clean their data, and streamline their repositories, to render them suitable for AI applications. This demands a comprehensive understanding of both their data sources and storage services.
Are you a pro? Subscribe to our newsletter
Sign up to the TechRadar Pro newsletter to get all the top news, opinion, features and guidance your business needs to succeed!
To create an AI chatbot, for example, a company needs to train it on a plethora of disparate data sources, from user manuals to previous customer call conversations. Only then can it be programmed, using that existing data, to respond accurately to common questions.
Get the right skills onboard and pay attention to regulations
In order to successfully execute AI projects, enterprises must find or recruit the necessary skills into their business. With AI expertise currently in extremely high demand, those with the relevant skillset can be both hard to find and expensive, so allowing time for this should be factored in.
They must also stay abreast of evolving AI regulations to ensure compliance. For example, the European Union’s landmark AI Act recently came into effect, regulating the development, use, and application of AI for developers and deployers alike. This significant step highlights the importance placed on the safe and ethical development of AI technologies within Europe – a sentiment that we are also seeing come into force across the globe.
With these regulations, there are numerous elements to consider. If organizations are feeding data into AI models, they must, for example, ensure that they’ve obtained the right consents to use it, and that it is anonymized as required. There are also restrictions on data leaving the premises of whoever gathered it – to move it to the cloud, for example.
Back to basics
If the right strategy, skills and data sources are not in place, AI projects could fail to be successful. However – somewhat reassuringly – this is not a new challenge; these are all obstacles that may have contributed to the failure of IT projects since such projects began. Yes, the possibilities that AI presents are different, but the fundamental elements that enterprises must think through when implementing these projects remain the same. It’s a fact that many business and IT leaders should take solace in when embarking upon their AI journeys.
If enterprises can do their homework – with support from the right partners – to ensure that the AI initiatives they look to integrate will contribute tangible value to their business, and then take the necessary steps for a rewarding implementation, they will set themselves up for success. Successful AI integration is there for the taking – organizations first just need to take the time to really get it right.
We've featured the best AI phone.
This article was produced as part of TechRadarPro's Expert Insights channel where we feature the best and brightest minds in the technology industry today. The views expressed here are those of the author and are not necessarily those of TechRadarPro or Future plc. If you are interested in contributing find out more here: https://www.techradar.com/news/submit-your-story-to-techradar-pro
Sasan Moaveni is Global Business Lead for AI & High-Performance Data Platforms, Hitachi Vantara.