Questions you should ask AI… before implementing AI
What should businesses be asking AI before implementing AI?
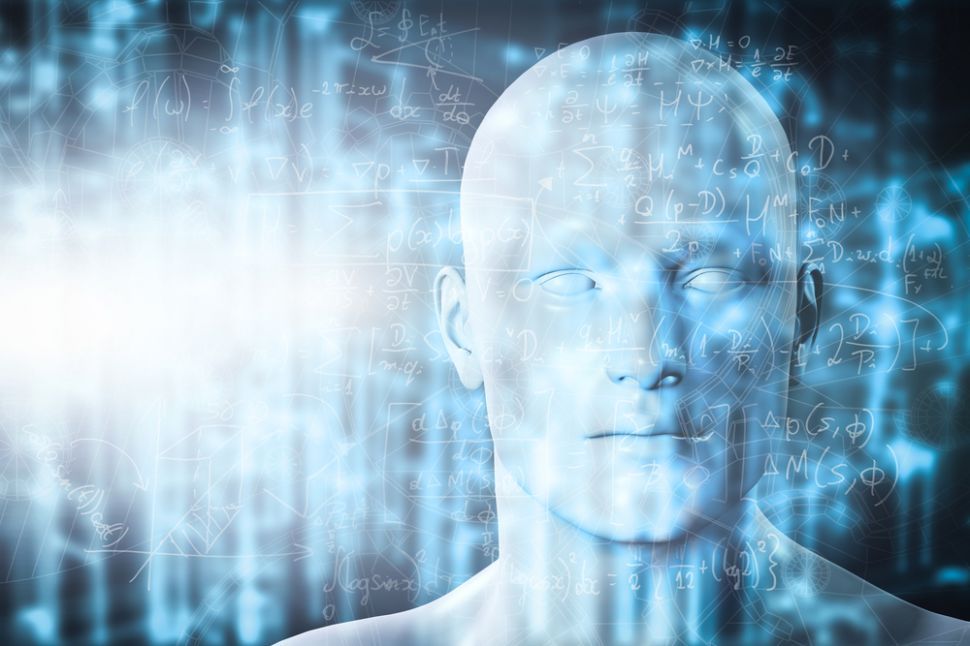
Across all industries, there’s a consensus that advances in AI have the potential to revolutionize a whole host of business processes. While some organizations are beginning to take AI from the lab to production, most have only just started to dip their toes into the water and are still in the exploratory phase of deployment.
However, in the race to establish a competitive edge, many organizations have rushed into AI deployments, meaning there have been some early teething problems. In some cases, AI’s output has been incorrect because it lacks the contextual data required for accurate responses. In others, organizations have effectively leaked valuable data via AI as they didn’t have strong governance in place.
Organizations must take a considered approach to AI deployment, weighing up the risks and ensuring that they have guardrails in place to mitigate them. At the same time, it is important to establish the most valuable use cases. However, with so many practical applications of AI emerging every day, from chatbots to risk modelling, it’s difficult to know where to begin.
Somewhat ironically, AI can help here too.
EMEA Field CTO at Cloudera.
AI prioritizing AI
By using a LLM to conduct background research, organizations can gain a better understanding of exactly where AI can provide the most value in just a few minutes. LLMs can also help organizations to prioritize these use cases by answering questions such as:
- What are the top 10 use cases for AI in my industry? Here, organisations can quickly cut through the noise and gain insight into where AI can provide the most value, specific to their industry. Some will be universal – such as content recommendations for marketing or chatbots for customer service. But other use cases will be more industry-specific, like network optimization for telcos or credit risk assessment for banks.
- Can you rank these use cases by financial impact on revenue? One aim of enterprise AI is to increase revenue. So, the next logical step is to understand which of these use cases will have the greatest impact on revenue, so that organizations can focus on the most impactful ones first.
- Can you map these use cases against the EU AI Act’s risk categories? Across the globe, regulators are looking to govern the safe use of AI. But the EU is a step ahead of most, having recently passed the EU AI Act, which applies to both companies based in the EU and those with operations there. So, mapping use cases against the EU AI Act can help organizations to understand the risk of deploying AI.
- My company operates in [insert locations]. Can you tell me which regulations these use cases could infringe upon? Outside of AI-specific regulations, there is also a mosaic of legislations that organisations must abide by, particularly if they operate internationally and in heavily regulated sectors like finance. LLMs can help shed light on the broad array of regulations that will apply to AI use cases.
These questions will provide organizations with a great starting point. But it’s important to not take this information at face value and supplement it with further research, as knowledge is key to making any informed decisions. LLMs can provide references for their own findings which will provide some direction for further reading around potential use cases to present to the business.
Armed with this knowledge, organizations will have a better understanding of where AI can help them the most. But having the ability to utilize AI is one thing, successful deployment is another – it means more than understanding use cases, risks and regulations.
Are you a pro? Subscribe to our newsletter
Sign up to the TechRadar Pro newsletter to get all the top news, opinion, features and guidance your business needs to succeed!
Data must be AI’s foundation
To drive real value from AI, organizations need to ensure their data is laying the groundwork for success. However, in today’s hybrid, multi-cloud environments, data often sits in siloes, making it hard to access. Across such vast, distributed environments, implementing consistent control and compliance is also a challenge.
This is why having a unified data platform supported by a modern data architecture is important. It enables organisations to provide AI with data from across any environment – whether in the cloud or on-premise. Strict governance can also be enforced to ensure that data doesn’t leak outside of an organisation and evoke the wrath of regulators.
Getting the most from AI
As utilizing AI in production becomes more common, prioritizing use cases will be key to success. But organizations need to take initial steps to ensure they’re truly ready for enterprise AI, and not just following the crowd.
With a modern data architecture in place, organisations can lay solid foundations for AI success. But this must be the first step or organizations risk embarking on AI projects that are doomed to fail from the start.
We've featured the best AI writer.
This article was produced as part of TechRadarPro's Expert Insights channel where we feature the best and brightest minds in the technology industry today. The views expressed here are those of the author and are not necessarily those of TechRadarPro or Future plc. If you are interested in contributing find out more here: https://www.techradar.com/news/submit-your-story-to-techradar-pro
Chris Royles is Field CTO for EMEA at Cloudera.
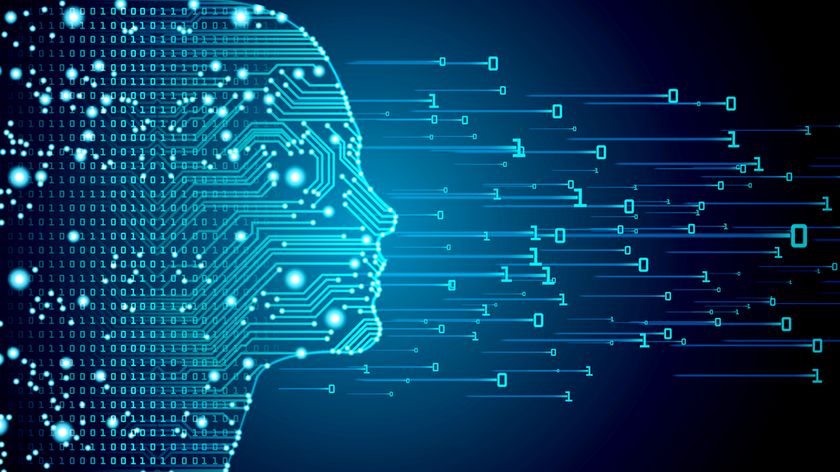
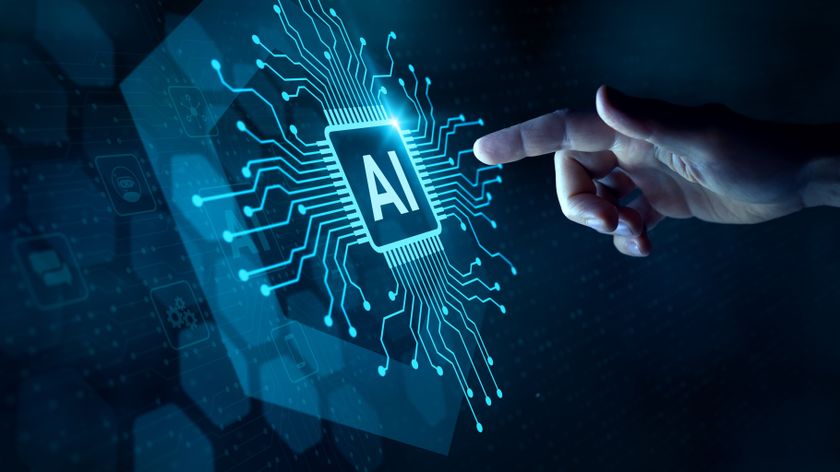
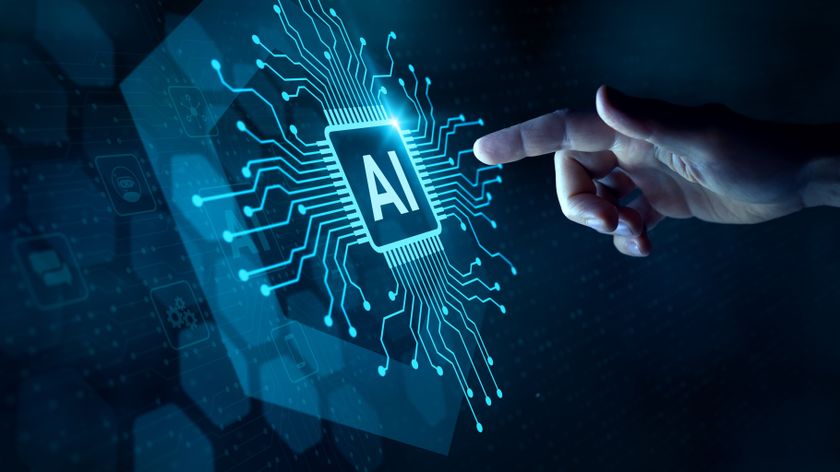
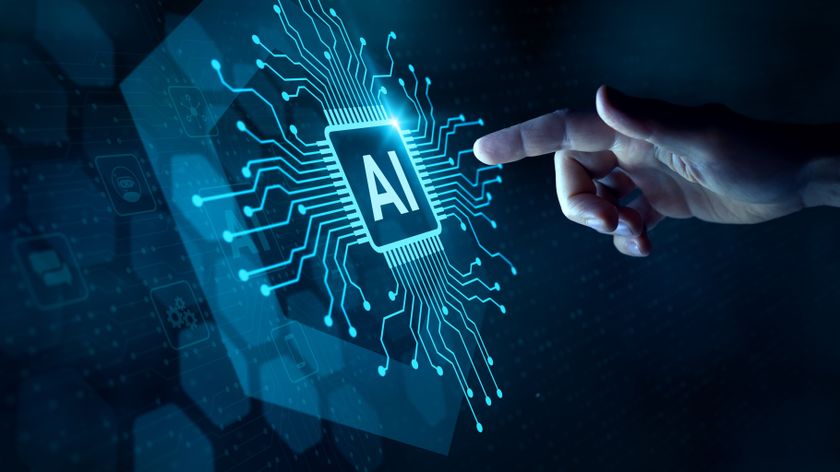
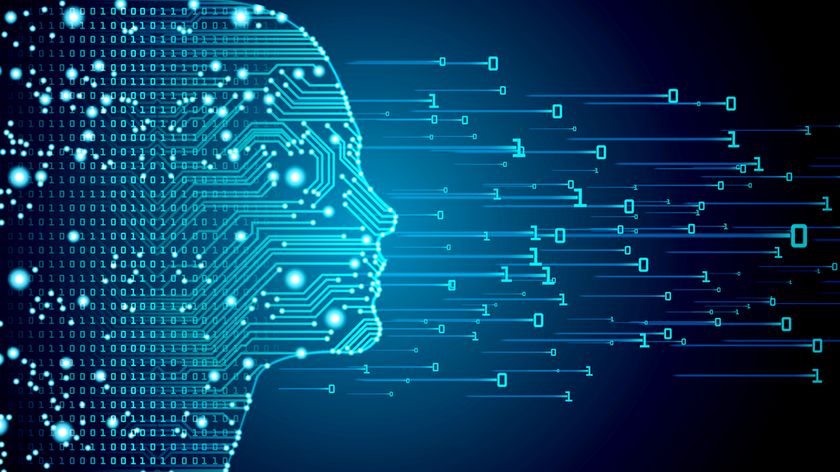
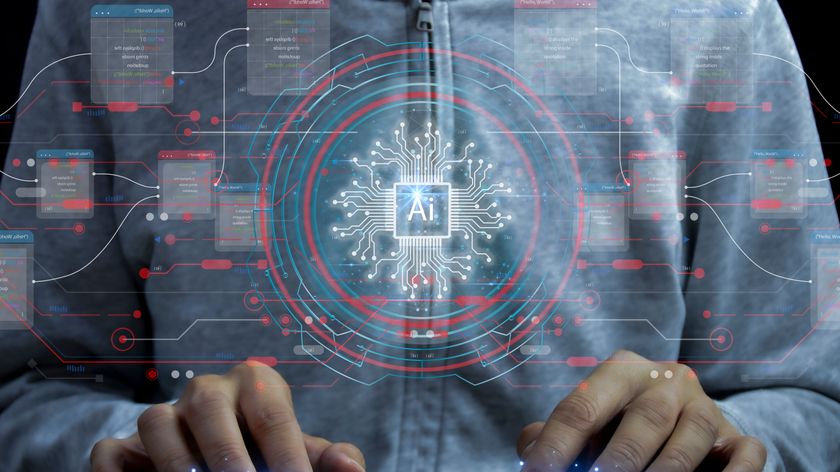
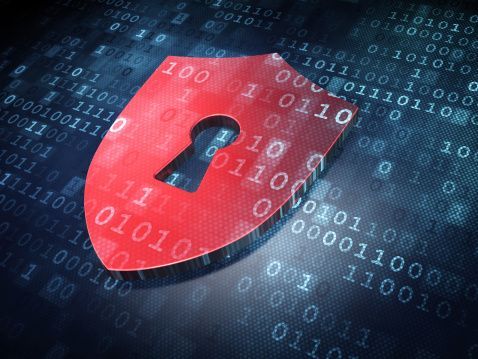
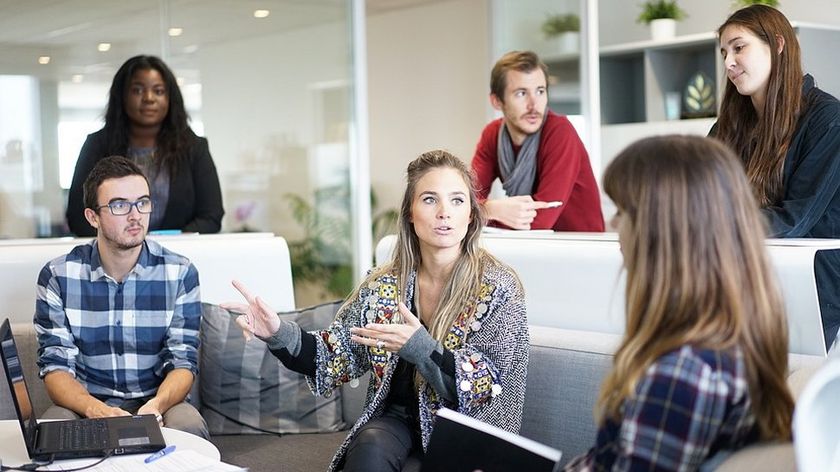
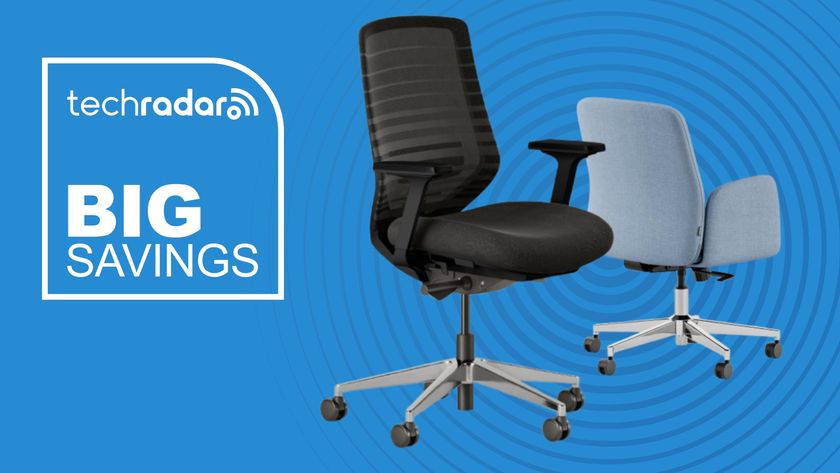
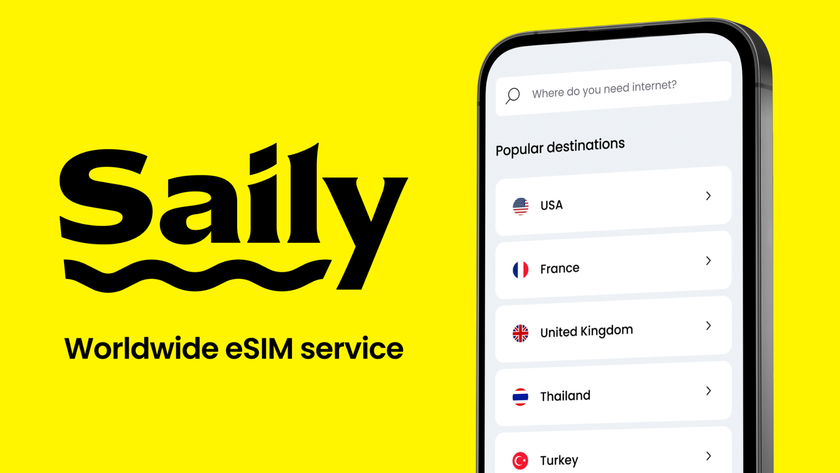
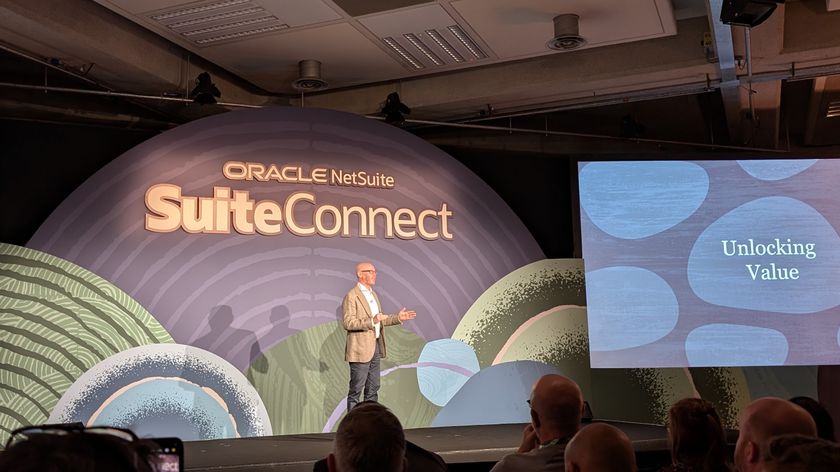
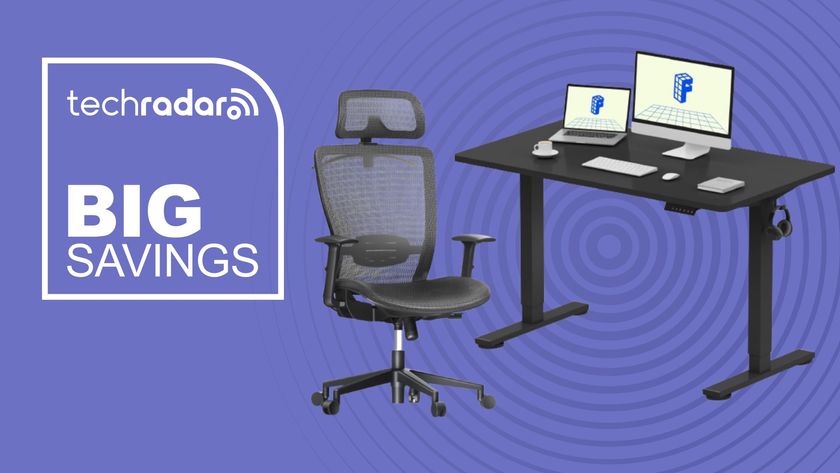
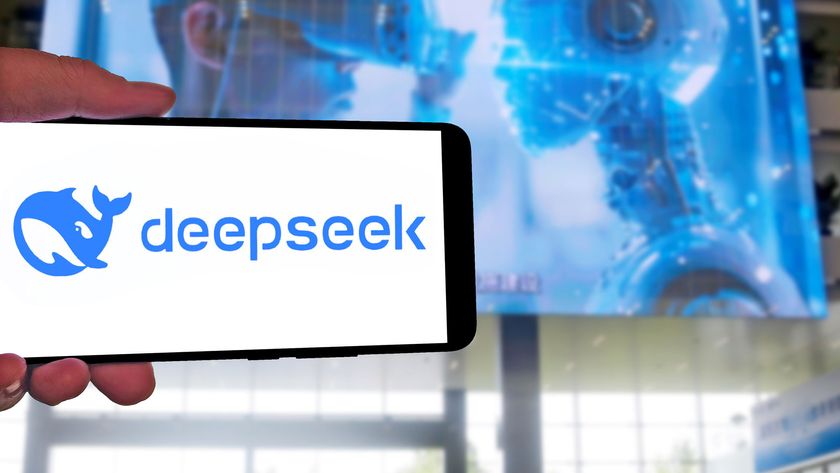
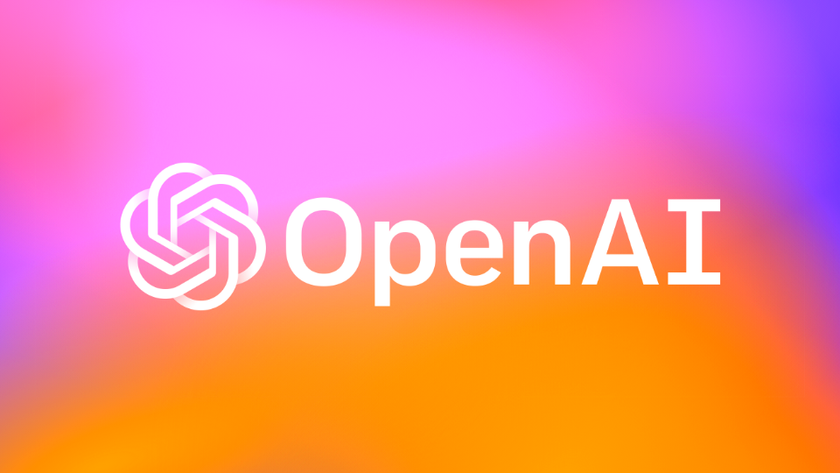
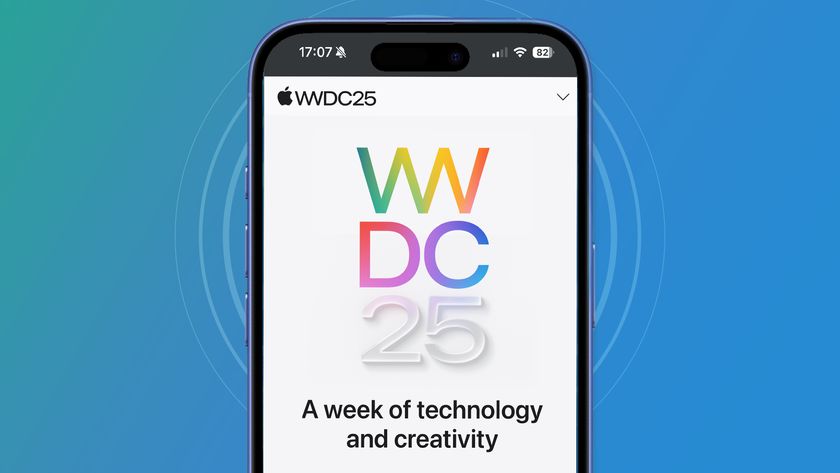
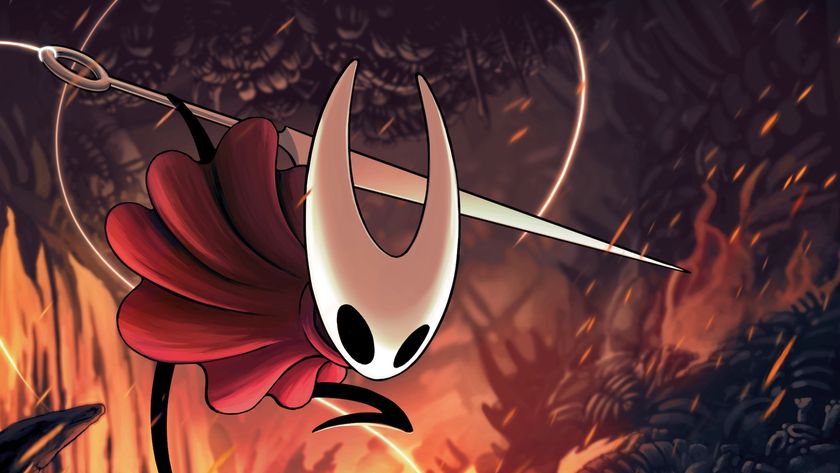
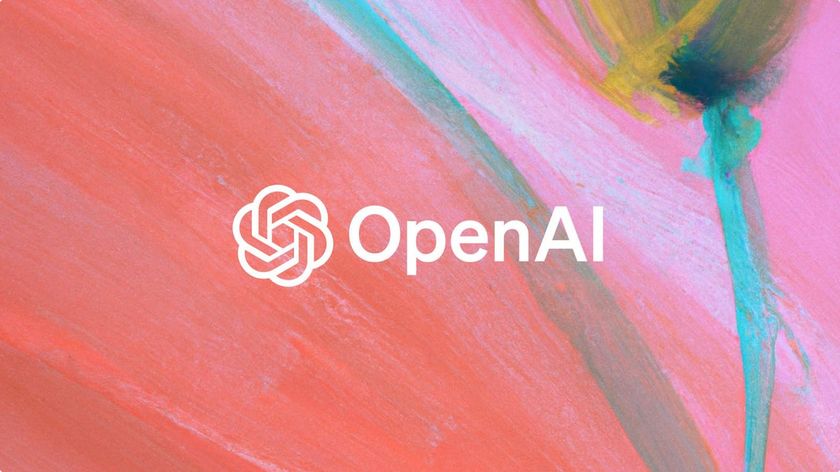
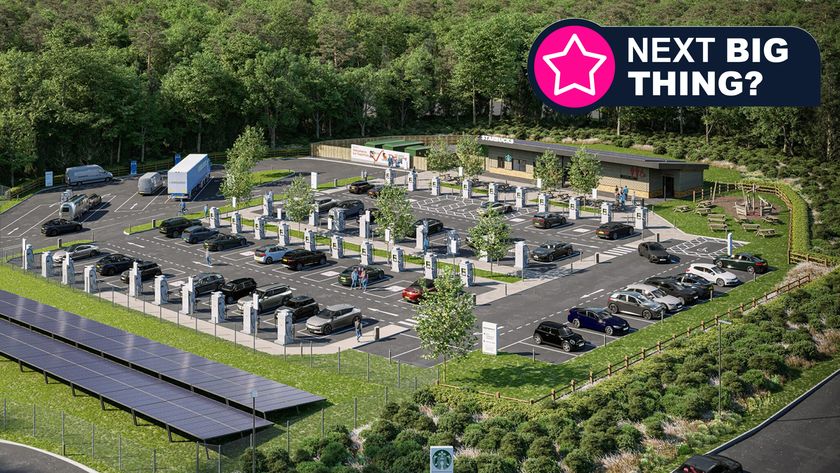