Looking before we leap: why security is essential to agentic AI success
Agentic AI has huge potential, but carries significant security risks
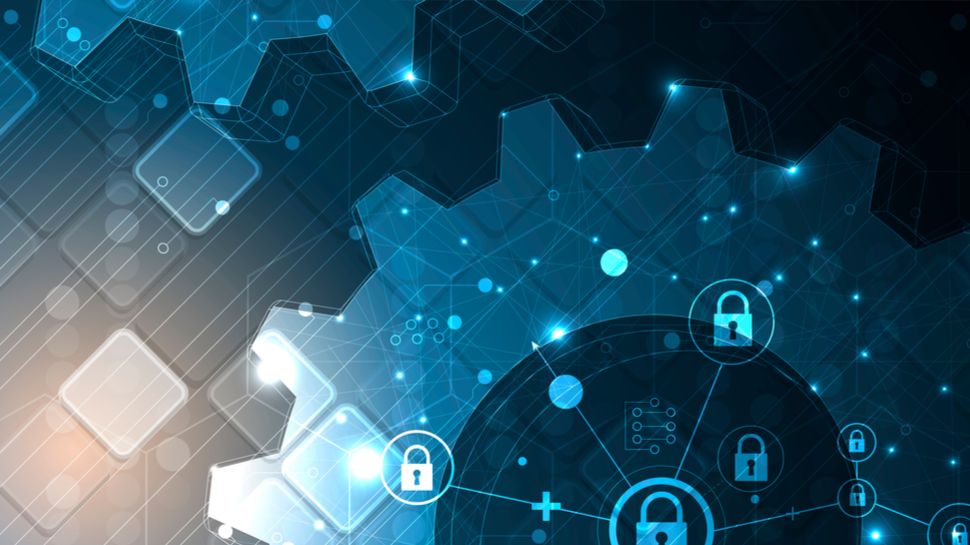
Is 2025 shaping up to be the year of agentic AI? The hype is already building as experts predict a new wave of the technology will drive two or three times the productivity gains possible with current large language models (LLMs). Governments are piling in: most recently, the UK’s new Labour administration announced plans to “turbocharge” growth and deliver a “decade of national renewal” via an AI Opportunities Action Plan.
But where there is opportunity, there is also risk. Agentic AI offers threat actors new ways to reach sensitive data and sabotage business-critical systems for gain. The autonomous nature of the technology could also lead to unintended consequences and potentially unsafe decision-making.
Organizations must therefore try harder to understand where risk is most acute, and take proactive steps to mitigate it before embarking on their own agentic AI journey.
Field CTO at Trend Micro.
Agentic AI for good and bad
Does the tech match the hype? Salesforce certainly thinks so. It describes agentic AI as a “third wave” of innovation, following predictive AI modelling and LLM-powered generative AI. The company’s chief scientist, Silvio Salvarese, describes where the tech is headed:
“Self-adaptive agents enabled by multi-agent reasoning—agents that can learn from their environment, improve through experience, and collaborate both with humans and agents from our enterprise customers, partners, vendors, and even the personalized AI assistants of consumers, which are becoming a bigger part of their lives every day.”
This is good news, not just for the large organizations already trialing the technology, but smaller businesses that will also benefit in time as it becomes more mature. PwC claims the technology could generate between $2.6tn and $4.4tn annually for global GDP by 2030.
Yet as AI systems move from assistive use cases to working dynamically and proactively on their own, caution is also required. Threat actors will relentlessly target a rapidly expanding AI attack surface, probing for vulnerabilities and misconfigurations to steal training data and data stored in “vector” databases, as well as look for opportunities to poison data/models. With unauthorized access, they can feed incorrect or biased data into the AI system to manipulate its behavior/outputs. There’s also a risk of introducing malware and/or vulnerabilities from the supply chain; especially the open source components used in great number by AI developers.
All of which could result in data breaches, extortion, service outages and major reputational/financial risk.
Unintentional misalignment
Yet there’s more. Because the value of agentic AI systems is that they can work autonomously, there’s a risk of models making unpredictable decisions. This is what’s known as “unintentional misalignment”—as opposed to “intentional misalignment” which occurs when someone deliberately tries to use AI in attacks, perhaps via prompt injection or data poisoning.
There are plenty of examples of unintentional misalignment to be concerned about. Consider a self-driving car programmed to prioritize passenger safety. It may misinterpret these directions by swerving into pedestrians to avoid a minor collision with another vehicle, thereby causing a far more severe accident. Agentic AI might also unintentionally DoS the infrastructure it runs on if resource consumption isn’t carefully controlled—by creating endless sub-problems to solve.
RAG risk is already here
These aren’t necessarily theoretical risks. Retrieval augmented generation (RAG) is another emerging type of AI which, like agentic systems, is designed to overcome the limitations AI developers are finding with LLMs—ie that training data is beginning to run out. RAG uses search algorithms to query third-party data sources like web pages and databases, processes what it finds, and then integrates it into a pre-trained LLM. In this way, it can provide more accurate and up-to-date answers than a traditional LLM could—and in so doing, reduces the likelihood of hallucinations. That’s why it’s increasingly popular in use cases like financial analysis, patient care and online product recommendations.
To function, it utilizes various components including LLMs, LLM-hosting platforms, open source code, and vector databases, which provide the crucial indexing and retrieval capabilities. However, these are riddled with security holes. Aside from the well-understood risks of malicious or vulnerable open source components, research reveals the existence of multiple CVEs, from 2024 alone, in LLM-hosting platform Ollama. With over a thousand new releases each year for the platform, these become hard to track, never mind patch. The same research reveals vulnerabilities in popular vector databases like Weaviate.
It also claims to have discovered scores of servers running open source LLM-hosting software llama.cpp, hundreds of instances of vector database ChromaDB, and thousands of misconfigured Ollama servers—all exposed to the internet without any authentication required. In the case of Ollama, this could provide threat actors with access as many as 15,000 discrete LLMs. Alongside vulnerability exploitation, this presents threat actors with an attractive opportunity to steal sensitive data and sabotage, manipulate or disrupt AI services. Given that agentic AI uses many of the same components as RAG—including LLMs and vector databases—it is arguably exposed to similar threats.
Stepping back and managing risk
So how can organizations hope to get back on the front foot? Most importantly, by approaching AI from a security-by-design perspective. That means ensuring security leaders get a seat at the table when new projects are being discussed. And that data protection impact assessments (DPIAs) are run before any new initiative is launched.
First, take a “human-in-the-loop” approach to ensure critical decisions made by agentic AI can be reviewed and, if necessary, overridden by IT experts. Real-time monitoring of AI behavior and performance will flag when there’s something anomalous for members of the IT team to inspect. Periodic audits of AI systems can also help to ensure they are making properly aligned (and no biased or risky) decisions.
A focus on governance is also important to provide ethical guidelines for AI development and usage, and regular reviews for compliance with such rules. Employees handling AI should be trained to enhance their literacy of the technology, and ability to use it ethically, safely and securely.
Finally, organisations should look to AI security leaders to help mitigate immediate cyber-related risks. Zero trust is an appropriate approach to take here, to ensure only authorized users can access AI systems, and to swiftly detect malicious activity like prompt injection and data leakage/theft.
As a wave of agentic AI breaks over global organizations, and the tech becomes embedded in ever more business processes, the risk of something going wrong—intentionally or otherwise—will only grow. Let’s get ahead of that risk now, rather than wait for it to escalate and potentially derail important projects.
We've featured the best AI phone.
This article was produced as part of TechRadarPro's Expert Insights channel where we feature the best and brightest minds in the technology industry today. The views expressed here are those of the author and are not necessarily those of TechRadarPro or Future plc. If you are interested in contributing find out more here: https://www.techradar.com/news/submit-your-story-to-techradar-pro
Are you a pro? Subscribe to our newsletter
Sign up to the TechRadar Pro newsletter to get all the top news, opinion, features and guidance your business needs to succeed!
Technical Director UK & Ireland at Trend Micro.
You must confirm your public display name before commenting
Please logout and then login again, you will then be prompted to enter your display name.
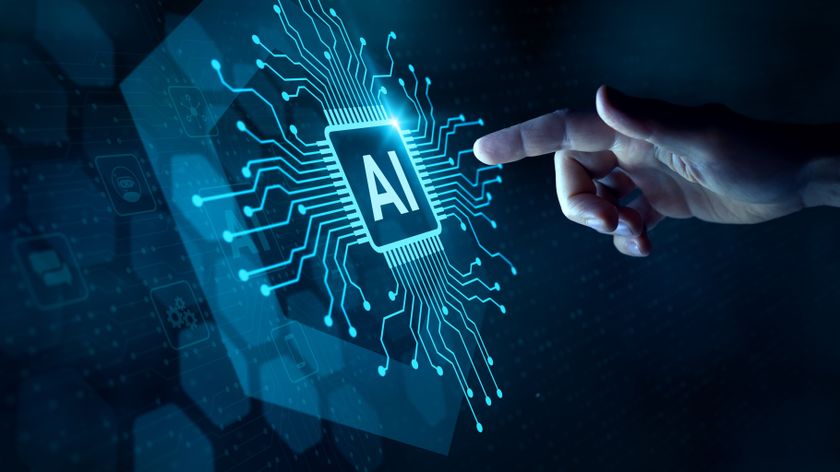
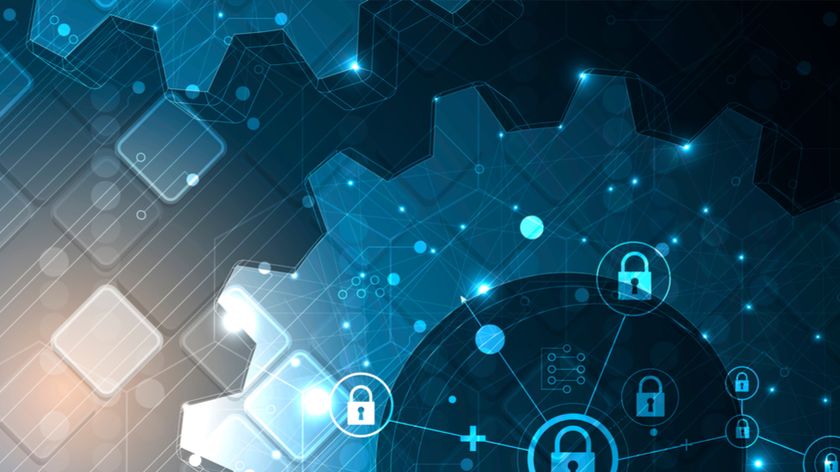
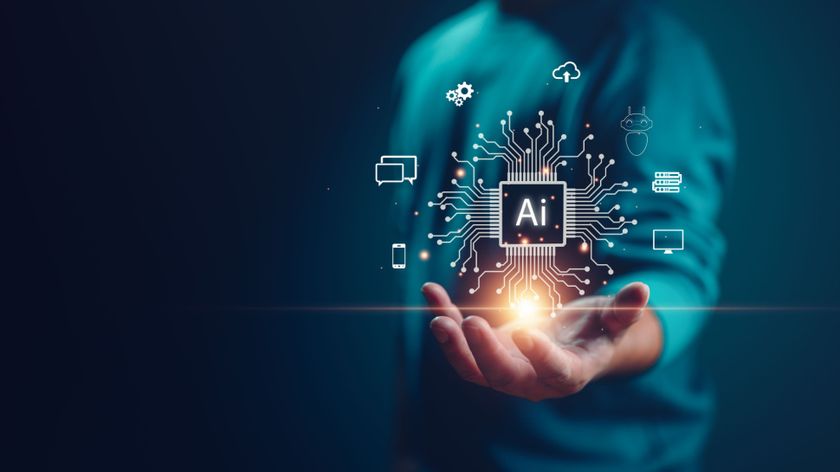
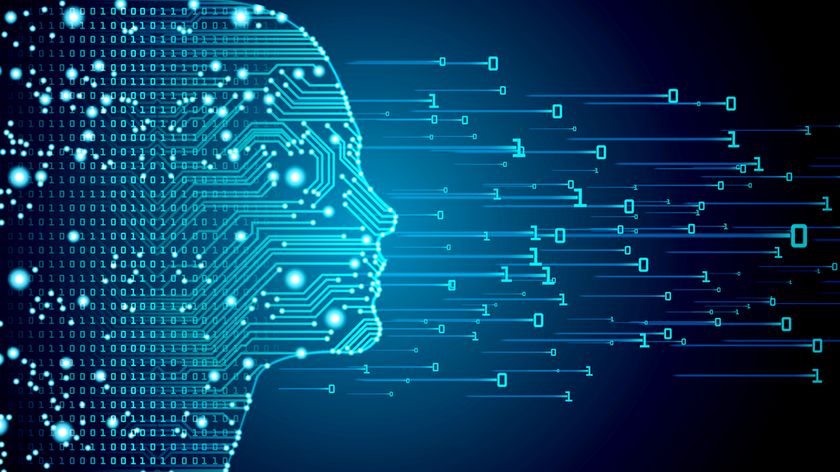
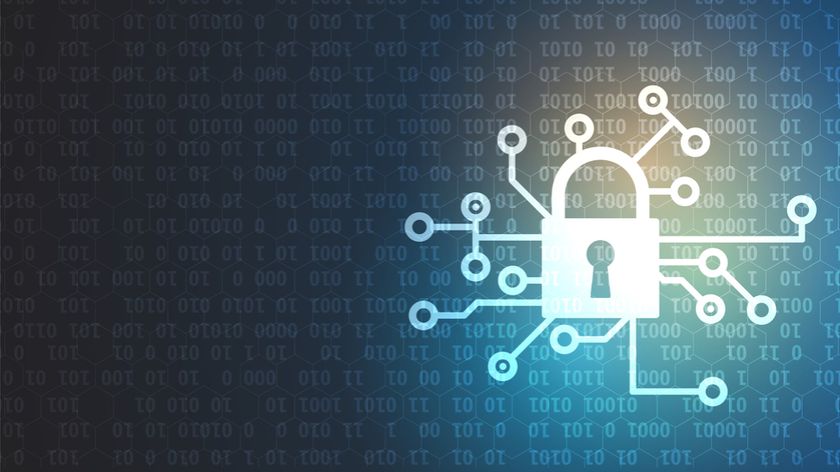
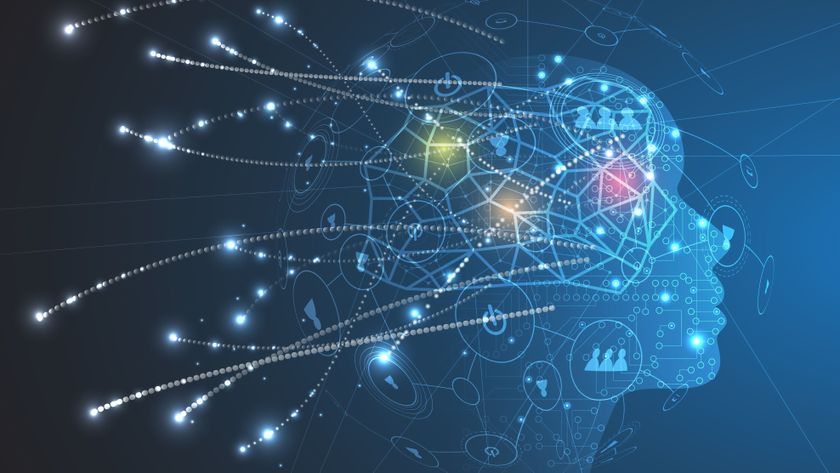
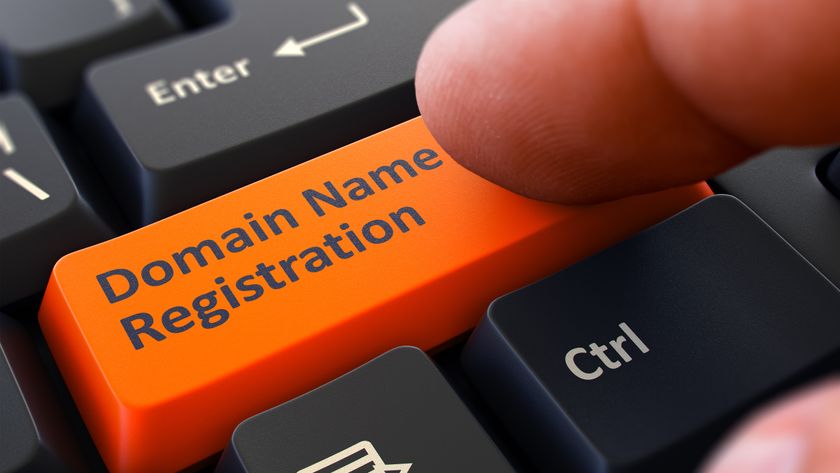
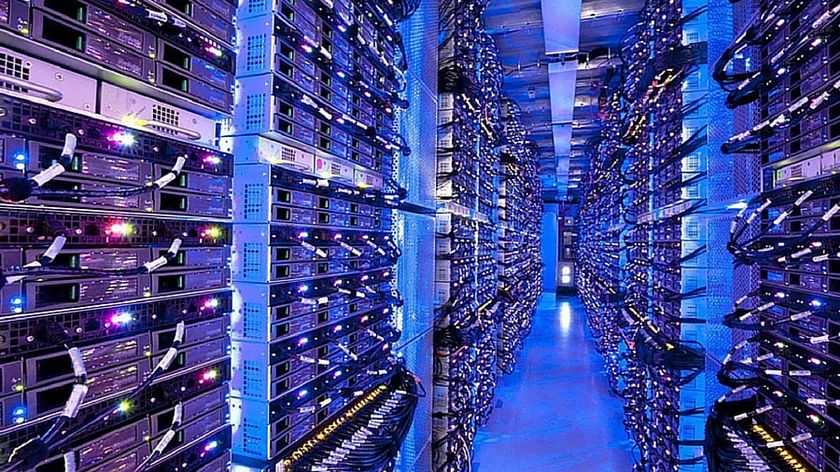
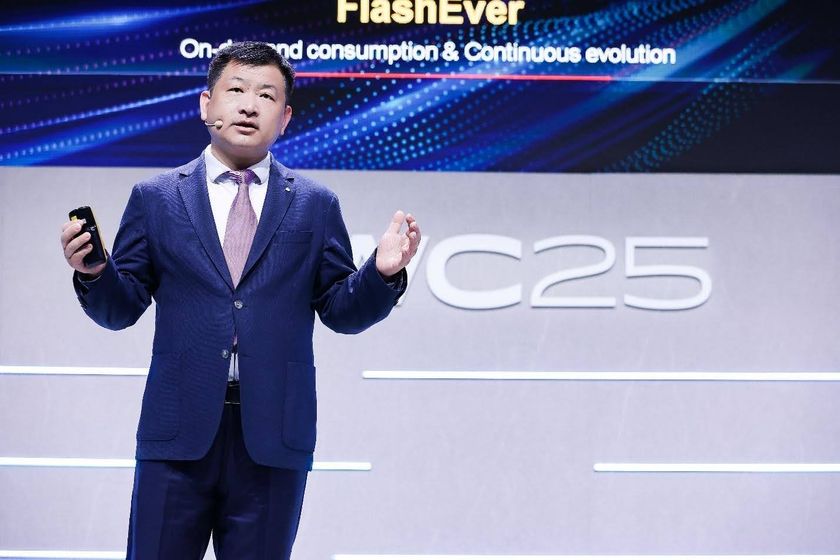
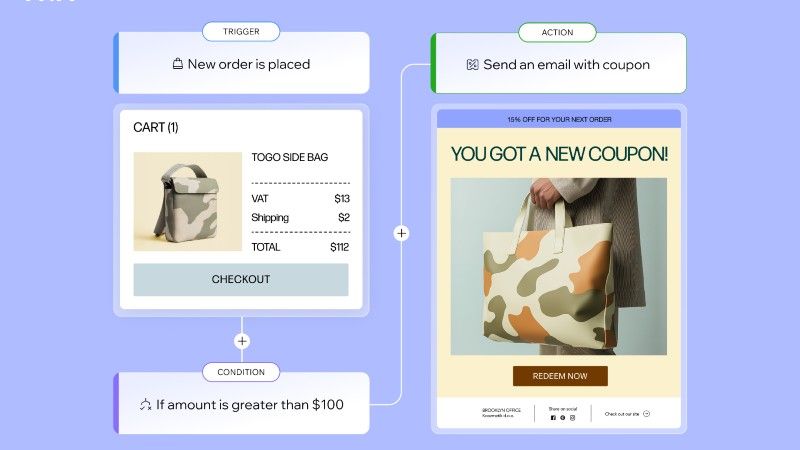
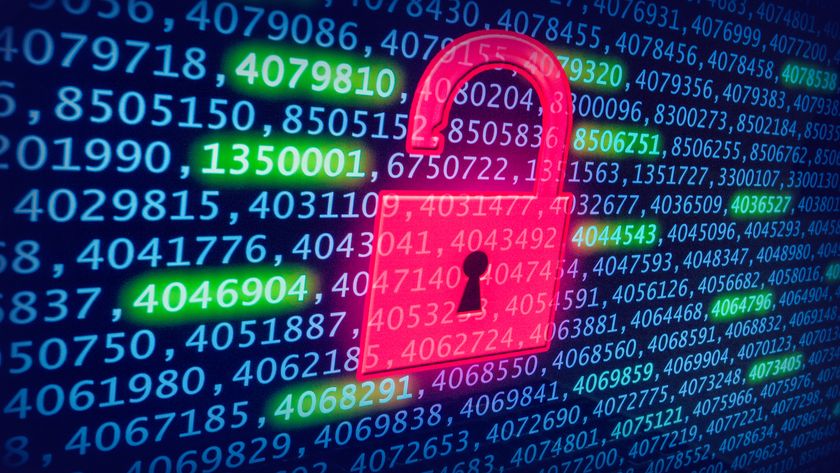
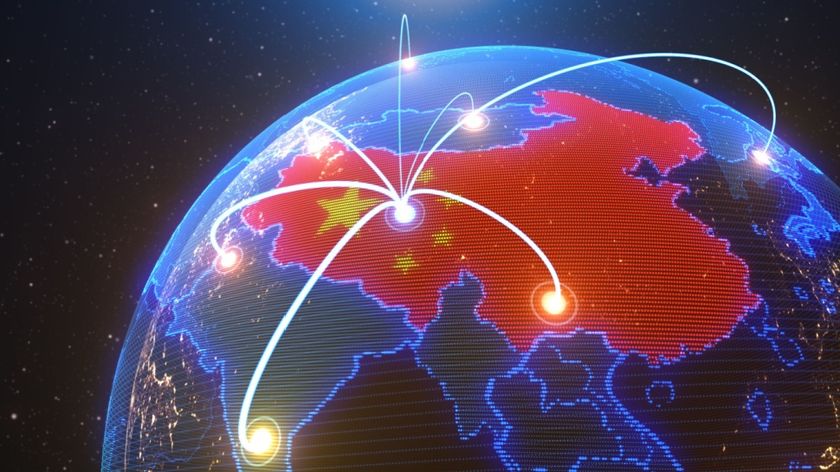
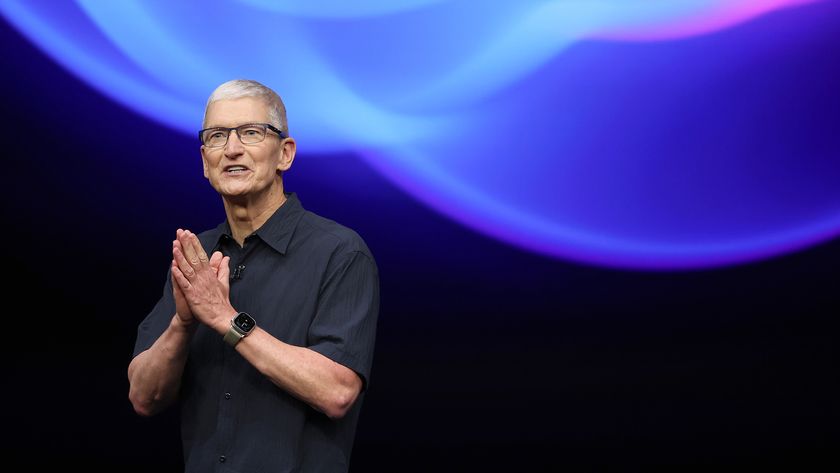
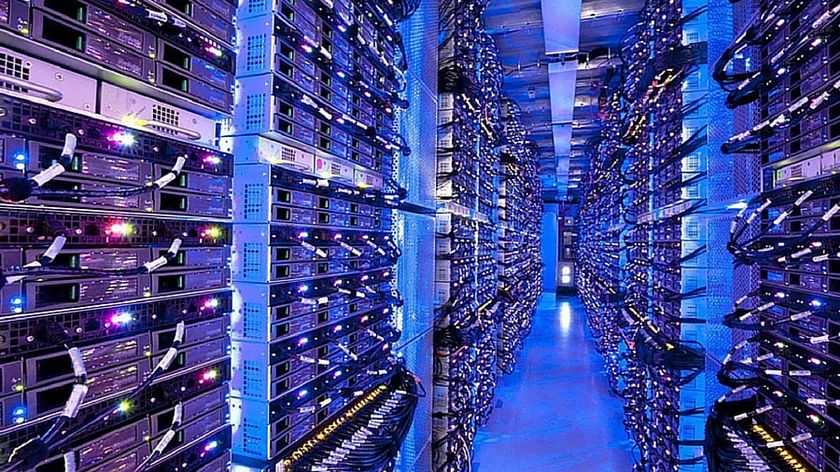
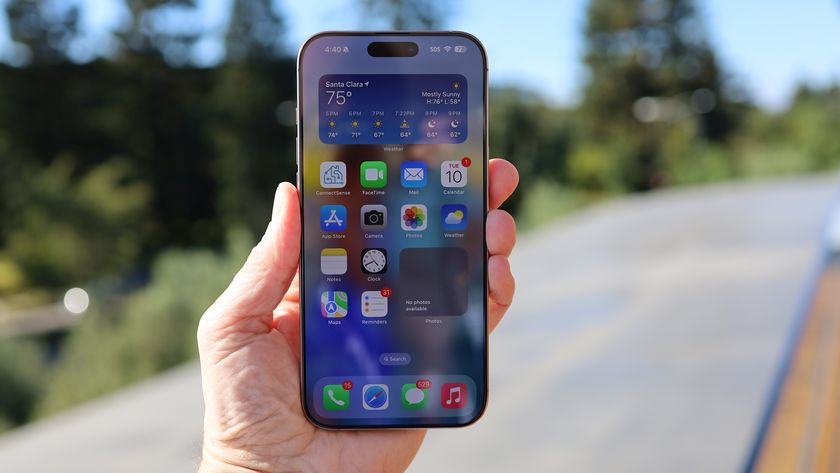
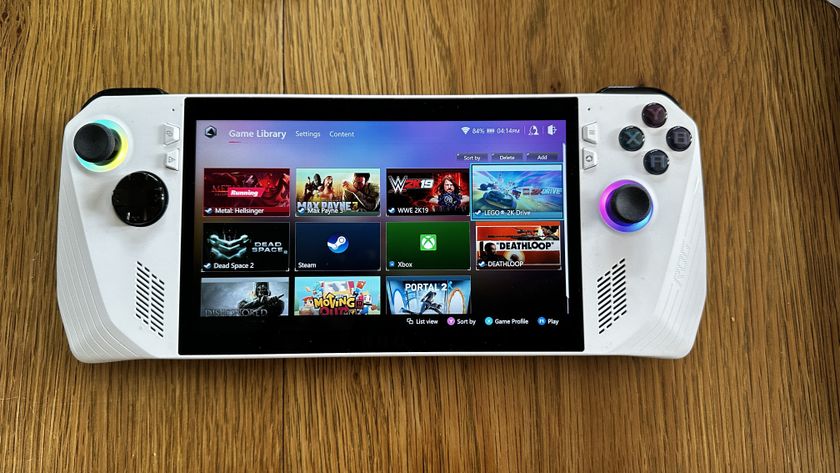
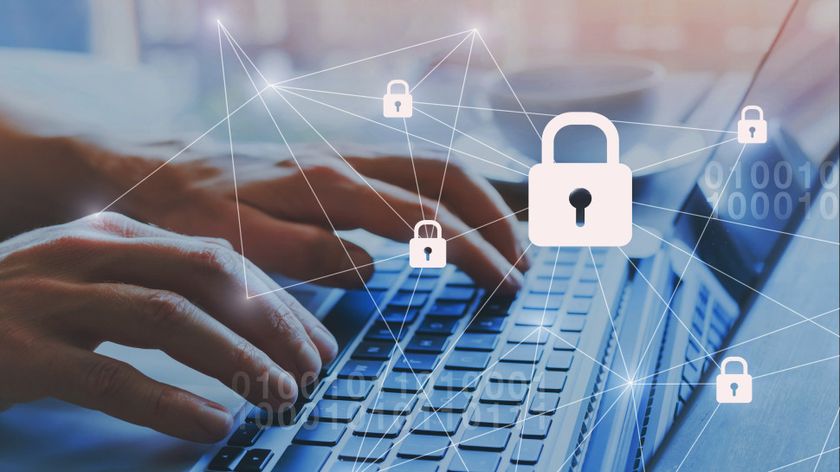
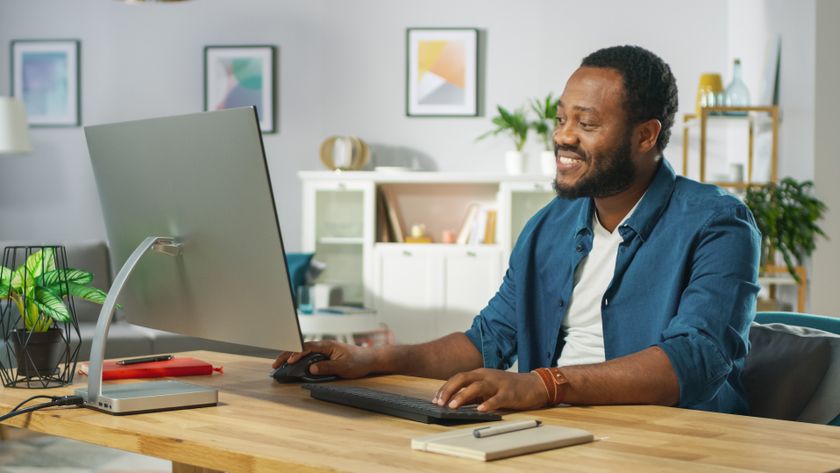