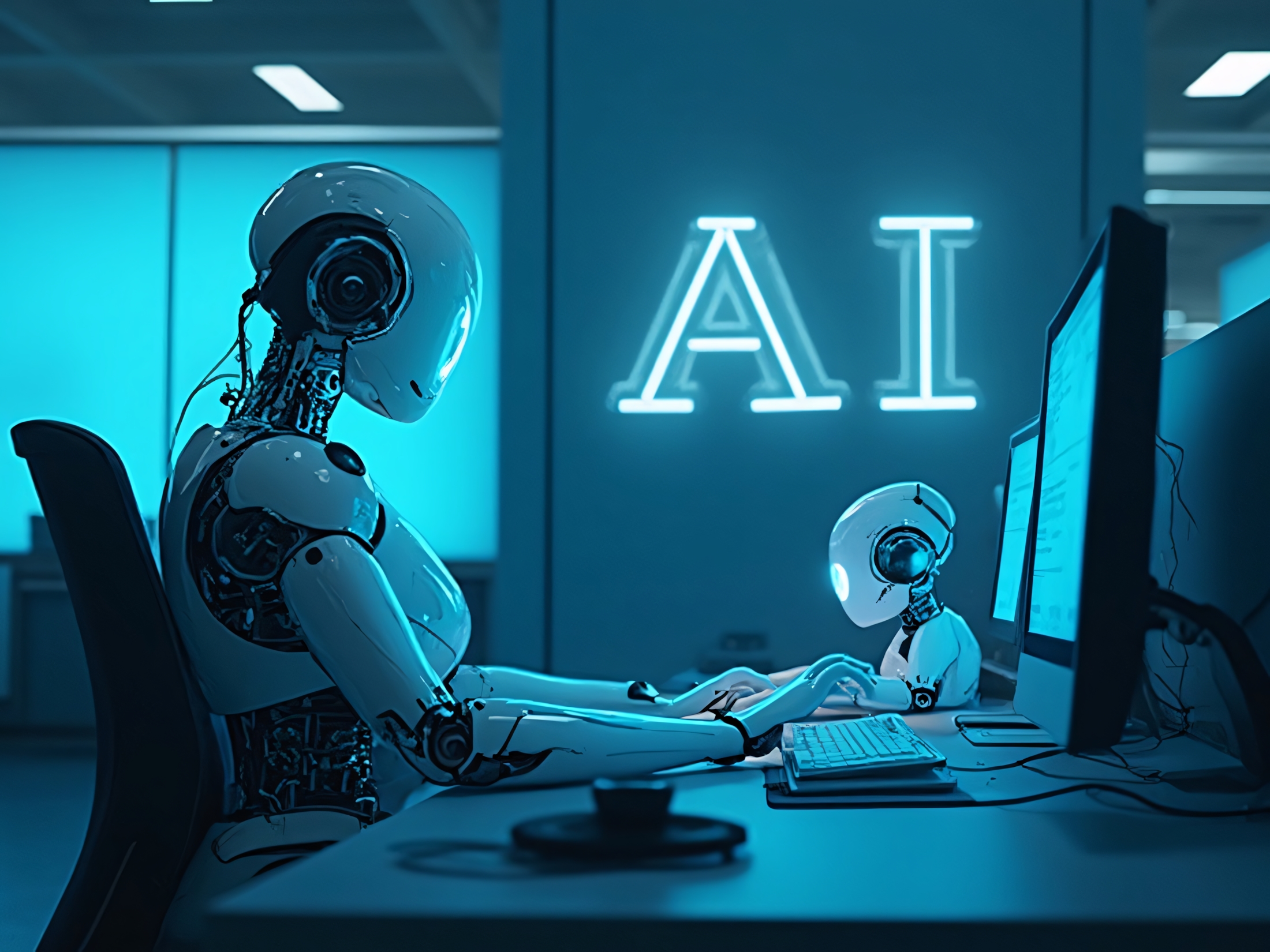
When OpenAI made headlines with its ChatGPT launch in 2022, it was clear that its evolution into the workforce was only a matter of time. According to Sam Altman, technology is advancing so rapidly that we can expect this shift within the next few months. But is replacing humans with AI employees a genius business move, or akin to hiring unpredictable interns?
While human employees come with additional costs such as training, sick leave, and salaries, AI workers bypass these expenses. Instead, they introduce their own unique challenges that can be just as disruptive for employers.
Founder and CTO of Enate.
Holidays vs. System Updates
Virtual workers don’t need to rest in the same way as humans, and it’s often seen as the biggest benefit of AI workers. AI doesn’t sleep and can work at all hours, unlike human employees who have set hours and need regular breaks. However, while AI never has the need for out of office replies, it does require plenty of maintenance and updates to perform.
For virtual employees, system downtime is the AI equivalent of a holiday, while planned maintenance or unexpected outages can disrupt operations just like a human taking time off for appointments or illness. The promise of 24/7 availability isn't entirely true if there are issues in the infrastructure.
According to a recent report by Splunk, unplanned downtime costs the world's largest 2,000 companies a combined $400 billion per year, averaging $200 million per company. From lost revenue, regulatory fines, and hidden costs like slower time to market and worsened brand reputations, downtime can be a very costly challenge.
So, although AI doesn't need a time off to recharge, ensuring its performing as it should needs to be a top priority for any organization introducing AI employees. AI models need to be regularly updated to avoid deteriorating in accuracy, leading to outdated or incorrect outputs. The recent shift towards retrieval-augmented generation (RAG) is an attempt to combat this, by enabling Large Language Models (LLMs) to source data from verified external sources, but it’s not an easy fix and still requires close monitoring.
Sickness vs Hallucinations
An AI employee won’t call in sick, but they can suffer from bugs and hallucinations that can be equally as disruptive. An AI hallucination occurs when a model generates incorrect or nonsense outputs, and this happens more than you would think. For instance, in 2017, Microsoft's AI chatbot Tay had to be shut down after it began generating offensive tweets less than a day after its launch.
Just like human immunity, different AI models vary in their robustness against bugs. OpenAI, Anthropic, and Google are all racing to reduce model hallucinations, but even top of the range models can still be unreliable at times. For organizations with AI employees, the priority should be treating these issues but also working to detect any potential future issues before they cause harm. Ongoing governance is therefore essential, in the same way as managing employee performance to identify any challenges or skills shortages.
Monthly pay vs. Updates
It’s a common misconception that AI is a pay-once, use forever deal. The cost of deploying AI solutions isn't set by a wide-open market; it's influenced by major players like Sam Altman and Google’s CEO, Sundar Pichai. As AI models evolve, it’s likely that businesses may need to invest further into costly updates or building entirely new systems to keep up with competitors. This ongoing investment could be the same as providing pay raises or professional development for human employees.
For example, ChatGPT recently launched a business-tier solution, and companies are already integrating AI co-pilots into their workflows. These tools are often tied to evolving licensing fees, cloud computing costs, and retraining requirements which means the AI you implement today could be severely outdated in just two years, requiring additional investment to maintain the same level of efficiency.
Training vs. Data input
In the same way that human employees need training to improve and develop in their role, LLMs need vast amounts of data to learn from to enhance performance. The more complex a model is, the more data it requires. For example, some models may need at least 10 million labelled items. It’s not just about the volume of data; the quality and relevance of this data are also crucial, as AI can only be as good as the data it is trained on. Poor data input means poor data output.
Linking abstract knowledge to real-world examples, also known as “Grounding AI” is a way to improve the ability of AI models to produce better predictions and responses. To do this, many companies are turning to RAG as a way of enhancing AI accuracy, by allowing models to pull from verified external sources instead of relying purely on pre-trained data.
Just like human development, training AI needs to be done regularly and consistently to get the best results. Especially as regulations around AI tighten and change, it’s crucial for companies to remain compliant with data protection laws, bias mitigation requirements, and industry-specific standards. Especially for businesses in highly regulated industries such as finance, firms using AI must align with regulations like the EU AI Act, ensuring models are transparent and accountable. This means regular audits, governance, and retraining.
So, what’s the verdict?
The truth is, it's not about choosing whether AI or human employees are better for a business. Instead, the real power comes by harnessing both. Issues such as governance, training, hallucinations and updates can be just as time consuming and expensive for businesses as managing human talent. The value of AI is in enabling humans to take more time for the things that only they can do; deeper thinking, creativity and building authentic human connections – capabilities that AI simply cannot, no matter how much it continues to evolve and advance.
Granted, implementing AI tools into business processes will free up time and improve efficiency, but that doesn’t mean that humans don’t still have a place. When the efficiency and intelligence of AI join forces with the creativity, empathy, and strategic thinking of humans, the possibilities are limitless. Together, they can drive innovation, boost productivity, and propel businesses towards long-term sustainable success.
We list the best task management app.
This article was produced as part of TechRadarPro's Expert Insights channel where we feature the best and brightest minds in the technology industry today. The views expressed here are those of the author and are not necessarily those of TechRadarPro or Future plc. If you are interested in contributing find out more here: https://www.techradar.com/news/submit-your-story-to-techradar-pro
Are you a pro? Subscribe to our newsletter
Sign up to the TechRadar Pro newsletter to get all the top news, opinion, features and guidance your business needs to succeed!
Founder and CTO of Enate.
You must confirm your public display name before commenting
Please logout and then login again, you will then be prompted to enter your display name.