RAG: The key to unlocking your enterprises' knowledge vault
Leveraging Retrieval Augmented Generation (RAG)
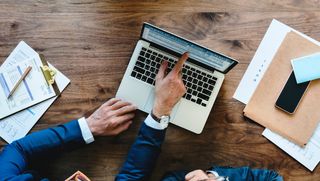
Imagine a world where every employee has access to the collective knowledge of your entire organization. When employees have powerful business insights at their fingertips, enterprises increase productivity, decrease costs and reap financial rewards. But the repository of valuable decks, spreadsheets, PDFs and other business collateral holds no collective insights without the ability to search and synthesize that information.
We’ve all heard if something sounds too good to be true, it probably is, so here’s the catch. Large Language Models (LLMs) are trained on publicly accessible data. However, most enterprises secure their data behind firewalls. This limitation can result in incorrect or misleading responses, known as hallucinations, which can adversely affect business decisions and growth.
Co-founder of Quantiphi.
Understanding Retrieval Augmented Generation (RAG)
In the most basic sense, RAG refines the responses of generative AI models through Knowledge Grounding, which makes generative AI results more precise. It also ensures the generated responses are “grounded” and connects the LLMs to the appropriate external sources, like databases, documents and webpages for contextually accurate responses. Paired with enterprise-specific data and robust retrieval mechanisms, we can mitigate hallucinations and produce contextually relevant, and helpful responses.
Knowledge grounding
Knowledge grounding guides generative language models to produce responses incorporating explicitly referenced information from a curated knowledge repository. Unlike training models with annotated data, knowledge grounding focuses on using existing information to influence real-time AI outputs.
In enterprises, knowledge grounding is essential because we want to provide enterprise-specific contextual information that the LLM might not have had access to before, thereby reducing hallucinations, which mostly occur due to lack of relevant information Grounded AI systems can adapt rapidly to changes in context, allowing enterprises to scale AI deployments across various departments and use cases easily. By integrating industry-specific and enterprise-specific knowledge into AI systems, organizations ensure that AI solutions are more relevant and useful in their particular domain, significantly enhancing the precision and reliability of AI-generated content.
The key to enterprise success
Enterprise-grade RAG-based offerings, like baioniq and others, empower businesses to harness the full potential of their data to inform business decisions. By automating workflows and enhancing knowledge worker productivity, RAG-based platforms drive enterprise efficiency gains while maintaining factualness. Additional benefits of leveraging RAG-based generative AI solutions for enterprises include:
1. Enhanced content generation - RAG’s contextual enrichment ensures LLM-generated responses are accurate and personalized, enhancing the enterprise’s business operations from the ground up. For example, a marketing team could query its customer preferences, market trends and even competitor strategies. Because the system can retrieve data from sources like market reports and surveys, it enables businesses to work in new, smarter ways and helps ensure the generated content reflects the many dynamics that make marketing content relevant and effective.
Are you a pro? Subscribe to our newsletter
Sign up to the TechRadar Pro newsletter to get all the top news, opinion, features and guidance your business needs to succeed!
2. Increased trust and reliability - RAG significantly enhances trust and reliability in enterprises by grounding AI-generated responses in verified, organization-specific information. By relying on real-time information from curated knowledge bases, RAG ensures that outputs are aligned with company policies, industry regulations and the latest internal data, which reduces the risk of outdated or incorrect information being used in decision-making processes.
Additionally, RAG provides transparency by allowing users to trace the sources of information, increasing confidence in the AI system's responses. This combination of accuracy, consistency, and traceability fosters greater trust in AI systems, leading to more reliable operations and improved customer experience. In a customer support role, by using RAG-powered LLM-based platforms, which pull from enriched, pre-vetted content, a customer service representative could easily pull from multiple data sources to provide contextually accurate responses, including real-time citations, elevating the overall customer experience through comprehensive responses that build trust.
3. Enhanced productivity - RAG-based systems leverage the power of LLMs with access to up-to-date, organization-specific information. This approach allows employees to quickly retrieve accurate, contextually relevant data from vast corporate knowledge bases, reducing time spent searching for information. By integrating seamlessly with existing workflows, RAG enables more informed decision-making, faster problem-solving, and improved team collaboration. This ultimately leads to increased efficiency, reduced errors and better utilization of institutional knowledge, driving overall productivity gains in the enterprise.
Limitations of RAG
While RAG is highly beneficial, like anything, it also has its limitations:
The quality of RAG's output is heavily dependent on the accuracy, completeness, and relevance of the underlying knowledge base. If this database is outdated, biased, or contains errors, RAG will propagate these and could cause an organizational lack of trust in AI systems.
The Retrieval component in the RAG systems may sometimes retrieve irrelevant information, leading to less focused or even incorrect responses. The process of indexing and maintaining large volumes of enterprise data can be challenging and expensive. Along the same lines, the retrieval process, upon such massive indexes, can be computationally expensive and time-consuming, potentially impacting real-time performance and end-user experience.
For example, during the recent presidential debate, OpenAI's ChatGPT and Microsoft's Copilot propagated a false AI-generated claim on social media indicating a broadcast delay would give CNN time to edit the broadcast thereby affecting the debate outcome between President Joe Biden and former President Donald Trump.
Despite news sources' prompt refutes, the AI chatbots continued to assert the “delay,” citing bogus online sources. Meanwhile, constituents panicked and news organizations scrambled to debunk the claims. Now imagine if this was your organization. If misinformation was propagated through AI and then leveraged company-wide, the resulting fallout could, at the very least, create a PR crisis requiring large-scale damage control and at worst, impact revenue. This real-life, presidential debate mishap shines a light on the inherent risks we face when leveraging AI from unfiltered data sources without appropriate checks and balances and it doubles down on the importance of effectively employing RAG in AI for enterprises.
Future direction and potential
Implementing RAG requires careful engineering and iterative refinement. Enterprises should experiment with different techniques for querying, information mapping, retrieval and synthesis to determine the best approach for their respective needs. With RAG's ability to understand and generate human-like text, facilitate automation and make decisions across diverse applications, industries like Healthcare and Life Sciences, Banking and Financial Services, Legal, Education, Media and Publishing and Marketing and Advertising will see the most significant impact from RAG.
As AI technologies continue to advance, RAG systems will likely become more sophisticated, incorporating improved retrieval algorithms and better integration with multi-modal data sources. This could enable enterprises to leverage not just text, but also images, audio and video in their knowledge bases, enabling them to make even more informed decisions with greater confidence.
With RAG, productivity increases, which can result in operational efficiency. This efficiency translates into streamlined workflows, faster decision-making processes and enhanced productivity across various functions. As businesses leverage RAG to generate contextually accurate insights and recommendations they also foster a culture of innovation and agility. Ultimately, RAG empowers organizations to operate more competitively in their respective industries by maximizing efficiency gains and maintaining lean operational structures.
We've featured the best business intelligence platform.
This article was produced as part of TechRadarPro's Expert Insights channel where we feature the best and brightest minds in the technology industry today. The views expressed here are those of the author and are not necessarily those of TechRadarPro or Future plc. If you are interested in contributing find out more here: https://www.techradar.com/news/submit-your-story-to-techradar-pro
Asif Hasan is Co-founder of Quantiphi, is a visionary leader with more than two decades of experience in technology services, healthcare and financial services industries.