Rapid AI development is putting security and privacy at risk
Pumping out AI systems increases the possibility of vulnerabilities, NIST warns
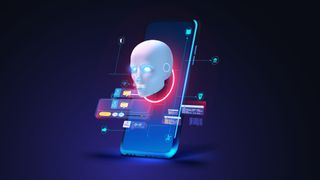
Rapid development of artificial intelligence is subject to a number of threats including misdirection, data poisoning and privacy attacks according to the National Institute of Standards and Technology (NIST).
A report from NIST states that hostile actors can attack and confuse AI systems and there is no way to fully protect against it.
The publication is intended to promote the responsible development of AI tools and help industries recognize that all AI can be subject to attacks, so greater care should be taken in deploying AI.
Evade, poison, abuse
Due to the massive data sets used to train large language models (LLM), it is not possible to fully audit all of the data being fed to an AI to train it, leaving vulnerabilities in the accuracy of the data, its content, or how it will respond to certain queries.
AI can be targeted during its training in an attack known as poisoning, which involves the AI recognizing obscene language as a common part of communication by throwing in swear words and toxic language into training material. In the past, AI trained on poisoned data have quickly become racist and derogatory in their responses to certain questions
There are also concerns that evasion attacks could target AI post-deployment by changing its recognition of inputs, or how an AI responds to an input. One example given in the publication is adding additional markings to a stop sign at an intersection, causing a self-driving car to not recognize the sign, potentially causing an accident.
The publication also highlights that the sources used to train AI can be identified by reverse engineering its responses to queries, and then adding malicious examples or information to these sources prompting inappropriate responses from the AI.
Are you a pro? Subscribe to our newsletter
Sign up to the TechRadar Pro newsletter to get all the top news, opinion, features and guidance your business needs to succeed!
Finally, it is possible for malicious actors to compromise a legitimate source of information used by the AI and edit its contents to change the AI’s behavior so that it no longer works within the context of its intended use.
The most worrying part of these attacks, the publication notes, is that these attacks can be done with “black-box” knowledge. Black-box implies that the attackers require very little knowledge of AI systems in order to carry out a successful attack. White-box would imply full knowledge of a system, and a partial knowledge is known as gray-box.
One of the authors of the publication, NIST computer scientist Apostol Vassilev, said, “We are providing an overview of attack techniques and methodologies that consider all types of AI systems.
“We also describe current mitigation strategies reported in the literature, but these available defenses currently lack robust assurances that they fully mitigate the risks. We are encouraging the community to come up with better defenses.”
More from TechRadar Pro
- Your next work PC will almost certainly be AI-equipped
- Have a look at our reviews of the best AI writer tools
- How AI is creating a retail revolution
Benedict has been writing about security issues for over 7 years, first focusing on geopolitics and international relations while at the University of Buckingham. During this time he studied BA Politics with Journalism, for which he received a second-class honours (upper division), then continuing his studies at a postgraduate level, achieving a distinction in MA Security, Intelligence and Diplomacy. Upon joining TechRadar Pro as a Staff Writer, Benedict transitioned his focus towards cybersecurity, exploring state-sponsored threat actors, malware, social engineering, and national security. Benedict is also an expert on B2B security products, including firewalls, antivirus, endpoint security, and password management.