Why it's important to A/B test your AI-powered website
A/B testing is a crucial part of the website build process
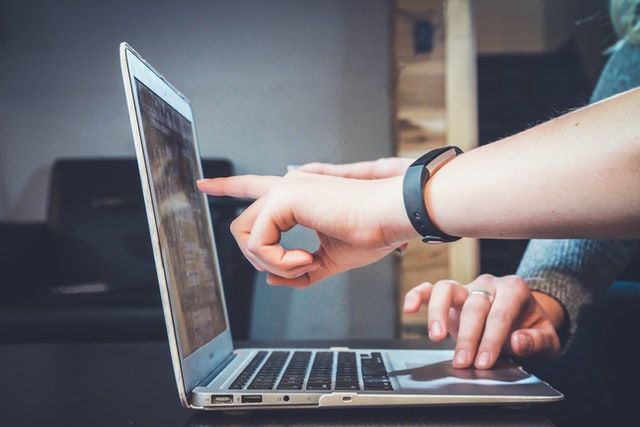
The best AI website builders can help you generate a fully-functioning website faster than ever before. But blindly implementing AI suggestions without a thorough quality assurance analysis and A/B test can hurt your business.
AI suggestions can reinforce existing biases within the algorithm, introduce errors that cause an erosion of customer trust, and even risk compliance issues with local regulations if you aren’t careful enough.
Let’s explore how A/B testing enables marketers to confidently harness AI's potential to create hyper-optimized and high-converting websites, without any of the risk. We’ll share some key benefits, offer strategic advice, and show what A/B testing for AI websites looks like in action.
Common pitfalls of AI website generation
While AI can streamline website creation, relying solely on an external algorithm without human oversight can lead to several issues. Here are a few common pitfalls of AI website builders that you should watch out for:
- Biased outputs: AI models trained on biased data may generate website content that reinforces stereotypes or excludes certain demographics.
- Factual inaccuracies: AI can sometimes generate incorrect information or misinterpret context, leading to errors in website copy.
- Lack of originality: AI-generated content may lack the unique perspective and creativity that human writers bring, resulting in generic or repetitive websites.
- Compliance risks: AI may not fully grasp legal and regulatory requirements, such as accessibility guidelines or data privacy laws, putting your website at risk of non-compliance.
- Poor user experience: Without testing, AI-generated websites may have confusing navigation, slow load times, or unappealing designs that frustrate users and increase bounce rates.
How an A/B test provides quality assurance for AI
A/B testing is a powerful tool for validating the effectiveness of AI-generated websites. By comparing different versions of web pages, you can determine which AI outputs resonate best with your target audience. A/B testing helps identify potential biases, inaccuracies, or usability issues in AI-created content before it's fully deployed.
Through data-driven experimentation, you can optimize models to generate higher-quality websites that drive key metrics like conversion rates and engagement. A/B testing also ensures your AI-powered site meets crucial compliance standards. With a robust A/B testing process, you can leverage AI's efficiency while maintaining the integrity and performance of your website. A few ways A/B testing can help:
- Data-driven optimization: A/B tests provide concrete data on how AI features impact user behavior, enabling informed optimization decisions.
- Improved user experience: By validating AI suggestions with real users, you can create a website that better meets their needs and preferences.
- Increased conversions: Identifying the best-performing AI elements through A/B testing can directly boost conversion rates and revenue.
- Reduced risk: A/B testing catches potential issues before they fully deploy, protecting your brand reputation and mitigating legal risks.
- Continuous improvement: Iterative A/B testing allows you to refine AI models over time based on evolving user data and expectations.
Tips for getting started with A/B testing
By embracing A/B testing as an essential tool in your AI website development process, you can harness the power of artificial intelligence while maintaining the quality, integrity, and performance of your site. To effectively A/B test your AI-powered website, consider implementing these best practices:
Are you a pro? Subscribe to our newsletter
Sign up to the TechRadar Pro newsletter to get all the top news, opinion, features and guidance your business needs to succeed!
1. Define clear objectives
Start by identifying what you want to achieve with your A/B testing. This could be increasing click-through rates, improving conversion rates, reducing bounce rates, or any other specific goal that aligns with your business objectives. Having a clear objective helps guide the design and implementation of the A/B test.
When defining your objectives, make sure they are SMART: Specific, Measurable, Actionable, Relevant, and Time-bound. For example, instead of a vague goal like "improve user experience", aim for something more concrete like "increase newsletter sign-ups by 10% in the next quarter". This provides a clear target to work towards and measure success against.
Communicate your objectives clearly to all stakeholders involved in the A/B testing process. Use simple language and visuals to ensure everyone understands the purpose and expected outcomes of the test. Getting buy-in and alignment upfront will make the entire process smoother.
2. Select the right metrics
Choose key performance indicators (KPIs) to measure the success of your test. This could include metrics like conversion rates, bounce rates, click-through rates, average order value, or any other metric that aligns with your objectives.
Focus on metrics that directly impact your business goals, like revenue per user or customer acquisition cost, rather than vanity metrics like page views that don't provide true insight. As the saying goes, "measure what matters".
Make sure the metrics you choose are relevant to your specific test and will provide actionable insights. Avoid tracking too many metrics which can muddy the results. Identify a primary metric that will be the key indicator of your test's performance.
Set up proper tracking in an analytics tool so you can monitor your chosen metrics throughout the test. Verify the data is being captured accurately before launching the test.
3. Segment your audience
Develop a hypothesis for each element you plan to test. A hypothesis is a prediction you create prior to running an experiment. It states clearly what is being changed, what you believe the outcome will be, and why.
A good hypothesis is specific and testable. Use a format like: If [variable], then [result], because [rationale]. For example: "If we reduce the number of form fields, form completions will increase, because it reduces friction in the signup process."
Grounding hypotheses in research and data will make them stronger. Do your homework first by analyzing existing site data, collecting user feedback, and reviewing competitor sites. Insights from these activities can inform your hypotheses.
Prioritize and limit the number of hypotheses. Focus on those you believe will have the greatest impact. Spreading yourself too thin by testing too many things at once will limit your ability to find meaningful results. Aim for quality over quantity.
4. Test one variable at a time
For conclusive results, only test one element at a time. If you change multiple variables simultaneously, you won't be able to determine which one was responsible for any change in performance.
For example, if you modify a headline and a button color at the same time and see an increase in conversions, you won't know which element (or combination of the two) actually caused the lift. You'd have to run follow-up tests to isolate the effect of each change.
By testing just one thing in each variation, like only the headline or only the button color, you can confidently attribute any difference in results to the specific variable being tested. This allows you to identify exactly what works and what doesn't.
5. Analyze and implement learnings
Once your test has run for the planned duration and reached the desired sample size, it's time to analyze the results. If you've set everything up properly, this should be as simple as comparing the performance of your key metrics for each variation in your testing tool.
Focus on your primary metric, but look at the data as a whole. Be aware of any potential external factors that could have skewed the results, like holidays, special promotions, or technical issues. Segment your results by user properties like traffic source, device, or location to uncover deeper insights.
Determine whether your results are statistically significant using an A/B significance test. Doing so will tell you the probability that your results are real and not due to chance. Most A/B testing tools have this feature built in, or there are plenty of free options online.
If a variation is statistically significant and aligns with your hypothesis, congratulations! You can confidently implement the change knowing it's an improvement. If not, don't fret. A negative result is still valuable learning. It's just as important to know what doesn't work as what does.
Ritoban Mukherjee is a tech and innovations journalist from West Bengal, India. These days, most of his work revolves around B2B software, such as AI website builders, VoIP platforms, and CRMs, among other things. He has also been published on Tom's Guide, Creative Bloq, IT Pro, Gizmodo, Quartz, and Mental Floss.
- Owain WilliamsB2B Editor, Website Builders & CRM