Your essential guide to Digital Twins: from basics to benefits - Part 1
Guide to digital twins: Basics, uses, benefits
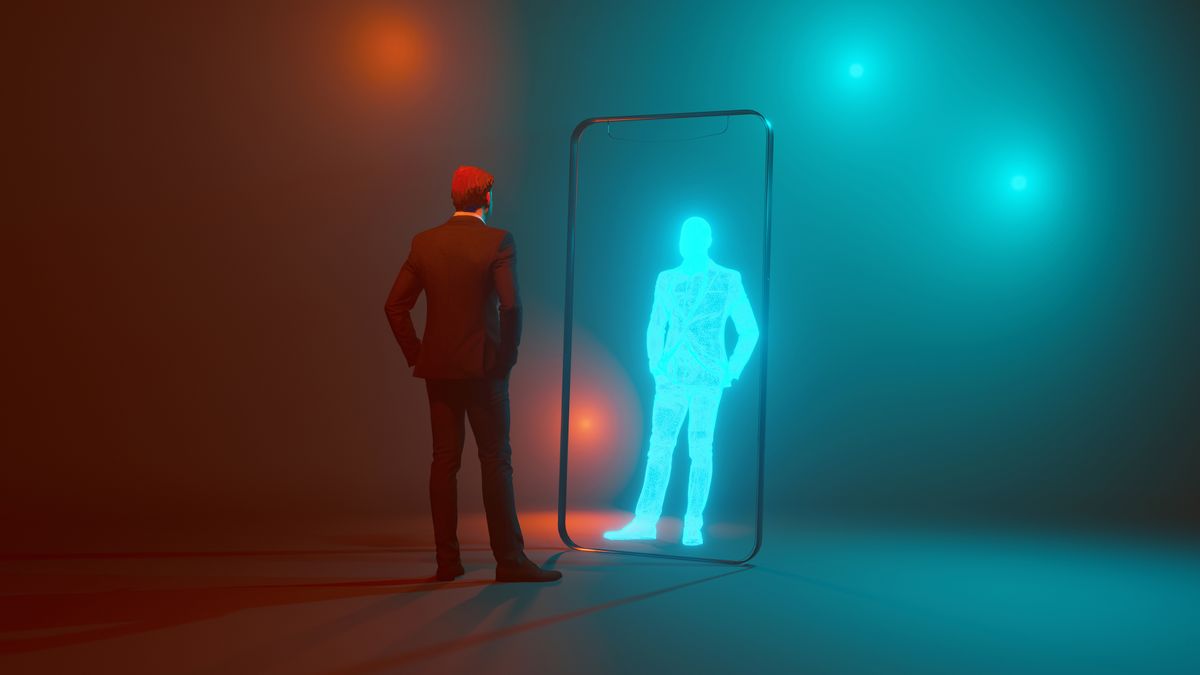
In today's rapidly advancing technological landscape, digital transformation is a key driver of innovation across many industries. One of the most impactful technologies leading this revolution is the digital twin. This is a real-time virtual model that replicates a physical object, system, or process. It continuously receives data from its physical twin, creating a dynamic, up-to-date digital replica. This allows users to monitor, simulate, and enhance the object or system without directly interacting with the real-world version.
While the concept of a digital twin isn’t entirely new, its application has exploded due to progress in Internet of Things (IoT) devices, artificial intelligence (AI), machine learning, and big data. Across industries such as manufacturing, healthcare, smart cities, and aerospace, digital twins are helping businesses operate more efficiently, reduce costs, and improve decision-making.
This comprehensive guide explains what digital twins are, how they are used in different industries, and how they are built, while also examining their potential impact on future technology.
Product Manager for Professional Visualization at PNY Technologies EMEA.
What is a Digital Twin?
A digital twin is a digital replica of a physical object or system that is kept in sync with its real-world counterpart. This virtual model collects real-time data through sensors, cameras, and IoT devices, providing an accurate representation of the object’s current state. A digital twin is more than a 3D model—it is a dynamic, data-driven simulation that evolves as the physical object changes over time.
One of the key features of a digital twin is its ability to simulate future scenarios. By using historical and real-time data, digital twins can model various conditions and outcomes, allowing businesses to foresee challenges, predict system failures, and optimize operations. These simulations are incredibly useful for making informed decisions without physically testing every possible outcome, saving both time and money.
The origins and evolution of Digital Twins
The idea of creating digital replicas of physical objects goes back to the early days of space exploration. NASA engineers used physical models and simulators to monitor and diagnose issues with spacecraft that were too far away to inspect directly. These early models laid the groundwork for the development of the digital twin concept.
But it wasn’t until the convergence of IoT, big data, and AI technologies that digital twins became a practical tool for mainstream use. Today, digital twins are more sophisticated than ever before. They can process vast amounts of data in real time, enabling detailed simulations and advanced analytics. As digital twins continue to evolve, they are playing a pivotal role in the Fourth Industrial Revolution (Industry 4.0), transforming sectors from manufacturing to urban planning.
What are Digital Twins used for?
Digital twins are incredibly versatile and have found applications across many industries. Below are some of the primary use cases for these tools and how they are transforming different sectors.
Manufacturing
Manufacturing is one of the largest users of digital twin technology. In this sector, digital twins are used to optimize production lines, monitor machinery, and improve product designs. By creating digital replicas of factory equipment and processes, manufacturers can simulate different production scenarios, spot inefficiencies, and predict potential breakdowns. For example, a car manufacturer might create a digital twin of an assembly line to test how adding a new robotic arm will affect workflow. By running simulations, the manufacturer can fine-tune the process to ensure the robotic arm integrates seamlessly, leading to more efficient working.
Additionally, digital twins are invaluable for predictive maintenance. By collecting data on the condition of machines—such as temperature, vibration, or pressure—they can predict when a machine is likely to break down. This allows manufacturers to schedule maintenance at the right time, cutting unplanned downtime and extending the lifespan of equipment.
Healthcare
Digital twin technology is making strides in healthcare, where it is used to model individual patients, medical devices, and biological systems. Personalized healthcare, in particular, benefits from digital twins. By creating a virtual model of a patient’s body or organ, doctors can simulate different treatment options to determine the best course of action.
For example, heart surgeons may use a digital twin of a patient’s heart to plan and simulate a procedure before performing it. This allows them to visualize the surgery and plan for potential complications, improving the chances of successful surgery.
Similarly, pharmaceutical companies use digital twins to simulate how drugs interact with the human body. This helps them develop new treatments more quickly and efficiently by testing drug reactions virtually before conducting human trials.
Medical device manufacturers also leverage digital twins to design and test products like pacemakers, joint replacements, or diagnostic machines. By using digital twins, they can ensure that devices perform optimally within the body before they are ever implanted or used.
Smart cities and urban planning
Digital twins are now playing an increasingly important role in the development of smart cities. City planners and local authorities are using digital twins to create virtual models of urban infrastructure and services, such as transport systems, energy grids, and waste management. With real-time data collected from sensors placed throughout an urban area, digital twins can help cities monitor traffic flow, energy usage, and pollution. This data allows town planners to test different strategies for improving transport networks, reducing congestion, and lowering energy consumption.
For example, local government could use a digital twin of its public transport system to simulate the impact of rerouting buses or adding new train lines. By running these models, planners can identify the best ways to cut travel times and improve service without disrupting the real-world network.
Digital twins are also instrumental in disaster response planning. By modelling how a city would be affected by natural disasters such as floods, earthquakes, or fires, emergency workers can develop better contingency plans and improve their ability to manage crises in real time.
Aerospace
In the aerospace industry, digital twins are widely used to improve aircraft design, production, and maintenance. By creating digital replicas of airplanes, engines, and other components, aerospace engineers can simulate how different factors—such as extreme weather, air pressure, or mechanical stress—will affect an aircraft over time.
One of key aspects of digital twins in aerospace is their ability to inform predictive maintenance. For example, digital twins of jet engines collect data on performance metrics like temperature, pressure, and vibration. Using this data, engineers can predict when parts are likely to wear out or malfunction, allowing airlines to perform maintenance before a problem occurs. This reduces the risk of in-flight issues and lowers operational costs. Airlines are also using digital twins to simulate flight conditions and boost fuel efficiency. By modelling various flight paths, weather conditions, and aircraft configurations, digital twins help pilots and airlines reduce fuel consumption, leading to both cost savings and environmental benefits.
Energy
Digital twins have found significant applications in the energy sector, where they are used to monitor and enhance the performance of power plants, wind farms, and solar energy systems. By creating digital replicas of these systems, operators can simulate different conditions—such as changes in weather or energy demand—and finetune operations accordingly. For example, wind farm operators use digital twins to track the performance of individual turbines. The digital twin collects data on wind speed, turbine rotation, and power output, providing insights into each turbine’s efficiency. This data helps operators identify underperforming turbines and adjust to maximize energy production.
In the case of power plants, digital twins can monitor critical elements, such as generators, cooling systems, and pipelines. By predicting when components are likely to fail, digital twins enable operators to carry out preventive maintenance, cutting downtime and improving the reliability of the energy grid.
Digital twins also help energy companies manage grid stability. With real-time data on energy consumption and generation, they allow operators to balance supply and demand more efficiently, preventing blackouts and reducing energy waste.
How to build a Digital Twin
Creating a digital twin involves several steps, from data collection to simulation and analysis. Below is a detailed explanation of each phase in the development process.
Data collection
The first step in building a digital twin is collecting data from the physical object or system. This is typically gathered using sensors, IoT devices, and control systems that measure key parameters such as temperature, pressure, speed, and vibration. In some cases, historical data may also be used to model how the object has performed over time.
For example, if you are creating a digital twin of a factory production line, you would install sensors on the machines to track their performance, energy consumption, and maintenance needs. The more data is collected, the more accurate and detailed the digital twin will be.
Create the digital model
Once the data is collected, the next step is to create a digital model of the object or system. This is often built using 3D modelling software or computer-aided design (CAD) tools. The complexity of the model will depend on the nature of the object being reproduced. For some applications, a simple 3D model might suffice, while for others, a highly detailed, physics-based simulation may be needed.
For instance, a digital twin of a wind turbine would not only include a 3D model of the turbine blades but also a simulation of how the blades interact with different wind speeds and environmental conditions.
Real-time data connection
To keep the digital twin updated, it must be connected to its physical twin through real-time data transmission. This connection ensures that the digital version evolves as the physical object changes or moves through different operating conditions.
For example, in smart cities, sensors placed throughout an urban area feed data back to the digital twin, which continuously updates itself in line with real-time conditions like traffic flow, air quality, or energy consumption.
In many cases, real-time data is transmitted via IoT platforms and processed in the cloud. Advanced AI and machine learning algorithms are often used to analyze this data, providing insights into how the physical object is performing and predicting future outcomes.
Simulation and optimization
Once the digital twin is live and connected to its physical equivalent, it can be used for simulations and optimizations. By testing different scenarios and variables on the digital model, users can identify areas which can be finetuned without affecting the real-world object. For instance, a digital twin of a factory machine could help simulate how different workloads or production speeds impact overall efficiency. Based on the findings of these models, factory managers can adjust operations to reduce bottlenecks, save energy, or cut downtime.
Digital twins can also be used for scenario planning, helping organisations to model how changes—such as new laws or market conditions—will impact their operations. By running these simulations, businesses can prepare for potential challenges and make more informed strategic decisions.
Continuous updates and maintenance
To remain accurate, digital twins must be constantly updated with real-time data and information about the physical object’s condition. This includes tracking wear and tear, repairs, and upgrades. Regular updates ensure that the digital twin remains a reliable tool for monitoring and simulation.
In the aerospace industry, digital twins of aircraft engines are regularly updated to reflect the engine’s current condition and usage history. These updates allow engineers to make accurate predictions about future maintenance needs and performance.
Understanding Digital Twins vs. traditional simulations
At the core, a digital twin is a digital replica of a physical object, system, or process, continuously updated with real-time data. Unlike traditional simulations, which run under set parameters and aren’t connected to the real world, digital twins are dynamic. They reflect the current state of their physical counterpart, using data gathered from sensors and other sources. This connection allows digital twins to provide accurate insights, predict future behaviors, and make real-time decisions.
For example, a simulation might help design a new product, testing it virtually under various conditions. However, once the simulation ends, it’s static. A digital twin, on the other hand, remains active, continuously mirroring the physical product’s lifecycle. This ongoing connection enables businesses to manage assets, troubleshoot issues, and optimize operations more effectively than a traditional simulation could.
Building a Digital Twin: timeframes and considerations
The timeline to create a digital twin depends heavily on the complexity of the object or system being modeled. For a simple asset, like a single machine or piece of equipment, a digital twin could be developed in a few weeks to a few months. The process involves setting up data collection through sensors, building the digital model, and validating its accuracy. For more complex systems—like an entire manufacturing plant or a smart city—development can take from 6 months to a year, or even longer. These large-scale digital twins require extensive data integration, advanced simulations, and rigorous testing to ensure they mirror their physical counterparts accurately.
Creating a digital twin isn’t just about initial setup; it’s an ongoing effort. A digital twin must be updated and maintained to keep it in sync with the physical world. This involves continuous data collection, periodic calibration, and refining the model to reflect any changes in the real-world object.
We've featured the best data visualization tool.
This article was produced as part of TechRadarPro's Expert Insights channel where we feature the best and brightest minds in the technology industry today. The views expressed here are those of the author and are not necessarily those of TechRadarPro or Future plc. If you are interested in contributing find out more here: https://www.techradar.com/news/submit-your-story-to-techradar-pro
Are you a pro? Subscribe to our newsletter
Sign up to the TechRadar Pro newsletter to get all the top news, opinion, features and guidance your business needs to succeed!
Frank Scheufens is Product Manager for Professional Visualization at PNY Technologies EMEA.
You must confirm your public display name before commenting
Please logout and then login again, you will then be prompted to enter your display name.
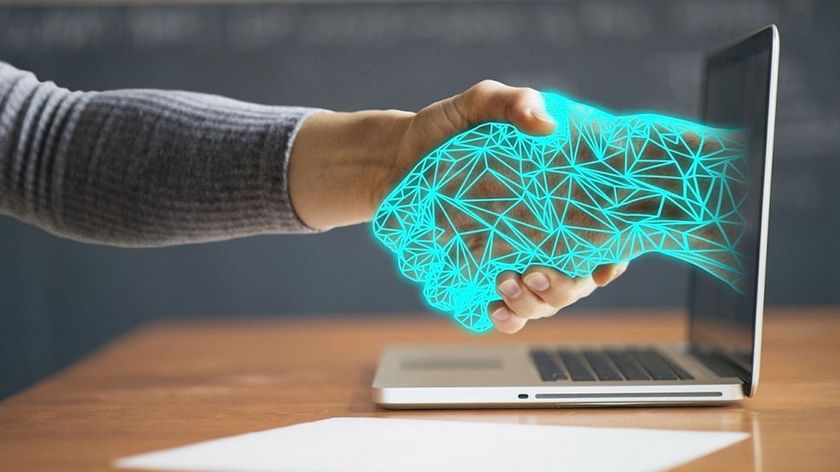
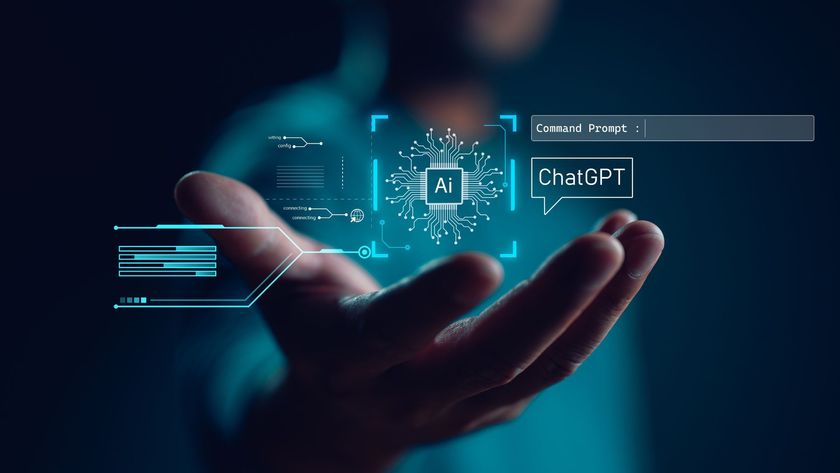
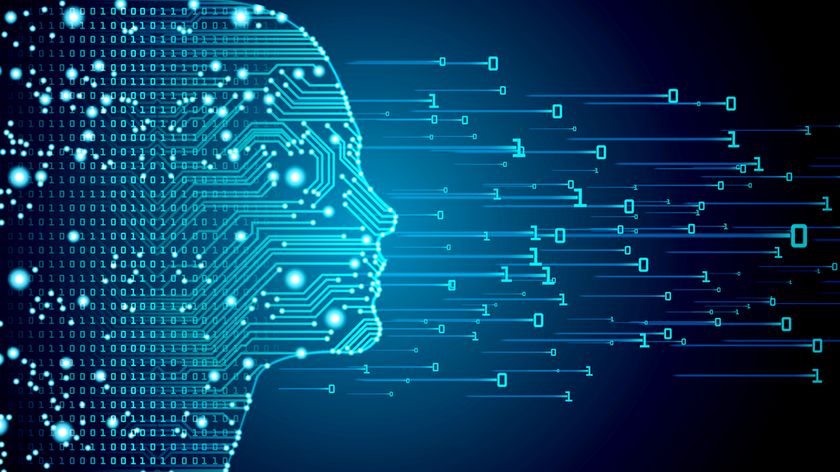
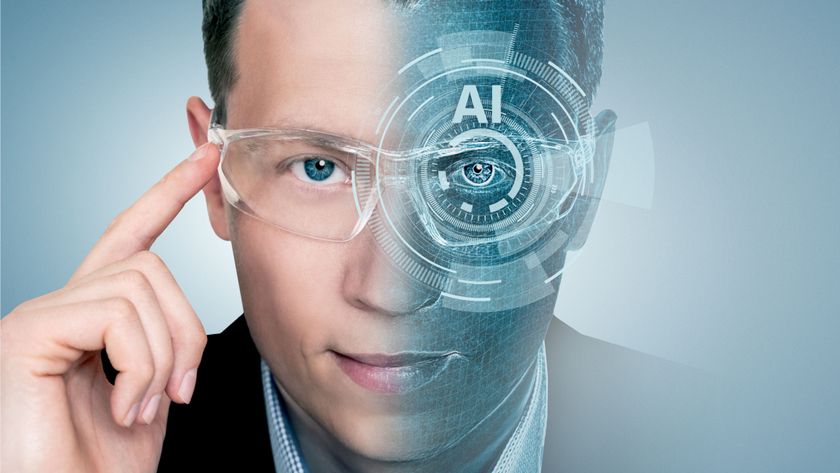
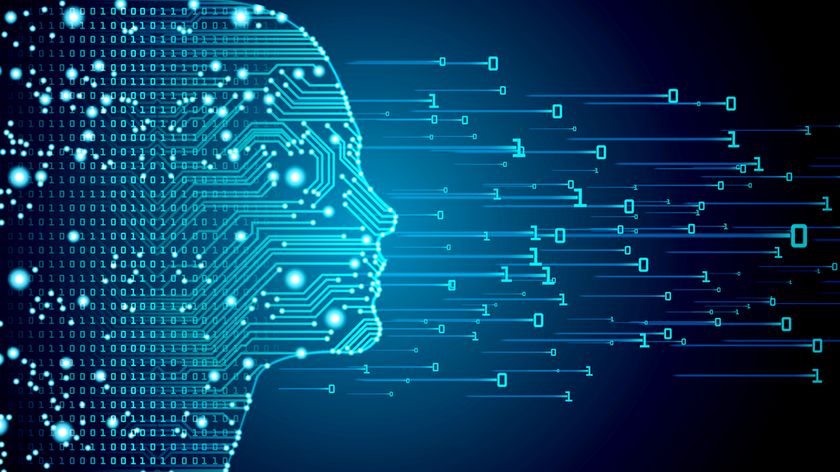
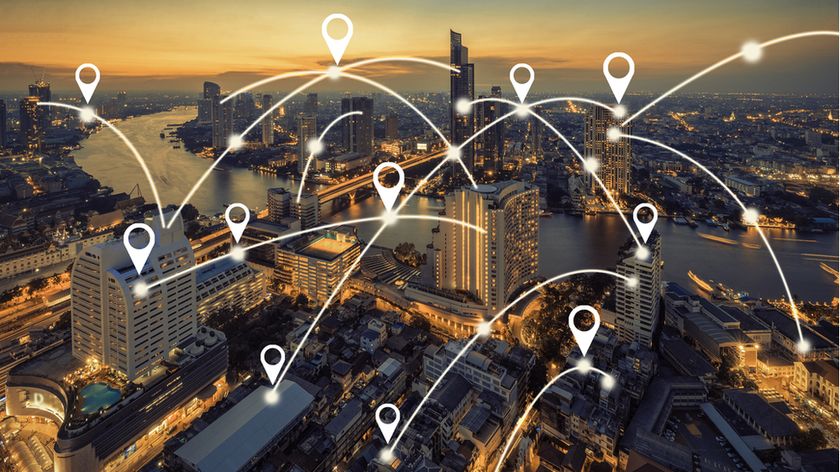
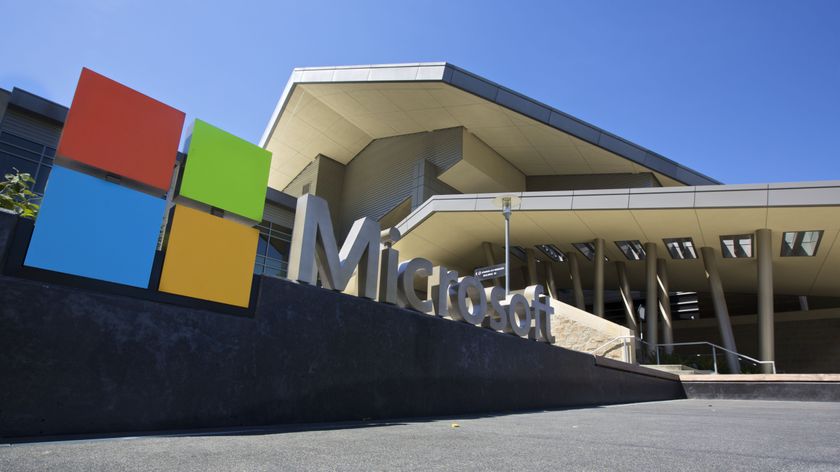
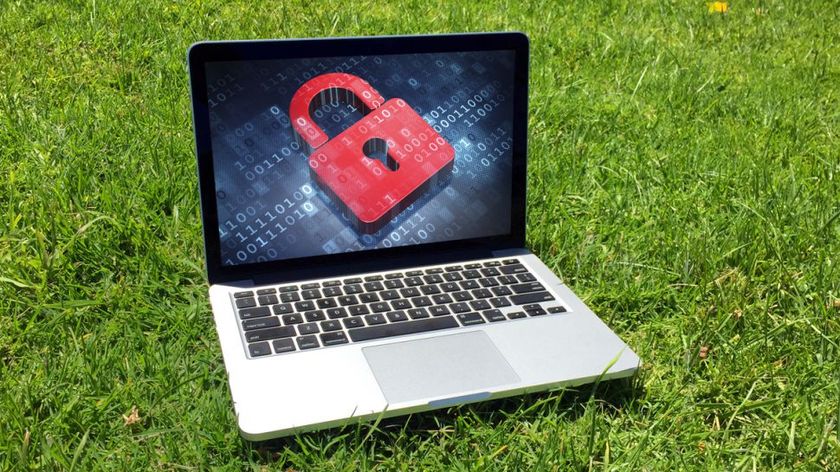
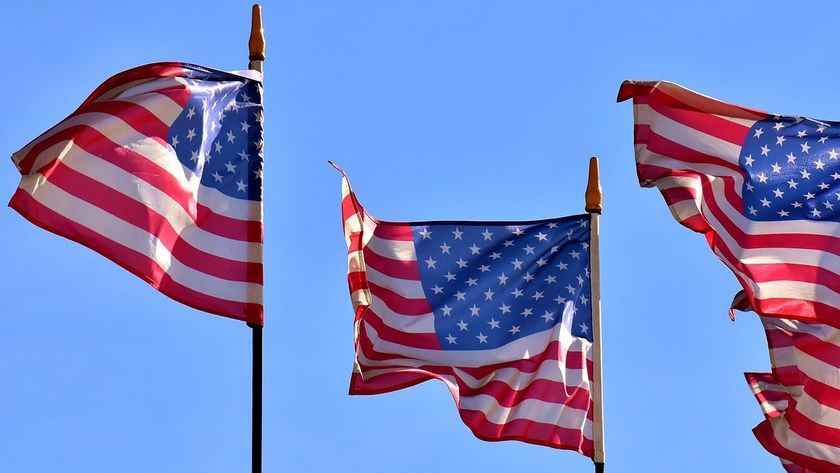
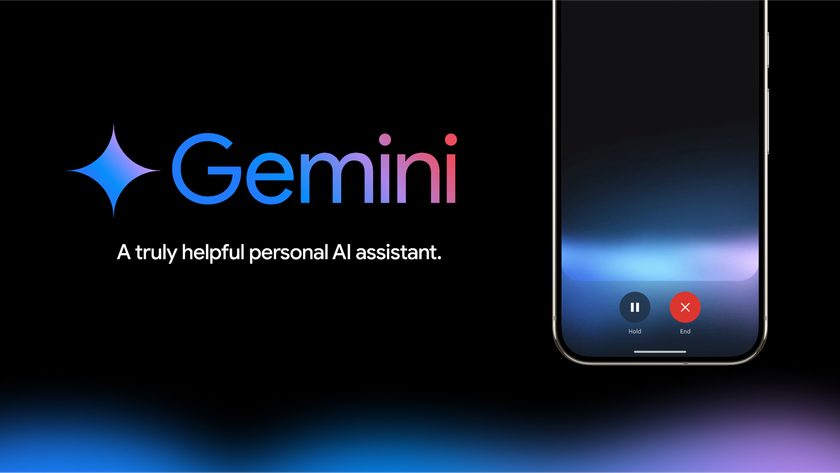
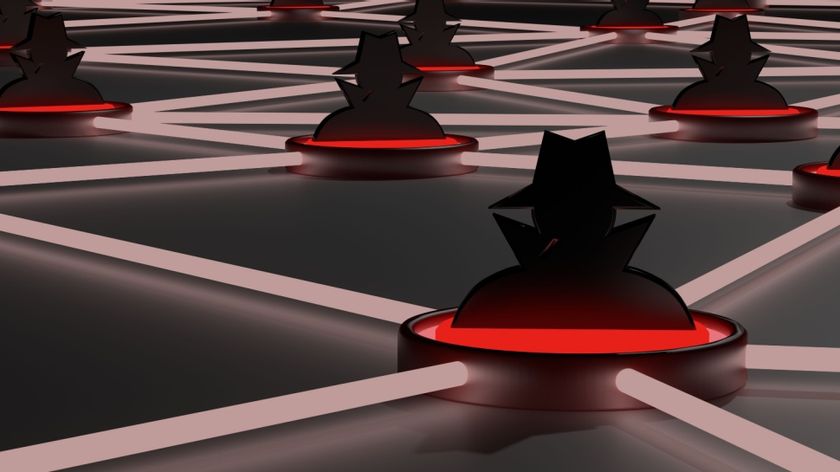
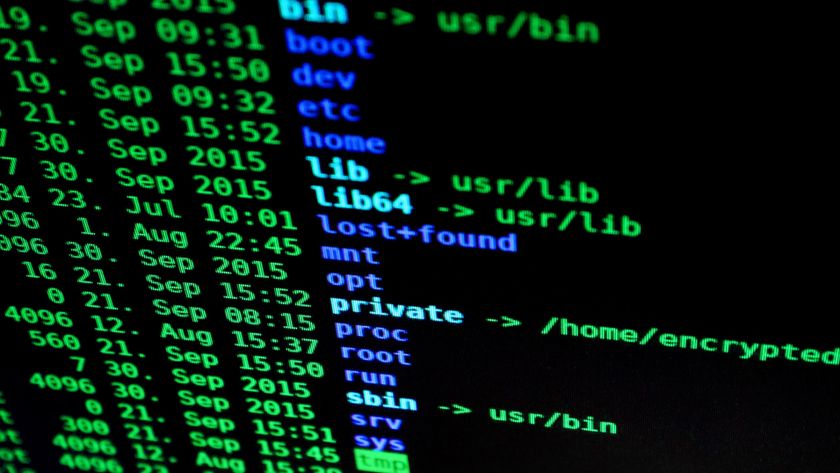
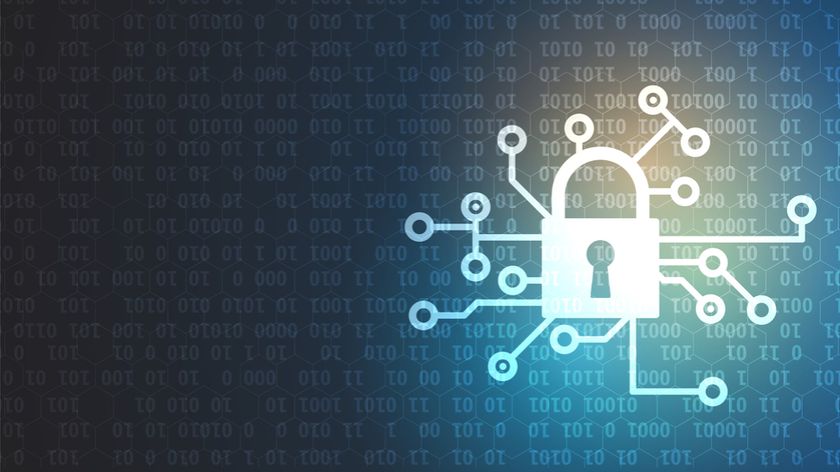
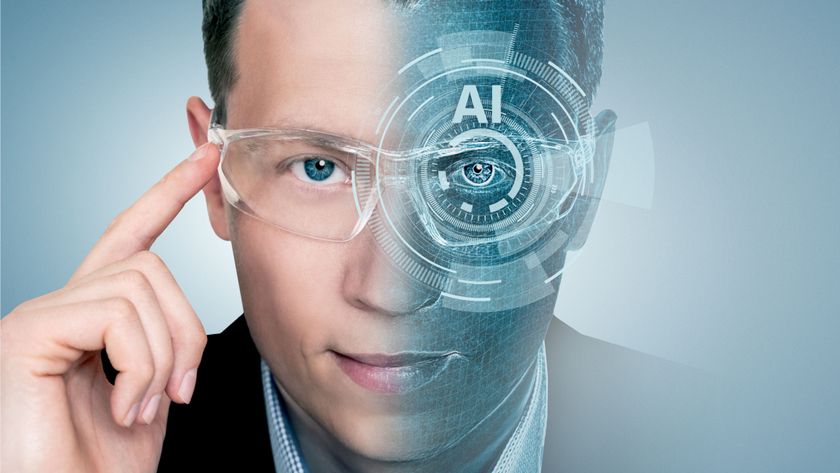
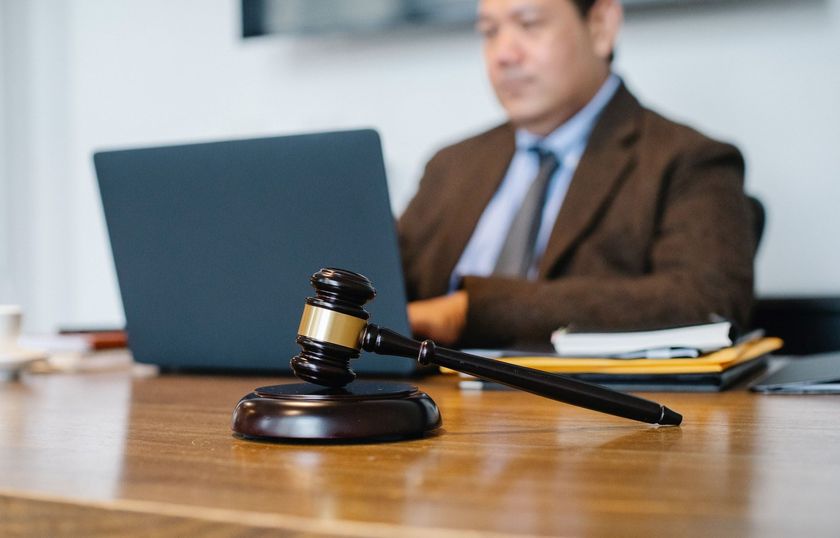
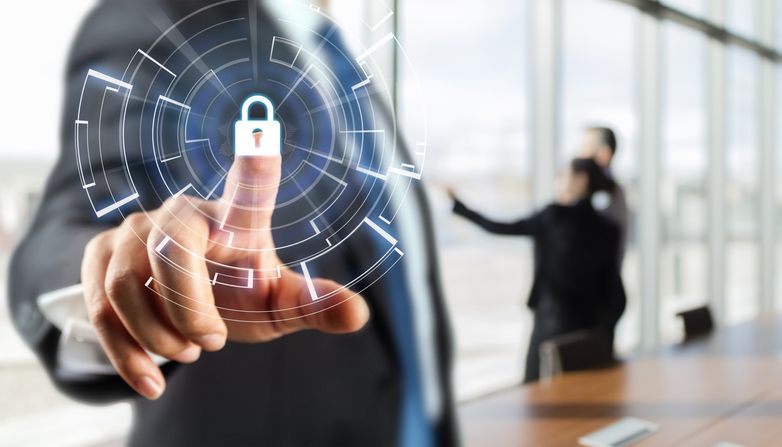
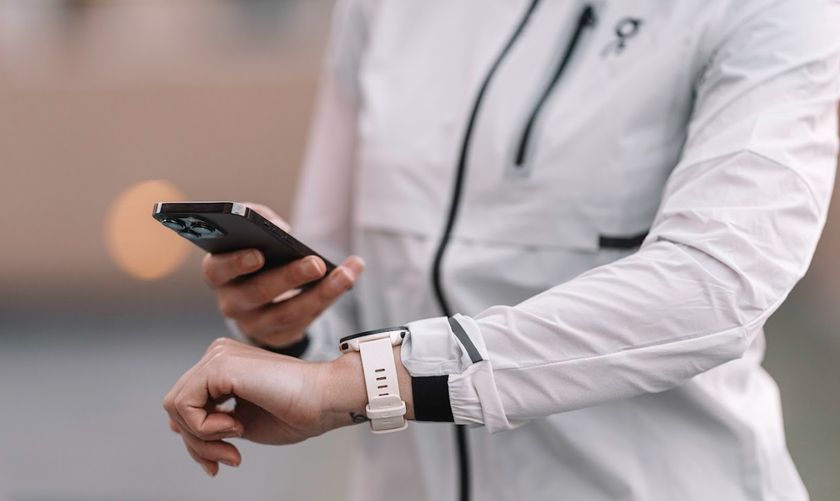
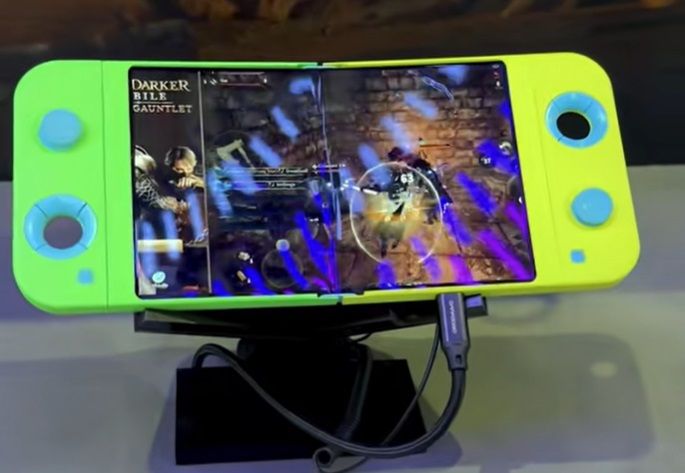